- Privacy Policy

Home » Research Paper – Structure, Examples and Writing Guide

Research Paper – Structure, Examples and Writing Guide
Table of Contents

Research Paper
Definition:
Research Paper is a written document that presents the author’s original research, analysis, and interpretation of a specific topic or issue.
It is typically based on Empirical Evidence, and may involve qualitative or quantitative research methods, or a combination of both. The purpose of a research paper is to contribute new knowledge or insights to a particular field of study, and to demonstrate the author’s understanding of the existing literature and theories related to the topic.
Structure of Research Paper
The structure of a research paper typically follows a standard format, consisting of several sections that convey specific information about the research study. The following is a detailed explanation of the structure of a research paper:
The title page contains the title of the paper, the name(s) of the author(s), and the affiliation(s) of the author(s). It also includes the date of submission and possibly, the name of the journal or conference where the paper is to be published.
The abstract is a brief summary of the research paper, typically ranging from 100 to 250 words. It should include the research question, the methods used, the key findings, and the implications of the results. The abstract should be written in a concise and clear manner to allow readers to quickly grasp the essence of the research.
Introduction
The introduction section of a research paper provides background information about the research problem, the research question, and the research objectives. It also outlines the significance of the research, the research gap that it aims to fill, and the approach taken to address the research question. Finally, the introduction section ends with a clear statement of the research hypothesis or research question.
Literature Review
The literature review section of a research paper provides an overview of the existing literature on the topic of study. It includes a critical analysis and synthesis of the literature, highlighting the key concepts, themes, and debates. The literature review should also demonstrate the research gap and how the current study seeks to address it.
The methods section of a research paper describes the research design, the sample selection, the data collection and analysis procedures, and the statistical methods used to analyze the data. This section should provide sufficient detail for other researchers to replicate the study.
The results section presents the findings of the research, using tables, graphs, and figures to illustrate the data. The findings should be presented in a clear and concise manner, with reference to the research question and hypothesis.
The discussion section of a research paper interprets the findings and discusses their implications for the research question, the literature review, and the field of study. It should also address the limitations of the study and suggest future research directions.
The conclusion section summarizes the main findings of the study, restates the research question and hypothesis, and provides a final reflection on the significance of the research.
The references section provides a list of all the sources cited in the paper, following a specific citation style such as APA, MLA or Chicago.
How to Write Research Paper
You can write Research Paper by the following guide:
- Choose a Topic: The first step is to select a topic that interests you and is relevant to your field of study. Brainstorm ideas and narrow down to a research question that is specific and researchable.
- Conduct a Literature Review: The literature review helps you identify the gap in the existing research and provides a basis for your research question. It also helps you to develop a theoretical framework and research hypothesis.
- Develop a Thesis Statement : The thesis statement is the main argument of your research paper. It should be clear, concise and specific to your research question.
- Plan your Research: Develop a research plan that outlines the methods, data sources, and data analysis procedures. This will help you to collect and analyze data effectively.
- Collect and Analyze Data: Collect data using various methods such as surveys, interviews, observations, or experiments. Analyze data using statistical tools or other qualitative methods.
- Organize your Paper : Organize your paper into sections such as Introduction, Literature Review, Methods, Results, Discussion, and Conclusion. Ensure that each section is coherent and follows a logical flow.
- Write your Paper : Start by writing the introduction, followed by the literature review, methods, results, discussion, and conclusion. Ensure that your writing is clear, concise, and follows the required formatting and citation styles.
- Edit and Proofread your Paper: Review your paper for grammar and spelling errors, and ensure that it is well-structured and easy to read. Ask someone else to review your paper to get feedback and suggestions for improvement.
- Cite your Sources: Ensure that you properly cite all sources used in your research paper. This is essential for giving credit to the original authors and avoiding plagiarism.
Research Paper Example
Note : The below example research paper is for illustrative purposes only and is not an actual research paper. Actual research papers may have different structures, contents, and formats depending on the field of study, research question, data collection and analysis methods, and other factors. Students should always consult with their professors or supervisors for specific guidelines and expectations for their research papers.
Research Paper Example sample for Students:
Title: The Impact of Social Media on Mental Health among Young Adults
Abstract: This study aims to investigate the impact of social media use on the mental health of young adults. A literature review was conducted to examine the existing research on the topic. A survey was then administered to 200 university students to collect data on their social media use, mental health status, and perceived impact of social media on their mental health. The results showed that social media use is positively associated with depression, anxiety, and stress. The study also found that social comparison, cyberbullying, and FOMO (Fear of Missing Out) are significant predictors of mental health problems among young adults.
Introduction: Social media has become an integral part of modern life, particularly among young adults. While social media has many benefits, including increased communication and social connectivity, it has also been associated with negative outcomes, such as addiction, cyberbullying, and mental health problems. This study aims to investigate the impact of social media use on the mental health of young adults.
Literature Review: The literature review highlights the existing research on the impact of social media use on mental health. The review shows that social media use is associated with depression, anxiety, stress, and other mental health problems. The review also identifies the factors that contribute to the negative impact of social media, including social comparison, cyberbullying, and FOMO.
Methods : A survey was administered to 200 university students to collect data on their social media use, mental health status, and perceived impact of social media on their mental health. The survey included questions on social media use, mental health status (measured using the DASS-21), and perceived impact of social media on their mental health. Data were analyzed using descriptive statistics and regression analysis.
Results : The results showed that social media use is positively associated with depression, anxiety, and stress. The study also found that social comparison, cyberbullying, and FOMO are significant predictors of mental health problems among young adults.
Discussion : The study’s findings suggest that social media use has a negative impact on the mental health of young adults. The study highlights the need for interventions that address the factors contributing to the negative impact of social media, such as social comparison, cyberbullying, and FOMO.
Conclusion : In conclusion, social media use has a significant impact on the mental health of young adults. The study’s findings underscore the need for interventions that promote healthy social media use and address the negative outcomes associated with social media use. Future research can explore the effectiveness of interventions aimed at reducing the negative impact of social media on mental health. Additionally, longitudinal studies can investigate the long-term effects of social media use on mental health.
Limitations : The study has some limitations, including the use of self-report measures and a cross-sectional design. The use of self-report measures may result in biased responses, and a cross-sectional design limits the ability to establish causality.
Implications: The study’s findings have implications for mental health professionals, educators, and policymakers. Mental health professionals can use the findings to develop interventions that address the negative impact of social media use on mental health. Educators can incorporate social media literacy into their curriculum to promote healthy social media use among young adults. Policymakers can use the findings to develop policies that protect young adults from the negative outcomes associated with social media use.
References :
- Twenge, J. M., & Campbell, W. K. (2019). Associations between screen time and lower psychological well-being among children and adolescents: Evidence from a population-based study. Preventive medicine reports, 15, 100918.
- Primack, B. A., Shensa, A., Escobar-Viera, C. G., Barrett, E. L., Sidani, J. E., Colditz, J. B., … & James, A. E. (2017). Use of multiple social media platforms and symptoms of depression and anxiety: A nationally-representative study among US young adults. Computers in Human Behavior, 69, 1-9.
- Van der Meer, T. G., & Verhoeven, J. W. (2017). Social media and its impact on academic performance of students. Journal of Information Technology Education: Research, 16, 383-398.
Appendix : The survey used in this study is provided below.
Social Media and Mental Health Survey
- How often do you use social media per day?
- Less than 30 minutes
- 30 minutes to 1 hour
- 1 to 2 hours
- 2 to 4 hours
- More than 4 hours
- Which social media platforms do you use?
- Others (Please specify)
- How often do you experience the following on social media?
- Social comparison (comparing yourself to others)
- Cyberbullying
- Fear of Missing Out (FOMO)
- Have you ever experienced any of the following mental health problems in the past month?
- Do you think social media use has a positive or negative impact on your mental health?
- Very positive
- Somewhat positive
- Somewhat negative
- Very negative
- In your opinion, which factors contribute to the negative impact of social media on mental health?
- Social comparison
- In your opinion, what interventions could be effective in reducing the negative impact of social media on mental health?
- Education on healthy social media use
- Counseling for mental health problems caused by social media
- Social media detox programs
- Regulation of social media use
Thank you for your participation!
Applications of Research Paper
Research papers have several applications in various fields, including:
- Advancing knowledge: Research papers contribute to the advancement of knowledge by generating new insights, theories, and findings that can inform future research and practice. They help to answer important questions, clarify existing knowledge, and identify areas that require further investigation.
- Informing policy: Research papers can inform policy decisions by providing evidence-based recommendations for policymakers. They can help to identify gaps in current policies, evaluate the effectiveness of interventions, and inform the development of new policies and regulations.
- Improving practice: Research papers can improve practice by providing evidence-based guidance for professionals in various fields, including medicine, education, business, and psychology. They can inform the development of best practices, guidelines, and standards of care that can improve outcomes for individuals and organizations.
- Educating students : Research papers are often used as teaching tools in universities and colleges to educate students about research methods, data analysis, and academic writing. They help students to develop critical thinking skills, research skills, and communication skills that are essential for success in many careers.
- Fostering collaboration: Research papers can foster collaboration among researchers, practitioners, and policymakers by providing a platform for sharing knowledge and ideas. They can facilitate interdisciplinary collaborations and partnerships that can lead to innovative solutions to complex problems.
When to Write Research Paper
Research papers are typically written when a person has completed a research project or when they have conducted a study and have obtained data or findings that they want to share with the academic or professional community. Research papers are usually written in academic settings, such as universities, but they can also be written in professional settings, such as research organizations, government agencies, or private companies.
Here are some common situations where a person might need to write a research paper:
- For academic purposes: Students in universities and colleges are often required to write research papers as part of their coursework, particularly in the social sciences, natural sciences, and humanities. Writing research papers helps students to develop research skills, critical thinking skills, and academic writing skills.
- For publication: Researchers often write research papers to publish their findings in academic journals or to present their work at academic conferences. Publishing research papers is an important way to disseminate research findings to the academic community and to establish oneself as an expert in a particular field.
- To inform policy or practice : Researchers may write research papers to inform policy decisions or to improve practice in various fields. Research findings can be used to inform the development of policies, guidelines, and best practices that can improve outcomes for individuals and organizations.
- To share new insights or ideas: Researchers may write research papers to share new insights or ideas with the academic or professional community. They may present new theories, propose new research methods, or challenge existing paradigms in their field.
Purpose of Research Paper
The purpose of a research paper is to present the results of a study or investigation in a clear, concise, and structured manner. Research papers are written to communicate new knowledge, ideas, or findings to a specific audience, such as researchers, scholars, practitioners, or policymakers. The primary purposes of a research paper are:
- To contribute to the body of knowledge : Research papers aim to add new knowledge or insights to a particular field or discipline. They do this by reporting the results of empirical studies, reviewing and synthesizing existing literature, proposing new theories, or providing new perspectives on a topic.
- To inform or persuade: Research papers are written to inform or persuade the reader about a particular issue, topic, or phenomenon. They present evidence and arguments to support their claims and seek to persuade the reader of the validity of their findings or recommendations.
- To advance the field: Research papers seek to advance the field or discipline by identifying gaps in knowledge, proposing new research questions or approaches, or challenging existing assumptions or paradigms. They aim to contribute to ongoing debates and discussions within a field and to stimulate further research and inquiry.
- To demonstrate research skills: Research papers demonstrate the author’s research skills, including their ability to design and conduct a study, collect and analyze data, and interpret and communicate findings. They also demonstrate the author’s ability to critically evaluate existing literature, synthesize information from multiple sources, and write in a clear and structured manner.
Characteristics of Research Paper
Research papers have several characteristics that distinguish them from other forms of academic or professional writing. Here are some common characteristics of research papers:
- Evidence-based: Research papers are based on empirical evidence, which is collected through rigorous research methods such as experiments, surveys, observations, or interviews. They rely on objective data and facts to support their claims and conclusions.
- Structured and organized: Research papers have a clear and logical structure, with sections such as introduction, literature review, methods, results, discussion, and conclusion. They are organized in a way that helps the reader to follow the argument and understand the findings.
- Formal and objective: Research papers are written in a formal and objective tone, with an emphasis on clarity, precision, and accuracy. They avoid subjective language or personal opinions and instead rely on objective data and analysis to support their arguments.
- Citations and references: Research papers include citations and references to acknowledge the sources of information and ideas used in the paper. They use a specific citation style, such as APA, MLA, or Chicago, to ensure consistency and accuracy.
- Peer-reviewed: Research papers are often peer-reviewed, which means they are evaluated by other experts in the field before they are published. Peer-review ensures that the research is of high quality, meets ethical standards, and contributes to the advancement of knowledge in the field.
- Objective and unbiased: Research papers strive to be objective and unbiased in their presentation of the findings. They avoid personal biases or preconceptions and instead rely on the data and analysis to draw conclusions.
Advantages of Research Paper
Research papers have many advantages, both for the individual researcher and for the broader academic and professional community. Here are some advantages of research papers:
- Contribution to knowledge: Research papers contribute to the body of knowledge in a particular field or discipline. They add new information, insights, and perspectives to existing literature and help advance the understanding of a particular phenomenon or issue.
- Opportunity for intellectual growth: Research papers provide an opportunity for intellectual growth for the researcher. They require critical thinking, problem-solving, and creativity, which can help develop the researcher’s skills and knowledge.
- Career advancement: Research papers can help advance the researcher’s career by demonstrating their expertise and contributions to the field. They can also lead to new research opportunities, collaborations, and funding.
- Academic recognition: Research papers can lead to academic recognition in the form of awards, grants, or invitations to speak at conferences or events. They can also contribute to the researcher’s reputation and standing in the field.
- Impact on policy and practice: Research papers can have a significant impact on policy and practice. They can inform policy decisions, guide practice, and lead to changes in laws, regulations, or procedures.
- Advancement of society: Research papers can contribute to the advancement of society by addressing important issues, identifying solutions to problems, and promoting social justice and equality.
Limitations of Research Paper
Research papers also have some limitations that should be considered when interpreting their findings or implications. Here are some common limitations of research papers:
- Limited generalizability: Research findings may not be generalizable to other populations, settings, or contexts. Studies often use specific samples or conditions that may not reflect the broader population or real-world situations.
- Potential for bias : Research papers may be biased due to factors such as sample selection, measurement errors, or researcher biases. It is important to evaluate the quality of the research design and methods used to ensure that the findings are valid and reliable.
- Ethical concerns: Research papers may raise ethical concerns, such as the use of vulnerable populations or invasive procedures. Researchers must adhere to ethical guidelines and obtain informed consent from participants to ensure that the research is conducted in a responsible and respectful manner.
- Limitations of methodology: Research papers may be limited by the methodology used to collect and analyze data. For example, certain research methods may not capture the complexity or nuance of a particular phenomenon, or may not be appropriate for certain research questions.
- Publication bias: Research papers may be subject to publication bias, where positive or significant findings are more likely to be published than negative or non-significant findings. This can skew the overall findings of a particular area of research.
- Time and resource constraints: Research papers may be limited by time and resource constraints, which can affect the quality and scope of the research. Researchers may not have access to certain data or resources, or may be unable to conduct long-term studies due to practical limitations.
About the author
Muhammad Hassan
Researcher, Academic Writer, Web developer
You may also like

Research Objectives – Types, Examples and...

Limitations in Research – Types, Examples and...

Future Research – Thesis Guide

Data Verification – Process, Types and Examples

APA Table of Contents – Format and Example

APA Research Paper Format – Example, Sample and...

How To Write A Research Paper
Step-By-Step Tutorial With Examples + FREE Template
By: Derek Jansen (MBA) | Expert Reviewer: Dr Eunice Rautenbach | March 2024
For many students, crafting a strong research paper from scratch can feel like a daunting task – and rightly so! In this post, we’ll unpack what a research paper is, what it needs to do , and how to write one – in three easy steps. 🙂
Overview: Writing A Research Paper
What (exactly) is a research paper.
- How to write a research paper
- Stage 1 : Topic & literature search
- Stage 2 : Structure & outline
- Stage 3 : Iterative writing
- Key takeaways
Let’s start by asking the most important question, “ What is a research paper? ”.
Simply put, a research paper is a scholarly written work where the writer (that’s you!) answers a specific question (this is called a research question ) through evidence-based arguments . Evidence-based is the keyword here. In other words, a research paper is different from an essay or other writing assignments that draw from the writer’s personal opinions or experiences. With a research paper, it’s all about building your arguments based on evidence (we’ll talk more about that evidence a little later).
Now, it’s worth noting that there are many different types of research papers , including analytical papers (the type I just described), argumentative papers, and interpretative papers. Here, we’ll focus on analytical papers , as these are some of the most common – but if you’re keen to learn about other types of research papers, be sure to check out the rest of the blog .
With that basic foundation laid, let’s get down to business and look at how to write a research paper .
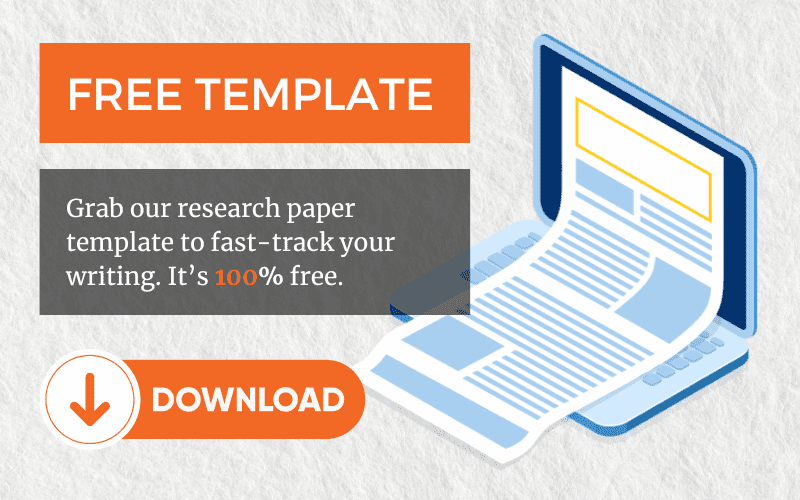
Overview: The 3-Stage Process
While there are, of course, many potential approaches you can take to write a research paper, there are typically three stages to the writing process. So, in this tutorial, we’ll present a straightforward three-step process that we use when working with students at Grad Coach.
These three steps are:
- Finding a research topic and reviewing the existing literature
- Developing a provisional structure and outline for your paper, and
- Writing up your initial draft and then refining it iteratively
Let’s dig into each of these.
Need a helping hand?
Step 1: Find a topic and review the literature
As we mentioned earlier, in a research paper, you, as the researcher, will try to answer a question . More specifically, that’s called a research question , and it sets the direction of your entire paper. What’s important to understand though is that you’ll need to answer that research question with the help of high-quality sources – for example, journal articles, government reports, case studies, and so on. We’ll circle back to this in a minute.
The first stage of the research process is deciding on what your research question will be and then reviewing the existing literature (in other words, past studies and papers) to see what they say about that specific research question. In some cases, your professor may provide you with a predetermined research question (or set of questions). However, in many cases, you’ll need to find your own research question within a certain topic area.
Finding a strong research question hinges on identifying a meaningful research gap – in other words, an area that’s lacking in existing research. There’s a lot to unpack here, so if you wanna learn more, check out the plain-language explainer video below.
Once you’ve figured out which question (or questions) you’ll attempt to answer in your research paper, you’ll need to do a deep dive into the existing literature – this is called a “ literature search ”. Again, there are many ways to go about this, but your most likely starting point will be Google Scholar .
If you’re new to Google Scholar, think of it as Google for the academic world. You can start by simply entering a few different keywords that are relevant to your research question and it will then present a host of articles for you to review. What you want to pay close attention to here is the number of citations for each paper – the more citations a paper has, the more credible it is (generally speaking – there are some exceptions, of course).
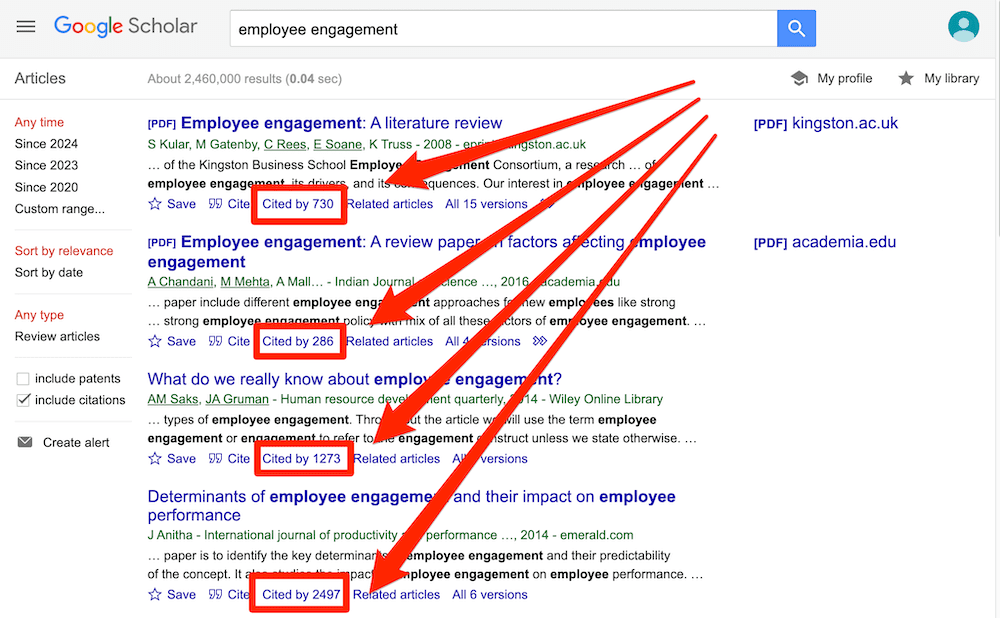
Ideally, what you’re looking for are well-cited papers that are highly relevant to your topic. That said, keep in mind that citations are a cumulative metric , so older papers will often have more citations than newer papers – just because they’ve been around for longer. So, don’t fixate on this metric in isolation – relevance and recency are also very important.
Beyond Google Scholar, you’ll also definitely want to check out academic databases and aggregators such as Science Direct, PubMed, JStor and so on. These will often overlap with the results that you find in Google Scholar, but they can also reveal some hidden gems – so, be sure to check them out.
Once you’ve worked your way through all the literature, you’ll want to catalogue all this information in some sort of spreadsheet so that you can easily recall who said what, when and within what context. If you’d like, we’ve got a free literature spreadsheet that helps you do exactly that.
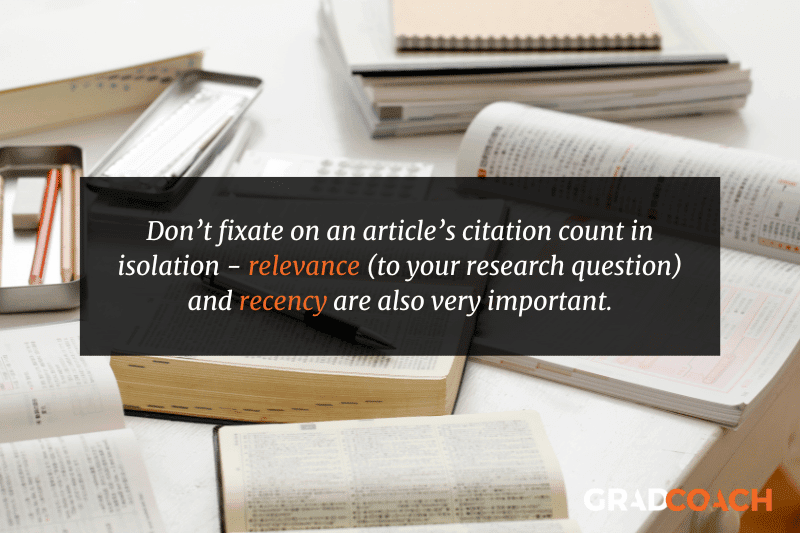
Step 2: Develop a structure and outline
With your research question pinned down and your literature digested and catalogued, it’s time to move on to planning your actual research paper .
It might sound obvious, but it’s really important to have some sort of rough outline in place before you start writing your paper. So often, we see students eagerly rushing into the writing phase, only to land up with a disjointed research paper that rambles on in multiple
Now, the secret here is to not get caught up in the fine details . Realistically, all you need at this stage is a bullet-point list that describes (in broad strokes) what you’ll discuss and in what order. It’s also useful to remember that you’re not glued to this outline – in all likelihood, you’ll chop and change some sections once you start writing, and that’s perfectly okay. What’s important is that you have some sort of roadmap in place from the start.
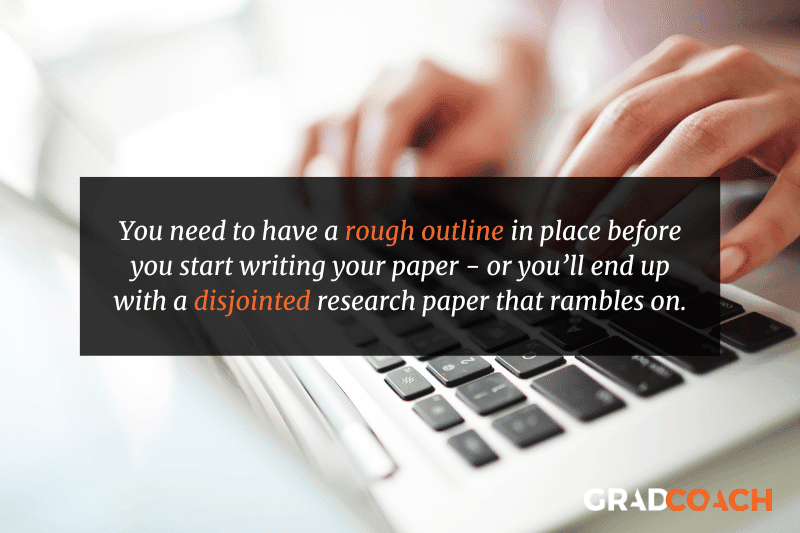
At this stage you might be wondering, “ But how should I structure my research paper? ”. Well, there’s no one-size-fits-all solution here, but in general, a research paper will consist of a few relatively standardised components:
- Introduction
- Literature review
- Methodology
Let’s take a look at each of these.
First up is the introduction section . As the name suggests, the purpose of the introduction is to set the scene for your research paper. There are usually (at least) four ingredients that go into this section – these are the background to the topic, the research problem and resultant research question , and the justification or rationale. If you’re interested, the video below unpacks the introduction section in more detail.
The next section of your research paper will typically be your literature review . Remember all that literature you worked through earlier? Well, this is where you’ll present your interpretation of all that content . You’ll do this by writing about recent trends, developments, and arguments within the literature – but more specifically, those that are relevant to your research question . The literature review can oftentimes seem a little daunting, even to seasoned researchers, so be sure to check out our extensive collection of literature review content here .
With the introduction and lit review out of the way, the next section of your paper is the research methodology . In a nutshell, the methodology section should describe to your reader what you did (beyond just reviewing the existing literature) to answer your research question. For example, what data did you collect, how did you collect that data, how did you analyse that data and so on? For each choice, you’ll also need to justify why you chose to do it that way, and what the strengths and weaknesses of your approach were.
Now, it’s worth mentioning that for some research papers, this aspect of the project may be a lot simpler . For example, you may only need to draw on secondary sources (in other words, existing data sets). In some cases, you may just be asked to draw your conclusions from the literature search itself (in other words, there may be no data analysis at all). But, if you are required to collect and analyse data, you’ll need to pay a lot of attention to the methodology section. The video below provides an example of what the methodology section might look like.
By this stage of your paper, you will have explained what your research question is, what the existing literature has to say about that question, and how you analysed additional data to try to answer your question. So, the natural next step is to present your analysis of that data . This section is usually called the “results” or “analysis” section and this is where you’ll showcase your findings.
Depending on your school’s requirements, you may need to present and interpret the data in one section – or you might split the presentation and the interpretation into two sections. In the latter case, your “results” section will just describe the data, and the “discussion” is where you’ll interpret that data and explicitly link your analysis back to your research question. If you’re not sure which approach to take, check in with your professor or take a look at past papers to see what the norms are for your programme.
Alright – once you’ve presented and discussed your results, it’s time to wrap it up . This usually takes the form of the “ conclusion ” section. In the conclusion, you’ll need to highlight the key takeaways from your study and close the loop by explicitly answering your research question. Again, the exact requirements here will vary depending on your programme (and you may not even need a conclusion section at all) – so be sure to check with your professor if you’re unsure.
Step 3: Write and refine
Finally, it’s time to get writing. All too often though, students hit a brick wall right about here… So, how do you avoid this happening to you?
Well, there’s a lot to be said when it comes to writing a research paper (or any sort of academic piece), but we’ll share three practical tips to help you get started.
First and foremost , it’s essential to approach your writing as an iterative process. In other words, you need to start with a really messy first draft and then polish it over multiple rounds of editing. Don’t waste your time trying to write a perfect research paper in one go. Instead, take the pressure off yourself by adopting an iterative approach.
Secondly , it’s important to always lean towards critical writing , rather than descriptive writing. What does this mean? Well, at the simplest level, descriptive writing focuses on the “ what ”, while critical writing digs into the “ so what ” – in other words, the implications . If you’re not familiar with these two types of writing, don’t worry! You can find a plain-language explanation here.
Last but not least, you’ll need to get your referencing right. Specifically, you’ll need to provide credible, correctly formatted citations for the statements you make. We see students making referencing mistakes all the time and it costs them dearly. The good news is that you can easily avoid this by using a simple reference manager . If you don’t have one, check out our video about Mendeley, an easy (and free) reference management tool that you can start using today.
Recap: Key Takeaways
We’ve covered a lot of ground here. To recap, the three steps to writing a high-quality research paper are:
- To choose a research question and review the literature
- To plan your paper structure and draft an outline
- To take an iterative approach to writing, focusing on critical writing and strong referencing
Remember, this is just a b ig-picture overview of the research paper development process and there’s a lot more nuance to unpack. So, be sure to grab a copy of our free research paper template to learn more about how to write a research paper.
Can you help me with a full paper template for this Abstract:
Background: Energy and sports drinks have gained popularity among diverse demographic groups, including adolescents, athletes, workers, and college students. While often used interchangeably, these beverages serve distinct purposes, with energy drinks aiming to boost energy and cognitive performance, and sports drinks designed to prevent dehydration and replenish electrolytes and carbohydrates lost during physical exertion.
Objective: To assess the nutritional quality of energy and sports drinks in Egypt.
Material and Methods: A cross-sectional study assessed the nutrient contents, including energy, sugar, electrolytes, vitamins, and caffeine, of sports and energy drinks available in major supermarkets in Cairo, Alexandria, and Giza, Egypt. Data collection involved photographing all relevant product labels and recording nutritional information. Descriptive statistics and appropriate statistical tests were employed to analyze and compare the nutritional values of energy and sports drinks.
Results: The study analyzed 38 sports drinks and 42 energy drinks. Sports drinks were significantly more expensive than energy drinks, with higher net content and elevated magnesium, potassium, and vitamin C. Energy drinks contained higher concentrations of caffeine, sugars, and vitamins B2, B3, and B6.
Conclusion: Significant nutritional differences exist between sports and energy drinks, reflecting their intended uses. However, these beverages’ high sugar content and calorie loads raise health concerns. Proper labeling, public awareness, and responsible marketing are essential to guide safe consumption practices in Egypt.
Submit a Comment Cancel reply
Your email address will not be published. Required fields are marked *
Save my name, email, and website in this browser for the next time I comment.
- Print Friendly
Home / Guides / Writing Guides / Paper Types / How to Write a Research Paper
How to Write a Research Paper
Research papers are a requirement for most college courses, so knowing how to write a research paper is important. These in-depth pieces of academic writing can seem pretty daunting, but there’s no need to panic. When broken down into its key components, writing your paper should be a manageable and, dare we say it, enjoyable task.
We’re going to look at the required elements of a paper in detail, and you might also find this webpage to be a useful reference .
Guide Overview
- What is a research paper?
- How to start a research paper
- Get clear instructions
- Brainstorm ideas
- Choose a topic
- Outline your outline
- Make friends with your librarian
- Find quality sources
- Understand your topic
- A detailed outline
- Keep it factual
- Finalize your thesis statement
- Think about format
- Cite, cite and cite
- The editing process
- Final checks
What is a Research Paper?
A research paper is more than just an extra long essay or encyclopedic regurgitation of facts and figures. The aim of this task is to combine in-depth study of a particular topic with critical thinking and evaluation by the student—that’s you!
There are two main types of research paper: argumentative and analytical.
Argumentative — takes a stance on a particular topic right from the start, with the aim of persuading the reader of the validity of the argument. These are best suited to topics that are debatable or controversial.
Analytical — takes no firm stance on a topic initially. Instead it asks a question and should come to an answer through the evaluation of source material. As its name suggests, the aim is to analyze the source material and offer a fresh perspective on the results.
If you wish to further your understanding, you can learn more here .
A required word count (think thousands!) can make writing that paper seem like an insurmountable task. Don’t worry! Our step-by-step guide will help you write that killer paper with confidence.
How to Start a Research Paper
Don’t rush ahead. Taking care during the planning and preparation stage will save time and hassle later.
Get Clear Instructions
Your lecturer or professor is your biggest ally—after all, they want you to do well. Make sure you get clear guidance from them on both the required format and preferred topics. In some cases, your tutor will assign a topic, or give you a set list to choose from. Often, however, you’ll be expected to select a suitable topic for yourself.
Having a research paper example to look at can also be useful for first-timers, so ask your tutor to supply you with one.
Brainstorm Ideas
Brainstorming research paper ideas is the first step to selecting a topic—and there are various methods you can use to brainstorm, including clustering (also known as mind mapping). Think about the research paper topics that interest you, and identify topics you have a strong opinion on.
Choose a Topic
Once you have a list of potential research paper topics, narrow them down by considering your academic strengths and ‘gaps in the market,’ e.g., don’t choose a common topic that’s been written about many times before. While you want your topic to be fresh and interesting, you also need to ensure there’s enough material available for you to work with. Similarly, while you shouldn’t go for easy research paper topics just for the sake of giving yourself less work, you do need to choose a topic that you feel confident you can do justice to.
Outline Your Outline
It might not be possible to form a full research paper outline until you’ve done some information gathering, but you can think about your overall aim; basically what you want to show and how you’re going to show it. Now’s also a good time to consider your thesis statement, although this might change as you delve into your source material deeper.
Researching the Research
Now it’s time to knuckle down and dig out all the information that’s relevant to your topic. Here are some tips.
Make Friends With Your Librarian
While lots of information gathering can be carried out online from anywhere, there’s still a place for old-fashioned study sessions in the library. A good librarian can help you to locate sources quickly and easily, and might even make suggestions that you hadn’t thought of. They’re great at helping you study and research, but probably can’t save you the best desk by the window.
Find Quality Sources
Not all sources are created equal, so make sure that you’re referring to reputable, reliable information. Examples of sources could include books, magazine articles, scholarly articles, reputable websites, databases and journals. Keywords relating to your topic can help you in your search.
As you search, you should begin to compile a list of references. This will make it much easier later when you are ready to build your paper’s bibliography. Keeping clear notes detailing any sources that you use will help you to avoid accidentally plagiarizing someone else’s work or ideas.
Understand Your Topic
Simply regurgitating facts and figures won’t make for an interesting paper. It’s essential that you fully understand your topic so you can come across as an authority on the subject and present your own ideas on it. You should read around your topic as widely as you can, before narrowing your area of interest for your paper, and critically analyzing your findings.
A Detailed Outline
Once you’ve got a firm grip on your subject and the source material available to you, formulate a detailed outline, including your thesis statement and how you are going to support it. The structure of your paper will depend on the subject type—ask a tutor for a research paper outline example if you’re unsure.
Get Writing!
If you’ve fully understood your topic and gathered quality source materials, bringing it all together should actually be the easy part!
Keep it Factual
There’s no place for sloppy writing in this kind of academic task, so keep your language simple and clear, and your points critical and succinct. The creative part is finding innovative angles and new insights on the topic to make your paper interesting.
Don’t forget about our verb , preposition , and adverb pages. You may find useful information to help with your writing!
Finalize Your Thesis Statement
You should now be in a position to finalize your thesis statement, showing clearly what your paper will show, answer or prove. This should usually be a one or two sentence statement; however, it’s the core idea of your paper, and every insight that you include should be relevant to it. Remember, a thesis statement is not merely a summary of your findings. It should present an argument or perspective that the rest of your paper aims to support.
Think About Format
The required style of your research paper format will usually depend on your subject area. For example, APA format is normally used for social science subjects, while MLA style is most commonly used for liberal arts and humanities. Still, there are thousands of more styles . Your tutor should be able to give you clear guidance on how to format your paper, how to structure it, and what elements it should include. Make sure that you follow their instruction. If possible, ask to see a sample research paper in the required format.
Cite, Cite and Cite
As all research paper topics invariably involve referring to other people’s work, it’s vital that you know how to properly cite your sources to avoid unintentional plagiarism. Whether you’re paraphrasing (putting someone else’s ideas into your own words) or directly quoting, the original source needs to be referenced. What style of citation formatting you use will depend on the requirements of your instructor, with common styles including APA and MLA format , which consist of in-text citations (short citations within the text, enclosed with parentheses) and a reference/works cited list.
The Editing Process
It’s likely that your paper will go through several drafts before you arrive at the very best version. The editing process is your chance to fix any weak points in your paper before submission. You might find that it needs a better balance of both primary and secondary sources (click through to find more info on the difference), that an adjective could use tweaking, or that you’ve included sources that aren’t relevant or credible. You might even feel that you need to be clearer in your argument, more thorough in your critical analysis, or more balanced in your evaluation.
From a stylistic point of view, you want to ensure that your writing is clear, simple and concise, with no long, rambling sentences or paragraphs. Keeping within the required word count parameters is also important, and another thing to keep in mind is the inclusion of gender-neutral language, to avoid the reinforcement of tired stereotypes.
Don’t forget about our other pages! If you are looking for help with other grammar-related topics, check out our noun , pronoun , and conjunction pages.
Final Checks
Once you’re happy with the depth and balance of the arguments and points presented, you can turn your attention to the finer details, such as formatting, spelling, punctuation, grammar and ensuring that your citations are all present and correct. The EasyBib Plus plagiarism checker is a handy tool for making sure that your sources are all cited. An EasyBib Plus subscription also comes with access to citation tools that can help you create citations in your choice of format.
Also, double-check your deadline date and the submissions guidelines to avoid any last-minute issues. Take a peek at our other grammar pages while you’re at it. We’ve included numerous links on this page, but we also have an interjection page and determiner page.
So you’ve done your final checks and handed in your paper according to the submissions guidelines and preferably before deadline day. Congratulations! If your schedule permits, now would be a great time to take a break from your studies. Maybe plan a fun activity with friends or just take the opportunity to rest and relax. A well-earned break from the books will ensure that you return to class refreshed and ready for your next stage of learning—and the next research paper requirement your tutor sets!
EasyBib Writing Resources
Writing a paper.
- Academic Essay
- Argumentative Essay
- College Admissions Essay
- Expository Essay
- Persuasive Essay
- Research Paper
- Thesis Statement
- Writing a Conclusion
- Writing an Introduction
- Writing an Outline
- Writing a Summary
EasyBib Plus Features
- Citation Generator
- Essay Checker
- Expert Check Proofreader
- Grammar Checker
- Paraphrasing Tools
Plagiarism Checker
- Spell Checker
How useful was this post?
Click on a star to rate it!
We are sorry that this post was not useful for you!
Let us improve this post!
Tell us how we can improve this post?
Grammar and Plagiarism Checkers
Grammar Basics
Plagiarism Basics
Writing Basics
Upload a paper to check for plagiarism against billions of sources and get advanced writing suggestions for clarity and style.
Get Started
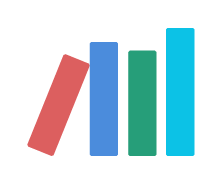
- Ask LitCharts AI
- Discussion Question Generator
- Essay Prompt Generator
- Quiz Question Generator
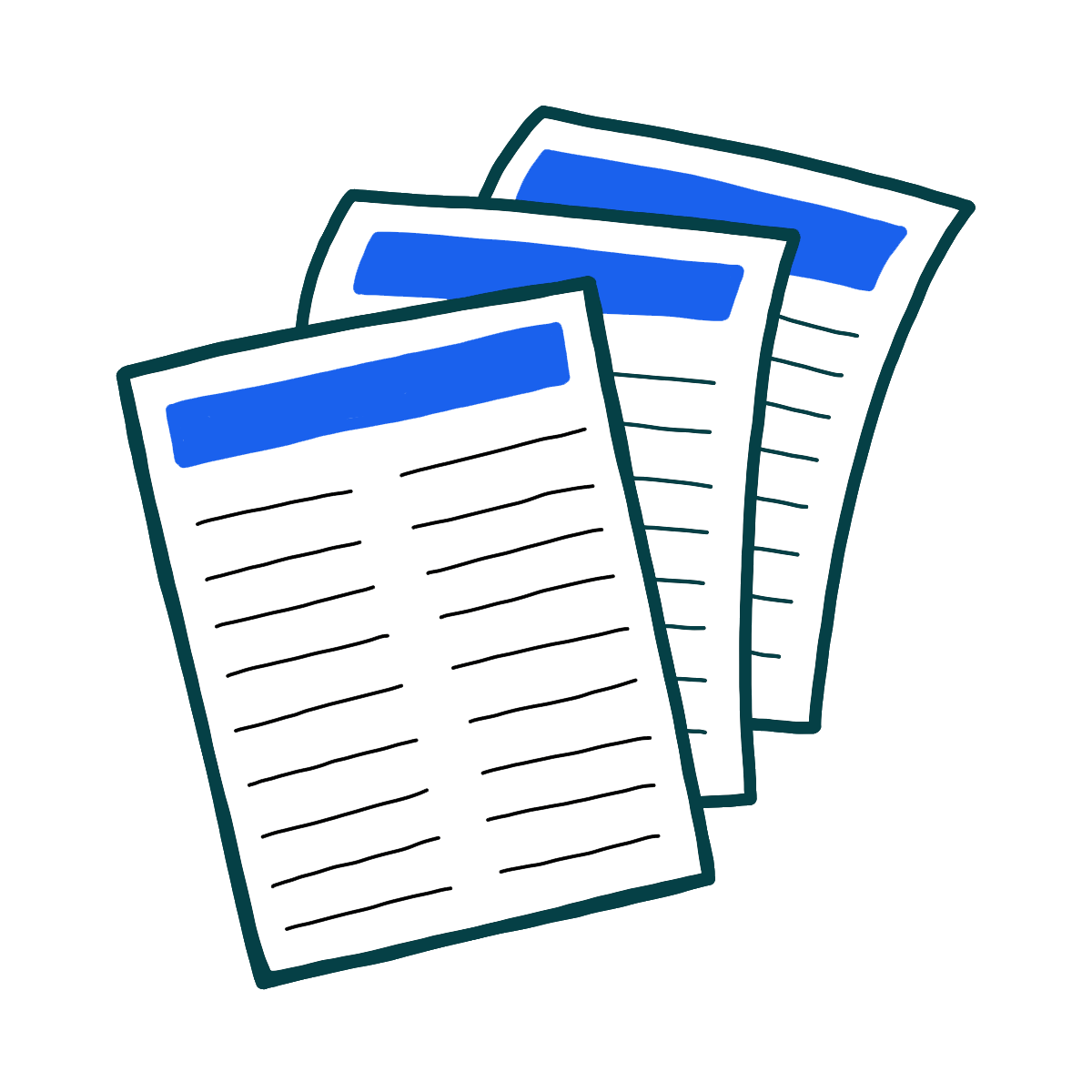
- Literature Guides
- Poetry Guides
- Shakespeare Translations
- Literary Terms
How to Write a Research Paper
Use the links below to jump directly to any section of this guide:
Research Paper Fundamentals
How to choose a topic or question, how to create a working hypothesis or thesis, common research paper methodologies, how to gather and organize evidence , how to write an outline for your research paper, how to write a rough draft, how to revise your draft, how to produce a final draft, resources for teachers .
It is not fair to say that no one writes anymore. Just about everyone writes text messages, brief emails, or social media posts every single day. Yet, most people don't have a lot of practice with the formal, organized writing required for a good academic research paper. This guide contains links to a variety of resources that can help demystify the process. Some of these resources are intended for teachers; they contain exercises, activities, and teaching strategies. Other resources are intended for direct use by students who are struggling to write papers, or are looking for tips to make the process go more smoothly.
The resources in this section are designed to help students understand the different types of research papers, the general research process, and how to manage their time. Below, you'll find links from university writing centers, the trusted Purdue Online Writing Lab, and more.
What is an Academic Research Paper?
"Genre and the Research Paper" (Purdue OWL)
There are different types of research papers. Different types of scholarly questions will lend themselves to one format or another. This is a brief introduction to the two main genres of research paper: analytic and argumentative.
"7 Most Popular Types of Research Papers" (Personal-writer.com)
This resource discusses formats that high school students commonly encounter, such as the compare and contrast essay and the definitional essay. Please note that the inclusion of this link is not an endorsement of this company's paid service.
How to Prepare and Plan Out Writing a Research Paper
Teachers can give their students a step-by-step guide like these to help them understand the different steps of the research paper process. These guides can be combined with the time management tools in the next subsection to help students come up with customized calendars for completing their papers.
"Ten Steps for Writing Research Papers" (American University)
This resource from American University is a comprehensive guide to the research paper writing process, and includes examples of proper research questions and thesis topics.
"Steps in Writing a Research Paper" (SUNY Empire State College)
This guide breaks the research paper process into 11 steps. Each "step" links to a separate page, which describes the work entailed in completing it.
How to Manage Time Effectively
The links below will help students determine how much time is necessary to complete a paper. If your sources are not available online or at your local library, you'll need to leave extra time for the Interlibrary Loan process. Remember that, even if you do not need to consult secondary sources, you'll still need to leave yourself ample time to organize your thoughts.
"Research Paper Planner: Timeline" (Baylor University)
This interactive resource from Baylor University creates a suggested writing schedule based on how much time a student has to work on the assignment.
"Research Paper Planner" (UCLA)
UCLA's library offers this step-by-step guide to the research paper writing process, which also includes a suggested planning calendar.
There's a reason teachers spend a long time talking about choosing a good topic. Without a good topic and a well-formulated research question, it is almost impossible to write a clear and organized paper. The resources below will help you generate ideas and formulate precise questions.
"How to Select a Research Topic" (Univ. of Michigan-Flint)
This resource is designed for college students who are struggling to come up with an appropriate topic. A student who uses this resource and still feels unsure about his or her topic should consult the course instructor for further personalized assistance.
"25 Interesting Research Paper Topics to Get You Started" (Kibin)
This resource, which is probably most appropriate for high school students, provides a list of specific topics to help get students started. It is broken into subsections, such as "paper topics on local issues."
"Writing a Good Research Question" (Grand Canyon University)
This introduction to research questions includes some embedded videos, as well as links to scholarly articles on research questions. This resource would be most appropriate for teachers who are planning lessons on research paper fundamentals.
"How to Write a Research Question the Right Way" (Kibin)
This student-focused resource provides more detail on writing research questions. The language is accessible, and there are embedded videos and examples of good and bad questions.
It is important to have a rough hypothesis or thesis in mind at the beginning of the research process. People who have a sense of what they want to say will have an easier time sorting through scholarly sources and other information. The key, of course, is not to become too wedded to the draft hypothesis or thesis. Just about every working thesis gets changed during the research process.
CrashCourse Video: "Sociology Research Methods" (YouTube)
Although this video is tailored to sociology students, it is applicable to students in a variety of social science disciplines. This video does a good job demonstrating the connection between the brainstorming that goes into selecting a research question and the formulation of a working hypothesis.
"How to Write a Thesis Statement for an Analytical Essay" (YouTube)
Students writing analytical essays will not develop the same type of working hypothesis as students who are writing research papers in other disciplines. For these students, developing the working thesis may happen as a part of the rough draft (see the relevant section below).
"Research Hypothesis" (Oakland Univ.)
This resource provides some examples of hypotheses in social science disciplines like Political Science and Criminal Justice. These sample hypotheses may also be useful for students in other soft social sciences and humanities disciplines like History.
When grading a research paper, instructors look for a consistent methodology. This section will help you understand different methodological approaches used in research papers. Students will get the most out of these resources if they use them to help prepare for conversations with teachers or discussions in class.
"Types of Research Designs" (USC)
A "research design," used for complex papers, is related to the paper's method. This resource contains introductions to a variety of popular research designs in the social sciences. Although it is not the most intuitive site to read, the information here is very valuable.
"Major Research Methods" (YouTube)
Although this video is a bit on the dry side, it provides a comprehensive overview of the major research methodologies in a format that might be more accessible to students who have struggled with textbooks or other written resources.
"Humanities Research Strategies" (USC)
This is a portal where students can learn about four methodological approaches for humanities papers: Historical Methodologies, Textual Criticism, Conceptual Analysis, and the Synoptic method.
"Selected Major Social Science Research Methods: Overview" (National Academies Press)
This appendix from the book Using Science as Evidence in Public Policy , printed by National Academies Press, introduces some methods used in social science papers.
"Organizing Your Social Sciences Research Paper: 6. The Methodology" (USC)
This resource from the University of Southern California's library contains tips for writing a methodology section in a research paper.
How to Determine the Best Methodology for You
Anyone who is new to writing research papers should be sure to select a method in consultation with their instructor. These resources can be used to help prepare for that discussion. They may also be used on their own by more advanced students.
"Choosing Appropriate Research Methodologies" (Palgrave Study Skills)
This friendly and approachable resource from Palgrave Macmillan can be used by students who are just starting to think about appropriate methodologies.
"How to Choose Your Research Methods" (NFER (UK))
This is another approachable resource students can use to help narrow down the most appropriate methods for their research projects.
The resources in this section introduce the process of gathering scholarly sources and collecting evidence. You'll find a range of material here, from introductory guides to advanced explications best suited to college students. Please consult the LitCharts How to Do Academic Research guide for a more comprehensive list of resources devoted to finding scholarly literature.
Google Scholar
Students who have access to library websites with detailed research guides should start there, but people who do not have access to those resources can begin their search for secondary literature here.
"Gathering Appropriate Information" (Texas Gateway)
This resource from the Texas Gateway for online resources introduces students to the research process, and contains interactive exercises. The level of complexity is suitable for middle school, high school, and introductory college classrooms.
"An Overview of Quantitative and Qualitative Data Collection Methods" (NSF)
This PDF from the National Science Foundation goes into detail about best practices and pitfalls in data collection across multiple types of methodologies.
"Social Science Methods for Data Collection and Analysis" (Swiss FIT)
This resource is appropriate for advanced undergraduates or teachers looking to create lessons on research design and data collection. It covers techniques for gathering data via interviews, observations, and other methods.
"Collecting Data by In-depth Interviewing" (Leeds Univ.)
This resource contains enough information about conducting interviews to make it useful for teachers who want to create a lesson plan, but is also accessible enough for college juniors or seniors to make use of it on their own.
There is no "one size fits all" outlining technique. Some students might devote all their energy and attention to the outline in order to avoid the paper. Other students may benefit from being made to sit down and organize their thoughts into a lengthy sentence outline. The resources in this section include strategies and templates for multiple types of outlines.
"Topic vs. Sentence Outlines" (UC Berkeley)
This resource introduces two basic approaches to outlining: the shorter topic-based approach, and the longer, more detailed sentence-based approach. This resource also contains videos on how to develop paper paragraphs from the sentence-based outline.
"Types of Outlines and Samples" (Purdue OWL)
The Purdue Online Writing Lab's guide is a slightly less detailed discussion of different types of outlines. It contains several sample outlines.
"Writing An Outline" (Austin C.C.)
This resource from a community college contains sample outlines from an American history class that students can use as models.
"How to Structure an Outline for a College Paper" (YouTube)
This brief (sub-2 minute) video from the ExpertVillage YouTube channel provides a model of outline writing for students who are struggling with the idea.
"Outlining" (Harvard)
This is a good resource to consult after completing a draft outline. It offers suggestions for making sure your outline avoids things like unnecessary repetition.
As with outlines, rough drafts can take on many different forms. These resources introduce teachers and students to the various approaches to writing a rough draft. This section also includes resources that will help you cite your sources appropriately according to the MLA, Chicago, and APA style manuals.
"Creating a Rough Draft for a Research Paper" (Univ. of Minnesota)
This resource is useful for teachers in particular, as it provides some suggested exercises to help students with writing a basic rough draft.
Rough Draft Assignment (Duke of Definition)
This sample assignment, with a brief list of tips, was developed by a high school teacher who runs a very successful and well-reviewed page of educational resources.
"Creating the First Draft of Your Research Paper" (Concordia Univ.)
This resource will be helpful for perfectionists or procrastinators, as it opens by discussing the problem of avoiding writing. It also provides a short list of suggestions meant to get students writing.
Using Proper Citations
There is no such thing as a rough draft of a scholarly citation. These links to the three major citation guides will ensure that your citations follow the correct format. Please consult the LitCharts How to Cite Your Sources guide for more resources.
Chicago Manual of Style Citation Guide
Some call The Chicago Manual of Style , which was first published in 1906, "the editors' Bible." The manual is now in its 17th edition, and is popular in the social sciences, historical journals, and some other fields in the humanities.
APA Citation Guide
According to the American Psychological Association, this guide was developed to aid reading comprehension, clarity of communication, and to reduce bias in language in the social and behavioral sciences. Its first full edition was published in 1952, and it is now in its sixth edition.
MLA Citation Guide
The Modern Language Association style is used most commonly within the liberal arts and humanities. The MLA Style Manual and Guide to Scholarly Publishing was first published in 1985 and (as of 2008) is in its third edition.
Any professional scholar will tell you that the best research papers are made in the revision stage. No matter how strong your research question or working thesis, it is not possible to write a truly outstanding paper without devoting energy to revision. These resources provide examples of revision exercises for the classroom, as well as tips for students working independently.
"The Art of Revision" (Univ. of Arizona)
This resource provides a wealth of information and suggestions for both students and teachers. There is a list of suggested exercises that teachers might use in class, along with a revision checklist that is useful for teachers and students alike.
"Script for Workshop on Revision" (Vanderbilt University)
Vanderbilt's guide for leading a 50-minute revision workshop can serve as a model for teachers who wish to guide students through the revision process during classtime.
"Revising Your Paper" (Univ. of Washington)
This detailed handout was designed for students who are beginning the revision process. It discusses different approaches and methods for revision, and also includes a detailed list of things students should look for while they revise.
"Revising Drafts" (UNC Writing Center)
This resource is designed for students and suggests things to look for during the revision process. It provides steps for the process and has a FAQ for students who have questions about why it is important to revise.
Conferencing with Writing Tutors and Instructors
No writer is so good that he or she can't benefit from meeting with instructors or peer tutors. These resources from university writing, learning, and communication centers provide suggestions for how to get the most out of these one-on-one meetings.
"Getting Feedback" (UNC Writing Center)
This very helpful resource talks about how to ask for feedback during the entire writing process. It contains possible questions that students might ask when developing an outline, during the revision process, and after the final draft has been graded.
"Prepare for Your Tutoring Session" (Otis College of Art and Design)
This guide from a university's student learning center contains a lot of helpful tips for getting the most out of working with a writing tutor.
"The Importance of Asking Your Professor" (Univ. of Waterloo)
This article from the university's Writing and Communication Centre's blog contains some suggestions for how and when to get help from professors and Teaching Assistants.
Once you've revised your first draft, you're well on your way to handing in a polished paper. These resources—each of them produced by writing professionals at colleges and universities—outline the steps required in order to produce a final draft. You'll find proofreading tips and checklists in text and video form.
"Developing a Final Draft of a Research Paper" (Univ. of Minnesota)
While this resource contains suggestions for revision, it also features a couple of helpful checklists for the last stages of completing a final draft.
Basic Final Draft Tips and Checklist (Univ. of Maryland-University College)
This short and accessible resource, part of UMUC's very thorough online guide to writing and research, contains a very basic checklist for students who are getting ready to turn in their final drafts.
Final Draft Checklist (Everett C.C.)
This is another accessible final draft checklist, appropriate for both high school and college students. It suggests reading your essay aloud at least once.
"How to Proofread Your Final Draft" (YouTube)
This video (approximately 5 minutes), produced by Eastern Washington University, gives students tips on proofreading final drafts.
"Proofreading Tips" (Georgia Southern-Armstrong)
This guide will help students learn how to spot common errors in their papers. It suggests focusing on content and editing for grammar and mechanics.
This final set of resources is intended specifically for high school and college instructors. It provides links to unit plans and classroom exercises that can help improve students' research and writing skills. You'll find resources that give an overview of the process, along with activities that focus on how to begin and how to carry out research.
"Research Paper Complete Resources Pack" (Teachers Pay Teachers)
This packet of assignments, rubrics, and other resources is designed for high school students. The resources in this packet are aligned to Common Core standards.
"Research Paper—Complete Unit" (Teachers Pay Teachers)
This packet of assignments, notes, PowerPoints, and other resources has a 4/4 rating with over 700 ratings. It is designed for high school teachers, but might also be useful to college instructors who work with freshmen.
"Teaching Students to Write Good Papers" (Yale)
This resource from Yale's Center for Teaching and Learning is designed for college instructors, and it includes links to appropriate activities and exercises.
"Research Paper Writing: An Overview" (CUNY Brooklyn)
CUNY Brooklyn offers this complete lesson plan for introducing students to research papers. It includes an accompanying set of PowerPoint slides.
"Lesson Plan: How to Begin Writing a Research Paper" (San Jose State Univ.)
This lesson plan is designed for students in the health sciences, so teachers will have to modify it for their own needs. It includes a breakdown of the brainstorming, topic selection, and research question process.
"Quantitative Techniques for Social Science Research" (Univ. of Pittsburgh)
This is a set of PowerPoint slides that can be used to introduce students to a variety of quantitative methods used in the social sciences.
- PDFs for all 136 Lit Terms we cover
- Downloads of 2003 LitCharts Lit Guides
- Teacher Editions for every Lit Guide
- Explanations and citation info for 42,322 quotes across 2003 books
- Downloadable (PDF) line-by-line translations of every Shakespeare play
Need something? Request a new guide .
How can we improve? Share feedback .
LitCharts is hiring!

- Quizzes, saving guides, requests, plus so much more.
Instant insights, infinite possibilities
- How to write a research paper
Last updated
11 January 2024
Reviewed by
With proper planning, knowledge, and framework, completing a research paper can be a fulfilling and exciting experience.
Though it might initially sound slightly intimidating, this guide will help you embrace the challenge.
By documenting your findings, you can inspire others and make a difference in your field. Here's how you can make your research paper unique and comprehensive.
- What is a research paper?
Research papers allow you to demonstrate your knowledge and understanding of a particular topic. These papers are usually lengthier and more detailed than typical essays, requiring deeper insight into the chosen topic.
To write a research paper, you must first choose a topic that interests you and is relevant to the field of study. Once you’ve selected your topic, gathering as many relevant resources as possible, including books, scholarly articles, credible websites, and other academic materials, is essential. You must then read and analyze these sources, summarizing their key points and identifying gaps in the current research.
You can formulate your ideas and opinions once you thoroughly understand the existing research. To get there might involve conducting original research, gathering data, or analyzing existing data sets. It could also involve presenting an original argument or interpretation of the existing research.
Writing a successful research paper involves presenting your findings clearly and engagingly, which might involve using charts, graphs, or other visual aids to present your data and using concise language to explain your findings. You must also ensure your paper adheres to relevant academic formatting guidelines, including proper citations and references.
Overall, writing a research paper requires a significant amount of time, effort, and attention to detail. However, it is also an enriching experience that allows you to delve deeply into a subject that interests you and contribute to the existing body of knowledge in your chosen field.
- How long should a research paper be?
Research papers are deep dives into a topic. Therefore, they tend to be longer pieces of work than essays or opinion pieces.
However, a suitable length depends on the complexity of the topic and your level of expertise. For instance, are you a first-year college student or an experienced professional?
Also, remember that the best research papers provide valuable information for the benefit of others. Therefore, the quality of information matters most, not necessarily the length. Being concise is valuable.
Following these best practice steps will help keep your process simple and productive:
1. Gaining a deep understanding of any expectations
Before diving into your intended topic or beginning the research phase, take some time to orient yourself. Suppose there’s a specific topic assigned to you. In that case, it’s essential to deeply understand the question and organize your planning and approach in response. Pay attention to the key requirements and ensure you align your writing accordingly.
This preparation step entails
Deeply understanding the task or assignment
Being clear about the expected format and length
Familiarizing yourself with the citation and referencing requirements
Understanding any defined limits for your research contribution
Where applicable, speaking to your professor or research supervisor for further clarification
2. Choose your research topic
Select a research topic that aligns with both your interests and available resources. Ideally, focus on a field where you possess significant experience and analytical skills. In crafting your research paper, it's crucial to go beyond summarizing existing data and contribute fresh insights to the chosen area.
Consider narrowing your focus to a specific aspect of the topic. For example, if exploring the link between technology and mental health, delve into how social media use during the pandemic impacts the well-being of college students. Conducting interviews and surveys with students could provide firsthand data and unique perspectives, adding substantial value to the existing knowledge.
When finalizing your topic, adhere to legal and ethical norms in the relevant area (this ensures the integrity of your research, protects participants' rights, upholds intellectual property standards, and ensures transparency and accountability). Following these principles not only maintains the credibility of your work but also builds trust within your academic or professional community.
For instance, in writing about medical research, consider legal and ethical norms , including patient confidentiality laws and informed consent requirements. Similarly, if analyzing user data on social media platforms, be mindful of data privacy regulations, ensuring compliance with laws governing personal information collection and use. Aligning with legal and ethical standards not only avoids potential issues but also underscores the responsible conduct of your research.
3. Gather preliminary research
Once you’ve landed on your topic, it’s time to explore it further. You’ll want to discover more about available resources and existing research relevant to your assignment at this stage.
This exploratory phase is vital as you may discover issues with your original idea or realize you have insufficient resources to explore the topic effectively. This key bit of groundwork allows you to redirect your research topic in a different, more feasible, or more relevant direction if necessary.
Spending ample time at this stage ensures you gather everything you need, learn as much as you can about the topic, and discover gaps where the topic has yet to be sufficiently covered, offering an opportunity to research it further.
4. Define your research question
To produce a well-structured and focused paper, it is imperative to formulate a clear and precise research question that will guide your work. Your research question must be informed by the existing literature and tailored to the scope and objectives of your project. By refining your focus, you can produce a thoughtful and engaging paper that effectively communicates your ideas to your readers.
5. Write a thesis statement
A thesis statement is a one-to-two-sentence summary of your research paper's main argument or direction. It serves as an overall guide to summarize the overall intent of the research paper for you and anyone wanting to know more about the research.
A strong thesis statement is:
Concise and clear: Explain your case in simple sentences (avoid covering multiple ideas). It might help to think of this section as an elevator pitch.
Specific: Ensure that there is no ambiguity in your statement and that your summary covers the points argued in the paper.
Debatable: A thesis statement puts forward a specific argument––it is not merely a statement but a debatable point that can be analyzed and discussed.
Here are three thesis statement examples from different disciplines:
Psychology thesis example: "We're studying adults aged 25-40 to see if taking short breaks for mindfulness can help with stress. Our goal is to find practical ways to manage anxiety better."
Environmental science thesis example: "This research paper looks into how having more city parks might make the air cleaner and keep people healthier. I want to find out if more green spaces means breathing fewer carcinogens in big cities."
UX research thesis example: "This study focuses on improving mobile banking for older adults using ethnographic research, eye-tracking analysis, and interactive prototyping. We investigate the usefulness of eye-tracking analysis with older individuals, aiming to spark debate and offer fresh perspectives on UX design and digital inclusivity for the aging population."
6. Conduct in-depth research
A research paper doesn’t just include research that you’ve uncovered from other papers and studies but your fresh insights, too. You will seek to become an expert on your topic––understanding the nuances in the current leading theories. You will analyze existing research and add your thinking and discoveries. It's crucial to conduct well-designed research that is rigorous, robust, and based on reliable sources. Suppose a research paper lacks evidence or is biased. In that case, it won't benefit the academic community or the general public. Therefore, examining the topic thoroughly and furthering its understanding through high-quality research is essential. That usually means conducting new research. Depending on the area under investigation, you may conduct surveys, interviews, diary studies , or observational research to uncover new insights or bolster current claims.
7. Determine supporting evidence
Not every piece of research you’ve discovered will be relevant to your research paper. It’s important to categorize the most meaningful evidence to include alongside your discoveries. It's important to include evidence that doesn't support your claims to avoid exclusion bias and ensure a fair research paper.
8. Write a research paper outline
Before diving in and writing the whole paper, start with an outline. It will help you to see if more research is needed, and it will provide a framework by which to write a more compelling paper. Your supervisor may even request an outline to approve before beginning to write the first draft of the full paper. An outline will include your topic, thesis statement, key headings, short summaries of the research, and your arguments.
9. Write your first draft
Once you feel confident about your outline and sources, it’s time to write your first draft. While penning a long piece of content can be intimidating, if you’ve laid the groundwork, you will have a structure to help you move steadily through each section. To keep up motivation and inspiration, it’s often best to keep the pace quick. Stopping for long periods can interrupt your flow and make jumping back in harder than writing when things are fresh in your mind.
10. Cite your sources correctly
It's always a good practice to give credit where it's due, and the same goes for citing any works that have influenced your paper. Building your arguments on credible references adds value and authenticity to your research. In the formatting guidelines section, you’ll find an overview of different citation styles (MLA, CMOS, or APA), which will help you meet any publishing or academic requirements and strengthen your paper's credibility. It is essential to follow the guidelines provided by your school or the publication you are submitting to ensure the accuracy and relevance of your citations.
11. Ensure your work is original
It is crucial to ensure the originality of your paper, as plagiarism can lead to serious consequences. To avoid plagiarism, you should use proper paraphrasing and quoting techniques. Paraphrasing is rewriting a text in your own words while maintaining the original meaning. Quoting involves directly citing the source. Giving credit to the original author or source is essential whenever you borrow their ideas or words. You can also use plagiarism detection tools such as Scribbr or Grammarly to check the originality of your paper. These tools compare your draft writing to a vast database of online sources. If you find any accidental plagiarism, you should correct it immediately by rephrasing or citing the source.
12. Revise, edit, and proofread
One of the essential qualities of excellent writers is their ability to understand the importance of editing and proofreading. Even though it's tempting to call it a day once you've finished your writing, editing your work can significantly improve its quality. It's natural to overlook the weaker areas when you've just finished writing a paper. Therefore, it's best to take a break of a day or two, or even up to a week, to refresh your mind. This way, you can return to your work with a new perspective. After some breathing room, you can spot any inconsistencies, spelling and grammar errors, typos, or missing citations and correct them.
- The best research paper format
The format of your research paper should align with the requirements set forth by your college, school, or target publication.
There is no one “best” format, per se. Depending on the stated requirements, you may need to include the following elements:
Title page: The title page of a research paper typically includes the title, author's name, and institutional affiliation and may include additional information such as a course name or instructor's name.
Table of contents: Include a table of contents to make it easy for readers to find specific sections of your paper.
Abstract: The abstract is a summary of the purpose of the paper.
Methods : In this section, describe the research methods used. This may include collecting data , conducting interviews, or doing field research .
Results: Summarize the conclusions you drew from your research in this section.
Discussion: In this section, discuss the implications of your research . Be sure to mention any significant limitations to your approach and suggest areas for further research.
Tables, charts, and illustrations: Use tables, charts, and illustrations to help convey your research findings and make them easier to understand.
Works cited or reference page: Include a works cited or reference page to give credit to the sources that you used to conduct your research.
Bibliography: Provide a list of all the sources you consulted while conducting your research.
Dedication and acknowledgments : Optionally, you may include a dedication and acknowledgments section to thank individuals who helped you with your research.
- General style and formatting guidelines
Formatting your research paper means you can submit it to your college, journal, or other publications in compliance with their criteria.
Research papers tend to follow the American Psychological Association (APA), Modern Language Association (MLA), or Chicago Manual of Style (CMOS) guidelines.
Here’s how each style guide is typically used:
Chicago Manual of Style (CMOS):
CMOS is a versatile style guide used for various types of writing. It's known for its flexibility and use in the humanities. CMOS provides guidelines for citations, formatting, and overall writing style. It allows for both footnotes and in-text citations, giving writers options based on their preferences or publication requirements.
American Psychological Association (APA):
APA is common in the social sciences. It’s hailed for its clarity and emphasis on precision. It has specific rules for citing sources, creating references, and formatting papers. APA style uses in-text citations with an accompanying reference list. It's designed to convey information efficiently and is widely used in academic and scientific writing.
Modern Language Association (MLA):
MLA is widely used in the humanities, especially literature and language studies. It emphasizes the author-page format for in-text citations and provides guidelines for creating a "Works Cited" page. MLA is known for its focus on the author's name and the literary works cited. It’s frequently used in disciplines that prioritize literary analysis and critical thinking.
To confirm you're using the latest style guide, check the official website or publisher's site for updates, consult academic resources, and verify the guide's publication date. Online platforms and educational resources may also provide summaries and alerts about any revisions or additions to the style guide.
Citing sources
When working on your research paper, it's important to cite the sources you used properly. Your citation style will guide you through this process. Generally, there are three parts to citing sources in your research paper:
First, provide a brief citation in the body of your essay. This is also known as a parenthetical or in-text citation.
Second, include a full citation in the Reference list at the end of your paper. Different types of citations include in-text citations, footnotes, and reference lists.
In-text citations include the author's surname and the date of the citation.
Footnotes appear at the bottom of each page of your research paper. They may also be summarized within a reference list at the end of the paper.
A reference list includes all of the research used within the paper at the end of the document. It should include the author, date, paper title, and publisher listed in the order that aligns with your citation style.
10 research paper writing tips:
Following some best practices is essential to writing a research paper that contributes to your field of study and creates a positive impact.
These tactics will help you structure your argument effectively and ensure your work benefits others:
Clear and precise language: Ensure your language is unambiguous. Use academic language appropriately, but keep it simple. Also, provide clear takeaways for your audience.
Effective idea separation: Organize the vast amount of information and sources in your paper with paragraphs and titles. Create easily digestible sections for your readers to navigate through.
Compelling intro: Craft an engaging introduction that captures your reader's interest. Hook your audience and motivate them to continue reading.
Thorough revision and editing: Take the time to review and edit your paper comprehensively. Use tools like Grammarly to detect and correct small, overlooked errors.
Thesis precision: Develop a clear and concise thesis statement that guides your paper. Ensure that your thesis aligns with your research's overall purpose and contribution.
Logical flow of ideas: Maintain a logical progression throughout the paper. Use transitions effectively to connect different sections and maintain coherence.
Critical evaluation of sources: Evaluate and critically assess the relevance and reliability of your sources. Ensure that your research is based on credible and up-to-date information.
Thematic consistency: Maintain a consistent theme throughout the paper. Ensure that all sections contribute cohesively to the overall argument.
Relevant supporting evidence: Provide concise and relevant evidence to support your arguments. Avoid unnecessary details that may distract from the main points.
Embrace counterarguments: Acknowledge and address opposing views to strengthen your position. Show that you have considered alternative arguments in your field.
7 research tips
If you want your paper to not only be well-written but also contribute to the progress of human knowledge, consider these tips to take your paper to the next level:
Selecting the appropriate topic: The topic you select should align with your area of expertise, comply with the requirements of your project, and have sufficient resources for a comprehensive investigation.
Use academic databases: Academic databases such as PubMed, Google Scholar, and JSTOR offer a wealth of research papers that can help you discover everything you need to know about your chosen topic.
Critically evaluate sources: It is important not to accept research findings at face value. Instead, it is crucial to critically analyze the information to avoid jumping to conclusions or overlooking important details. A well-written research paper requires a critical analysis with thorough reasoning to support claims.
Diversify your sources: Expand your research horizons by exploring a variety of sources beyond the standard databases. Utilize books, conference proceedings, and interviews to gather diverse perspectives and enrich your understanding of the topic.
Take detailed notes: Detailed note-taking is crucial during research and can help you form the outline and body of your paper.
Stay up on trends: Keep abreast of the latest developments in your field by regularly checking for recent publications. Subscribe to newsletters, follow relevant journals, and attend conferences to stay informed about emerging trends and advancements.
Engage in peer review: Seek feedback from peers or mentors to ensure the rigor and validity of your research . Peer review helps identify potential weaknesses in your methodology and strengthens the overall credibility of your findings.
- The real-world impact of research papers
Writing a research paper is more than an academic or business exercise. The experience provides an opportunity to explore a subject in-depth, broaden one's understanding, and arrive at meaningful conclusions. With careful planning, dedication, and hard work, writing a research paper can be a fulfilling and enriching experience contributing to advancing knowledge.
How do I publish my research paper?
Many academics wish to publish their research papers. While challenging, your paper might get traction if it covers new and well-written information. To publish your research paper, find a target publication, thoroughly read their guidelines, format your paper accordingly, and send it to them per their instructions. You may need to include a cover letter, too. After submission, your paper may be peer-reviewed by experts to assess its legitimacy, quality, originality, and methodology. Following review, you will be informed by the publication whether they have accepted or rejected your paper.
What is a good opening sentence for a research paper?
Beginning your research paper with a compelling introduction can ensure readers are interested in going further. A relevant quote, a compelling statistic, or a bold argument can start the paper and hook your reader. Remember, though, that the most important aspect of a research paper is the quality of the information––not necessarily your ability to storytell, so ensure anything you write aligns with your goals.
Research paper vs. a research proposal—what’s the difference?
While some may confuse research papers and proposals, they are different documents.
A research proposal comes before a research paper. It is a detailed document that outlines an intended area of exploration. It includes the research topic, methodology, timeline, sources, and potential conclusions. Research proposals are often required when seeking approval to conduct research.
A research paper is a summary of research findings. A research paper follows a structured format to present those findings and construct an argument or conclusion.
Should you be using a customer insights hub?
Do you want to discover previous research faster?
Do you share your research findings with others?
Do you analyze research data?
Start for free today, add your research, and get to key insights faster
Editor’s picks
Last updated: 18 April 2023
Last updated: 27 February 2023
Last updated: 22 August 2024
Last updated: 5 February 2023
Last updated: 16 August 2024
Last updated: 9 March 2023
Last updated: 30 April 2024
Last updated: 12 December 2023
Last updated: 11 March 2024
Last updated: 4 July 2024
Last updated: 6 March 2024
Last updated: 5 March 2024
Last updated: 13 May 2024
Latest articles
Related topics, .css-je19u9{-webkit-align-items:flex-end;-webkit-box-align:flex-end;-ms-flex-align:flex-end;align-items:flex-end;display:-webkit-box;display:-webkit-flex;display:-ms-flexbox;display:flex;-webkit-flex-direction:row;-ms-flex-direction:row;flex-direction:row;-webkit-box-flex-wrap:wrap;-webkit-flex-wrap:wrap;-ms-flex-wrap:wrap;flex-wrap:wrap;-webkit-box-pack:center;-ms-flex-pack:center;-webkit-justify-content:center;justify-content:center;row-gap:0;text-align:center;max-width:671px;}@media (max-width: 1079px){.css-je19u9{max-width:400px;}.css-je19u9>span{white-space:pre;}}@media (max-width: 799px){.css-je19u9{max-width:400px;}.css-je19u9>span{white-space:pre;}} decide what to .css-1kiodld{max-height:56px;display:-webkit-box;display:-webkit-flex;display:-ms-flexbox;display:flex;-webkit-align-items:center;-webkit-box-align:center;-ms-flex-align:center;align-items:center;}@media (max-width: 1079px){.css-1kiodld{display:none;}} build next, decide what to build next.
- 10 research paper
Log in or sign up
Get started for free
How to Write an APA Research Paper
Psychology/neuroscience 201, v iew in pdf format.
An APA-style paper includes the following sections: title page, abstract, introduction, method, results, discussion, and references. Your paper may also include one or more tables and/or figures. Different types of information about your study are addressed in each of the sections, as described below.
General formatting rules are as follows:
Do not put page breaks in between the introduction, method, results, and discussion sections.
The title page, abstract, references, table(s), and figure(s) should be on their own pages. The entire paper should be written in the past tense, in a 12-point font, double-spaced, and with one-inch margins all around.
(see sample on p. 41 of APA manual)
- Title should be between 10-12 words and should reflect content of paper (e.g., IV and DV).
- Title, your name, and Hamilton College are all double-spaced (no extra spaces)
- Create a page header using the “View header” function in MS Word. On the title page, the header should include the following: Flush left: Running head: THE RUNNING HEAD SHOULD BE IN ALL CAPITAL LETTERS. The running head is a short title that appears at the top of pages of published articles. It should not exceed 50 characters, including punctuation and spacing. (Note: on the title page, you actually write the words “Running head,” but these words do not appear on subsequent pages; just the actual running head does. If you make a section break between the title page and the rest of the paper you can make the header different for those two parts of the manuscript). Flush right, on same line: page number. Use the toolbox to insert a page number, so it will automatically number each page.
Abstract (labeled, centered, not bold)
No more than 120 words, one paragraph, block format (i.e., don’t indent), double-spaced.
- State topic, preferably in one sentence. Provide overview of method, results, and discussion.
Introduction
(Do not label as “Introduction.” Title of paper goes at the top of the page—not bold)
The introduction of an APA-style paper is the most difficult to write. A good introduction will summarize, integrate, and critically evaluate the empirical knowledge in the relevant area(s) in a way that sets the stage for your study and why you conducted it. The introduction starts out broad (but not too broad!) and gets more focused toward the end. Here are some guidelines for constructing a good introduction:
- Don’t put your readers to sleep by beginning your paper with the time-worn sentence, “Past research has shown (blah blah blah)” They’ll be snoring within a paragraph! Try to draw your reader in by saying something interesting or thought-provoking right off the bat. Take a look at articles you’ve read. Which ones captured your attention right away? How did the authors accomplish this task? Which ones didn’t? Why not? See if you can use articles you liked as a model. One way to begin (but not the only way) is to provide an example or anecdote illustrative of your topic area.
- Although you won’t go into the details of your study and hypotheses until the end of the intro, you should foreshadow your study a bit at the end of the first paragraph by stating your purpose briefly, to give your reader a schema for all the information you will present next.
- Your intro should be a logical flow of ideas that leads up to your hypothesis. Try to organize it in terms of the ideas rather than who did what when. In other words, your intro shouldn’t read like a story of “Schmirdley did such-and-such in 1991. Then Gurglehoff did something-or-other in 1993. Then....(etc.)” First, brainstorm all of the ideas you think are necessary to include in your paper. Next, decide which ideas make sense to present first, second, third, and so forth, and think about how you want to transition between ideas. When an idea is complex, don’t be afraid to use a real-life example to clarify it for your reader. The introduction will end with a brief overview of your study and, finally, your specific hypotheses. The hypotheses should flow logically out of everything that’s been presented, so that the reader has the sense of, “Of course. This hypothesis makes complete sense, given all the other research that was presented.”
- When incorporating references into your intro, you do not necessarily need to describe every single study in complete detail, particularly if different studies use similar methodologies. Certainly you want to summarize briefly key articles, though, and point out differences in methods or findings of relevant studies when necessary. Don’t make one mistake typical of a novice APA-paper writer by stating overtly why you’re including a particular article (e.g., “This article is relevant to my study because…”). It should be obvious to the reader why you’re including a reference without your explicitly saying so. DO NOT quote from the articles, instead paraphrase by putting the information in your own words.
- Be careful about citing your sources (see APA manual). Make sure there is a one-to-one correspondence between the articles you’ve cited in your intro and the articles listed in your reference section.
- Remember that your audience is the broader scientific community, not the other students in your class or your professor. Therefore, you should assume they have a basic understanding of psychology, but you need to provide them with the complete information necessary for them to understand the research you are presenting.
Method (labeled, centered, bold)
The Method section of an APA-style paper is the most straightforward to write, but requires precision. Your goal is to describe the details of your study in such a way that another researcher could duplicate your methods exactly.
The Method section typically includes Participants, Materials and/or Apparatus, and Procedure sections. If the design is particularly complicated (multiple IVs in a factorial experiment, for example), you might also include a separate Design subsection or have a “Design and Procedure” section.
Note that in some studies (e.g., questionnaire studies in which there are many measures to describe but the procedure is brief), it may be more useful to present the Procedure section prior to the Materials section rather than after it.
Participants (labeled, flush left, bold)
Total number of participants (# women, # men), age range, mean and SD for age, racial/ethnic composition (if applicable), population type (e.g., college students). Remember to write numbers out when they begin a sentence.
- How were the participants recruited? (Don’t say “randomly” if it wasn’t random!) Were they compensated for their time in any way? (e.g., money, extra credit points)
- Write for a broad audience. Thus, do not write, “Students in Psych. 280...” Rather, write (for instance), “Students in a psychological statistics and research methods course at a small liberal arts college….”
- Try to avoid short, choppy sentences. Combine information into a longer sentence when possible.
Materials (labeled, flush left, bold)
Carefully describe any stimuli, questionnaires, and so forth. It is unnecessary to mention things such as the paper and pencil used to record the responses, the data recording sheet, the computer that ran the data analysis, the color of the computer, and so forth.
- If you included a questionnaire, you should describe it in detail. For instance, note how many items were on the questionnaire, what the response format was (e.g., a 5-point Likert-type scale ranging from 1 (strongly disagree) to 5 (strongly agree)), how many items were reverse-scored, whether the measure had subscales, and so forth. Provide a sample item or two for your reader.
- If you have created a new instrument, you should attach it as an Appendix.
- If you presented participants with various word lists to remember or stimuli to judge, you should describe those in detail here. Use subheadings to separate different types of stimuli if needed. If you are only describing questionnaires, you may call this section “Measures.”
Apparatus (labeled, flush left, bold)
Include an apparatus section if you used specialized equipment for your study (e.g., the eye tracking machine) and need to describe it in detail.
Procedure (labeled, flush left, bold)
What did participants do, and in what order? When you list a control variable (e.g., “Participants all sat two feet from the experimenter.”), explain WHY you did what you did. In other words, what nuisance variable were you controlling for? Your procedure should be as brief and concise as possible. Read through it. Did you repeat yourself anywhere? If so, how can you rearrange things to avoid redundancy? You may either write the instructions to the participants verbatim or paraphrase, whichever you deem more appropriate. Don’t forget to include brief statements about informed consent and debriefing.
Results (labeled, centered, bold)
In this section, describe how you analyzed the data and what you found. If your data analyses were complex, feel free to break this section down into labeled subsections, perhaps one section for each hypothesis.
- Include a section for descriptive statistics
- List what type of analysis or test you conducted to test each hypothesis.
- Refer to your Statistics textbook for the proper way to report results in APA style. A t-test, for example, is reported in the following format: t (18) = 3.57, p < .001, where 18 is the number of degrees of freedom (N – 2 for an independent-groups t test). For a correlation: r (32) = -.52, p < .001, where 32 is the number of degrees of freedom (N – 2 for a correlation). For a one-way ANOVA: F (2, 18) = 7.00, p < .001, where 2 represents the between and 18 represents df within Remember that if a finding has a p value greater than .05, it is “nonsignificant,” not “insignificant.” For nonsignificant findings, still provide the exact p values. For correlations, be sure to report the r 2 value as an assessment of the strength of the finding, to show what proportion of variability is shared by the two variables you’re correlating. For t- tests and ANOVAs, report eta 2 .
- Report exact p values to two or three decimal places (e.g., p = .042; see p. 114 of APA manual). However, for p-values less than .001, simply put p < .001.
- Following the presentation of all the statistics and numbers, be sure to state the nature of your finding(s) in words and whether or not they support your hypothesis (e.g., “As predicted …”). This information can typically be presented in a sentence or two following the numbers (within the same paragraph). Also, be sure to include the relevant means and SDs.
- It may be useful to include a table or figure to represent your results visually. Be sure to refer to these in your paper (e.g., “As illustrated in Figure 1…”). Remember that you may present a set of findings either as a table or as a figure, but not as both. Make sure that your text is not redundant with your tables/figures. For instance, if you present a table of means and standard deviations, you do not need to also report these in the text. However, if you use a figure to represent your results, you may wish to report means and standard deviations in the text, as these may not always be precisely ascertained by examining the figure. Do describe the trends shown in the figure.
- Do not spend any time interpreting or explaining the results; save that for the Discussion section.
Discussion (labeled, centered, bold)
The goal of the discussion section is to interpret your findings and place them in the broader context of the literature in the area. A discussion section is like the reverse of the introduction, in that you begin with the specifics and work toward the more general (funnel out). Some points to consider:
- Begin with a brief restatement of your main findings (using words, not numbers). Did they support the hypothesis or not? If not, why not, do you think? Were there any surprising or interesting findings? How do your findings tie into the existing literature on the topic, or extend previous research? What do the results say about the broader behavior under investigation? Bring back some of the literature you discussed in the Introduction, and show how your results fit in (or don’t fit in, as the case may be). If you have surprising findings, you might discuss other theories that can help to explain the findings. Begin with the assumption that your results are valid, and explain why they might differ from others in the literature.
- What are the limitations of the study? If your findings differ from those of other researchers, or if you did not get statistically significant results, don’t spend pages and pages detailing what might have gone wrong with your study, but do provide one or two suggestions. Perhaps these could be incorporated into the future research section, below.
- What additional questions were generated from this study? What further research should be conducted on the topic? What gaps are there in the current body of research? Whenever you present an idea for a future research study, be sure to explain why you think that particular study should be conducted. What new knowledge would be gained from it? Don’t just say, “I think it would be interesting to re-run the study on a different college campus” or “It would be better to run the study again with more participants.” Really put some thought into what extensions of the research might be interesting/informative, and why.
- What are the theoretical and/or practical implications of your findings? How do these results relate to larger issues of human thoughts, feelings, and behavior? Give your readers “the big picture.” Try to answer the question, “So what?
Final paragraph: Be sure to sum up your paper with a final concluding statement. Don’t just trail off with an idea for a future study. End on a positive note by reminding your reader why your study was important and what it added to the literature.
References (labeled, centered, not bold)
Provide an alphabetical listing of the references (alphabetize by last name of first author). Double-space all, with no extra spaces between references. The second line of each reference should be indented (this is called a hanging indent and is easily accomplished using the ruler in Microsoft Word). See the APA manual for how to format references correctly.
Examples of references to journal articles start on p. 198 of the manual, and examples of references to books and book chapters start on pp. 202. Digital object identifiers (DOIs) are now included for electronic sources (see pp. 187-192 of APA manual to learn more).
Journal article example: [Note that only the first letter of the first word of the article title is capitalized; the journal name and volume are italicized. If the journal name had multiple words, each of the major words would be capitalized.]
Ebner-Priemer, U. W., & Trull, T. J. (2009). Ecological momentary assessment of mood disorders and mood dysregulation. Psychological Assessment, 21, 463-475. doi:10.1037/a0017075
Book chapter example: [Note that only the first letter of the first word of both the chapter title and book title are capitalized.]
Stephan, W. G. (1985). Intergroup relations. In G. Lindzey & E. Aronson (Eds.), The handbook of social psychology (3 rd ed., Vol. 2, pp. 599-658). New York: Random House.
Book example: Gray, P. (2010). Psychology (6 th ed.). New York: Worth
Table There are various formats for tables, depending upon the information you wish to include. See the APA manual. Be sure to provide a table number and table title (the latter is italicized). Tables can be single or double-spaced.
Figure If you have more than one figure, each one gets its own page. Use a sans serif font, such as Helvetica, for any text within your figure. Be sure to label your x- and y-axes clearly, and make sure you’ve noted the units of measurement of the DV. Underneath the figure provide a label and brief caption (e.g., “Figure 1. Mean evaluation of job applicant qualifications as a function of applicant attractiveness level”). The figure caption typically includes the IVs/predictor variables and the DV. Include error bars in your bar graphs, and note what the bars represent in the figure caption: Error bars represent one standard error above and below the mean.
In-Text Citations: (see pp. 174-179 of APA manual) When citing sources in your paper, you need to include the authors’ names and publication date.
You should use the following formats:
- When including the citation as part of the sentence, use AND: “According to Jones and Smith (2003), the…”
- When the citation appears in parentheses, use “&”: “Studies have shown that priming can affect actual motor behavior (Jones & Smith, 2003; Klein, Bailey, & Hammer, 1999).” The studies appearing in parentheses should be ordered alphabetically by the first author’s last name, and should be separated by semicolons.
- If you are quoting directly (which you should avoid), you also need to include the page number.
- For sources with three or more authors, once you have listed all the authors’ names, you may write “et al.” on subsequent mentions. For example: “Klein et al. (1999) found that….” For sources with two authors, both authors must be included every time the source is cited. When a source has six or more authors, the first author’s last name and “et al.” are used every time the source is cited (including the first time).
Secondary Sources
“Secondary source” is the term used to describe material that is cited in another source. If in his article entitled “Behavioral Study of Obedience” (1963), Stanley Milgram makes reference to the ideas of Snow (presented above), Snow (1961) is the primary source, and Milgram (1963) is the secondary source.
Try to avoid using secondary sources in your papers; in other words, try to find the primary source and read it before citing it in your own work. If you must use a secondary source, however, you should cite it in the following way:
Snow (as cited in Milgram, 1963) argued that, historically, the cause of most criminal acts... The reference for the Milgram article (but not the Snow reference) should then appear in the reference list at the end of your paper.
Tutor Appointments
Peer tutor and consultant appointments are managed through TracCloud (login required). Find resources and more information about the ALEX centers using the following links.
Office / Department Name
Nesbitt-Johnston Writing Center
Contact Name
Jennifer Ambrose
Writing Center Director

Help us provide an accessible education, offer innovative resources and programs, and foster intellectual exploration.
Site Search
Reference management. Clean and simple.
How to start your research paper [step-by-step guide]
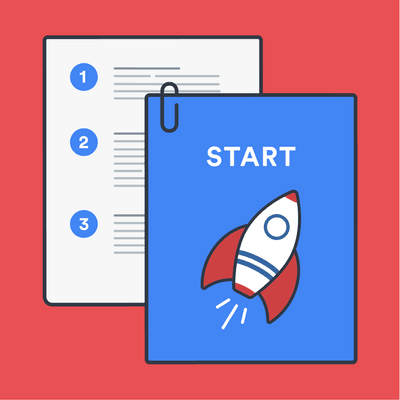
1. Choose your topic
2. find information on your topic, 3. create a thesis statement, 4. create a research paper outline, 5. organize your notes, 6. write your introduction, 7. write your first draft of the body, 9. write your conclusion, 10. revise again, edit, and proofread, frequently asked questions about starting your research paper, related articles.
Research papers can be short or in-depth, but no matter what type of research paper, they all follow pretty much the same pattern and have the same structure .
A research paper is a paper that makes an argument about a topic based on research and analysis.
There will be some basic differences, but if you can write one type of research paper, you can write another. Below is a step-by-step guide to starting and completing your research paper.
Choose a topic that interests you. Writing your research paper will be so much more pleasant with a topic that you actually want to know more about. Your interest will show in the way you write and effort you put into the paper. Consider these issues when coming up with a topic:
- make sure your topic is not too broad
- narrow it down if you're using terms that are too general
Academic search engines are a great source to find background information on your topic. Your institution's library will most likely provide access to plenty of online research databases. Take a look at our guide on how to efficiently search online databases for academic research to learn how to gather all the information needed on your topic.
Tip: If you’re struggling with finding research, consider meeting with an academic librarian to help you come up with more balanced keywords.
If you’re struggling to find a topic for your thesis, take a look at our guide on how to come up with a thesis topic .
The thesis statement is one of the most important elements of any piece of academic writing. It can be defined as a very brief statement of what the main point or central message of your paper is. Our thesis statement guide will help you write an excellent thesis statement.
In the next step, you need to create your research paper outline . The outline is the skeleton of your research paper. Simply start by writing down your thesis and the main ideas you wish to present. This will likely change as your research progresses; therefore, do not worry about being too specific in the early stages of writing your outline.
Then, fill out your outline with the following components:
- the main ideas that you want to cover in the paper
- the types of evidence that you will use to support your argument
- quotes from secondary sources that you may want to use
Organizing all the information you have gathered according to your outline will help you later on in the writing process. Analyze your notes, check for accuracy, verify the information, and make sure you understand all the information you have gathered in a way that you can communicate your findings effectively.
Start with the introduction. It will set the direction of your paper and help you a lot as you write. Waiting to write it at the end can leave you with a poorly written setup to an otherwise well-written paper.
The body of your paper argues, explains or describes your topic. Start with the first topic from your outline. Ideally, you have organized your notes in a way that you can work through your research paper outline and have all the notes ready.
After your first draft, take some time to check the paper for content errors. Rearrange ideas, make changes and check if the order of your paragraphs makes sense. At this point, it is helpful to re-read the research paper guidelines and make sure you have followed the format requirements. You can also use free grammar and proof reading checkers such as Grammarly .
Tip: Consider reading your paper from back to front when you undertake your initial revision. This will help you ensure that your argument and organization are sound.
Write your conclusion last and avoid including any new information that has not already been presented in the body of the paper. Your conclusion should wrap up your paper and show that your research question has been answered.
Allow a few days to pass after you finished writing the final draft of your research paper, and then start making your final corrections. The University of North Carolina at Chapel Hill gives some great advice here on how to revise, edit, and proofread your paper.
Tip: Take a break from your paper before you start your final revisions. Then, you’ll be able to approach your paper with fresh eyes.
As part of your final revision, be sure to check that you’ve cited everything correctly and that you have a full bibliography. Use a reference manager like Paperpile to organize your research and to create accurate citations.
The first step to start writing a research paper is to choose a topic. Make sure your topic is not too broad; narrow it down if you're using terms that are too general.
The format of your research paper will vary depending on the journal you submit to. Make sure to check first which citation style does the journal follow, in order to format your paper accordingly. Check Getting started with your research paper outline to have an idea of what a research paper looks like.
The last step of your research paper should be proofreading. Allow a few days to pass after you finished writing the final draft of your research paper, and then start making your final corrections. The University of North Carolina at Chapel Hill gives some great advice here on how to revise, edit and proofread your paper.
There are plenty of software you can use to write a research paper. We recommend our own citation software, Paperpile , as well as grammar and proof reading checkers such as Grammarly .
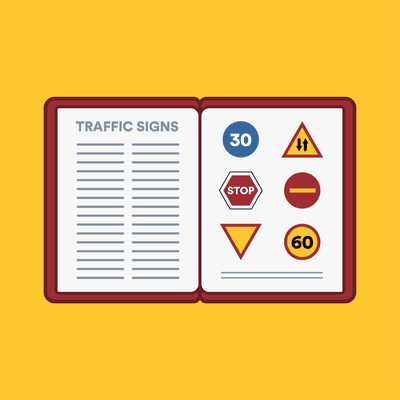
Purdue Online Writing Lab Purdue OWL® College of Liberal Arts
Writing a Research Paper
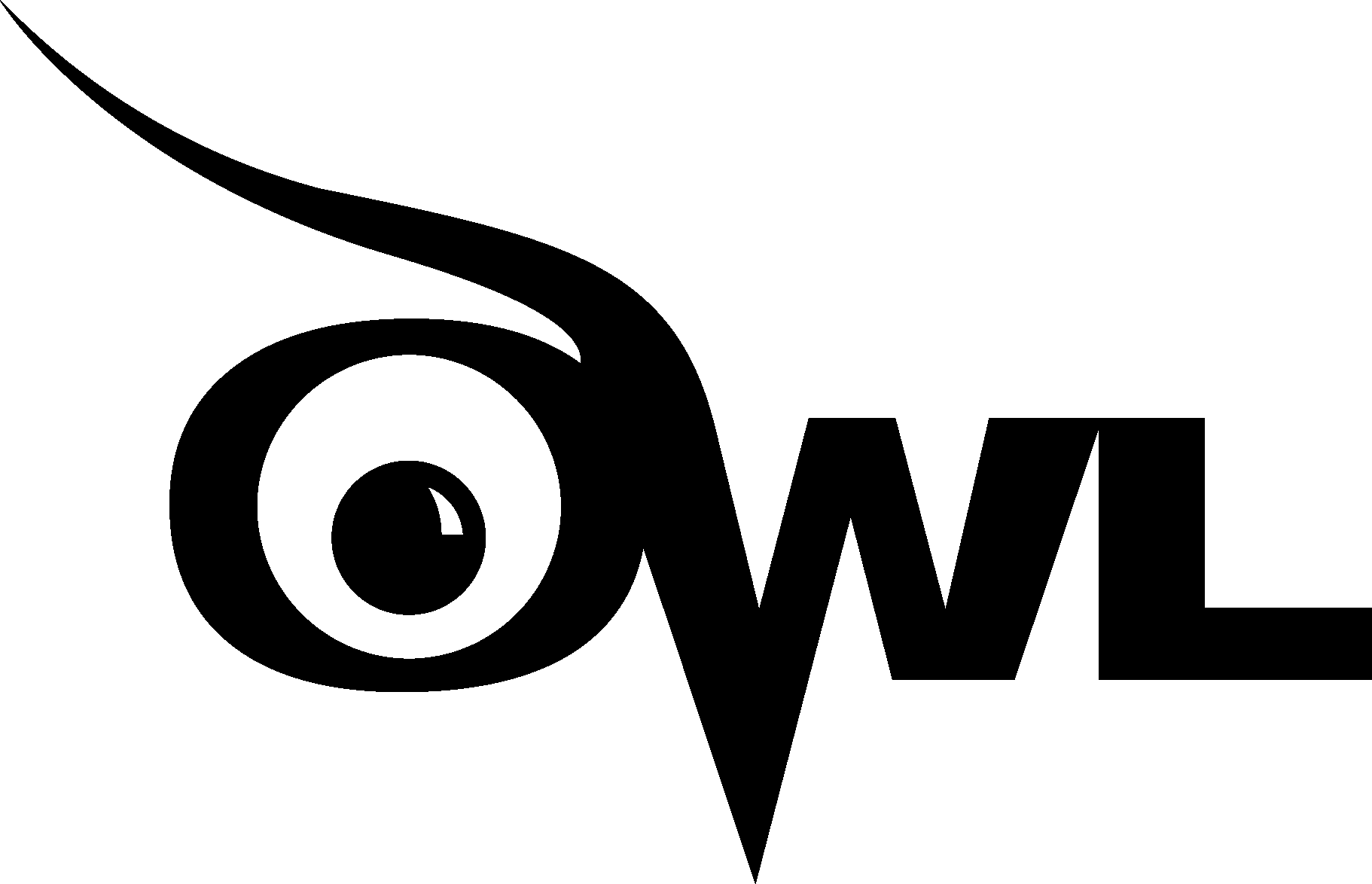
Welcome to the Purdue OWL
This page is brought to you by the OWL at Purdue University. When printing this page, you must include the entire legal notice.
Copyright ©1995-2018 by The Writing Lab & The OWL at Purdue and Purdue University. All rights reserved. This material may not be published, reproduced, broadcast, rewritten, or redistributed without permission. Use of this site constitutes acceptance of our terms and conditions of fair use.
The Research Paper
There will come a time in most students' careers when they are assigned a research paper. Such an assignment often creates a great deal of unneeded anxiety in the student, which may result in procrastination and a feeling of confusion and inadequacy. This anxiety frequently stems from the fact that many students are unfamiliar and inexperienced with this genre of writing. Never fear—inexperience and unfamiliarity are situations you can change through practice! Writing a research paper is an essential aspect of academics and should not be avoided on account of one's anxiety. In fact, the process of writing a research paper can be one of the more rewarding experiences one may encounter in academics. What is more, many students will continue to do research throughout their careers, which is one of the reasons this topic is so important.
Becoming an experienced researcher and writer in any field or discipline takes a great deal of practice. There are few individuals for whom this process comes naturally. Remember, even the most seasoned academic veterans have had to learn how to write a research paper at some point in their career. Therefore, with diligence, organization, practice, a willingness to learn (and to make mistakes!), and, perhaps most important of all, patience, students will find that they can achieve great things through their research and writing.
The pages in this section cover the following topic areas related to the process of writing a research paper:
- Genre - This section will provide an overview for understanding the difference between an analytical and argumentative research paper.
- Choosing a Topic - This section will guide the student through the process of choosing topics, whether the topic be one that is assigned or one that the student chooses themselves.
- Identifying an Audience - This section will help the student understand the often times confusing topic of audience by offering some basic guidelines for the process.
- Where Do I Begin - This section concludes the handout by offering several links to resources at Purdue, and also provides an overview of the final stages of writing a research paper.
Writing a Research Paper
This page lists some of the stages involved in writing a library-based research paper.
Although this list suggests that there is a simple, linear process to writing such a paper, the actual process of writing a research paper is often a messy and recursive one, so please use this outline as a flexible guide.
Discovering, Narrowing, and Focusing a Researchable Topic
- Try to find a topic that truly interests you
- Try writing your way to a topic
- Talk with your course instructor and classmates about your topic
- Pose your topic as a question to be answered or a problem to be solved
Finding, Selecting, and Reading Sources
You will need to look at the following types of sources:
- library catalog, periodical indexes, bibliographies, suggestions from your instructor
- primary vs. secondary sources
- journals, books, other documents

Grouping, Sequencing, and Documenting Information
The following systems will help keep you organized:
- a system for noting sources on bibliography cards
- a system for organizing material according to its relative importance
- a system for taking notes
Writing an Outline and a Prospectus for Yourself
Consider the following questions:
- What is the topic?
- Why is it significant?
- What background material is relevant?
- What is my thesis or purpose statement?
- What organizational plan will best support my purpose?
Writing the Introduction
In the introduction you will need to do the following things:
- present relevant background or contextual material
- define terms or concepts when necessary
- explain the focus of the paper and your specific purpose
- reveal your plan of organization
Writing the Body
- Use your outline and prospectus as flexible guides
- Build your essay around points you want to make (i.e., don’t let your sources organize your paper)
- Integrate your sources into your discussion
- Summarize, analyze, explain, and evaluate published work rather than merely reporting it
- Move up and down the “ladder of abstraction” from generalization to varying levels of detail back to generalization
Writing the Conclusion
- If the argument or point of your paper is complex, you may need to summarize the argument for your reader.
- If prior to your conclusion you have not yet explained the significance of your findings or if you are proceeding inductively, use the end of your paper to add your points up, to explain their significance.
- Move from a detailed to a general level of consideration that returns the topic to the context provided by the introduction.
- Perhaps suggest what about this topic needs further research.
Revising the Final Draft
- Check overall organization : logical flow of introduction, coherence and depth of discussion in body, effectiveness of conclusion.
- Paragraph level concerns : topic sentences, sequence of ideas within paragraphs, use of details to support generalizations, summary sentences where necessary, use of transitions within and between paragraphs.
- Sentence level concerns: sentence structure, word choices, punctuation, spelling.
- Documentation: consistent use of one system, citation of all material not considered common knowledge, appropriate use of endnotes or footnotes, accuracy of list of works cited.

Academic and Professional Writing
This is an accordion element with a series of buttons that open and close related content panels.
Analysis Papers
Reading Poetry
A Short Guide to Close Reading for Literary Analysis
Using Literary Quotations
Play Reviews
Writing a Rhetorical Précis to Analyze Nonfiction Texts
Incorporating Interview Data
Grant Proposals
Planning and Writing a Grant Proposal: The Basics
Additional Resources for Grants and Proposal Writing
Job Materials and Application Essays
Writing Personal Statements for Ph.D. Programs
- Before you begin: useful tips for writing your essay
- Guided brainstorming exercises
- Get more help with your essay
- Frequently Asked Questions
Resume Writing Tips
CV Writing Tips
Cover Letters
Business Letters
Proposals and Dissertations
Resources for Proposal Writers
Resources for Dissertators
Research Papers
Planning and Writing Research Papers
Quoting and Paraphrasing
Writing Annotated Bibliographies
Creating Poster Presentations
Writing an Abstract for Your Research Paper
Thank-You Notes
Advice for Students Writing Thank-You Notes to Donors
Reading for a Review
Critical Reviews
Writing a Review of Literature
Scientific Reports
Scientific Report Format
Sample Lab Assignment
Writing for the Web
Writing an Effective Blog Post
Writing for Social Media: A Guide for Academics
Canvas | University | Ask a Librarian
- Library Homepage
- Arrendale Library
Writing a Research Paper
Types of research papers.
- About This Guide
- Choosing a Topic
- Writing a Thesis Statement
- Gathering Research
- Journals and Magazines This link opens in a new window
- Creating an Outline
- Writing Your Paper
- Citing Resources
- Academic Integrity This link opens in a new window
- Contact Us!
Call us at 706-776-0111
Chat with a Librarian
Send Us Email
Library Hours
Although research paper assignments may vary widely, there are essentially two basic types of research papers. These are argumentative and analytical .
Argumentative
In an argumentative research paper, a student both states the topic they will be exploring and immediately establishes the position they will argue regarding that topic in a thesis statement . This type of paper hopes to persuade its reader to adopt the view presented.
Example : a paper that argues the merits of early exposure to reading for children would be an argumentative essay.
An analytical research paper states the topic that the writer will be exploring, usually in the form of a question, initially taking a neutral stance. The body of the paper will present multifaceted information and, ultimately, the writer will state their conclusion, based on the information that has unfolded throughout the course of the essay. This type of paper hopes to offer a well-supported critical analysis without necessarily persuading the reader to any particular way of thinking.
Example : a paper that explores the use of metaphor in one of Shakespeare's sonnets would be an example of an analytical essay.
*Please note that this LibGuide will primarily be concerning itself with argumentative or rhetorical research papers.
- << Previous: About This Guide
- Next: Choosing a Topic >>
- Last Updated: May 16, 2024 10:46 AM
- URL: https://library.piedmont.edu/research_paper
- Ebooks & Online Video
- New Materials
- Renew Checkouts
- Faculty Resources
- Library Friends
- Library Services
- Our Mission
- Library History
- Ask a Librarian!
- Making Citations
- Working Online

Arrendale Library Piedmont University 706-776-0111

Paper Format
Consistency in the order, structure, and format of a paper allows readers to focus on a paper’s content rather than its presentation.
To format a paper in APA Style, writers can typically use the default settings and automatic formatting tools of their word-processing program or make only minor adjustments.
The guidelines for paper format apply to both student assignments and manuscripts being submitted for publication to a journal. If you are using APA Style to create another kind of work (e.g., a website, conference poster, or PowerPoint presentation), you may need to format your work differently in order to optimize its presentation, for example, by using different line spacing and font sizes. Follow the guidelines of your institution or publisher to adapt APA Style formatting guidelines as needed.
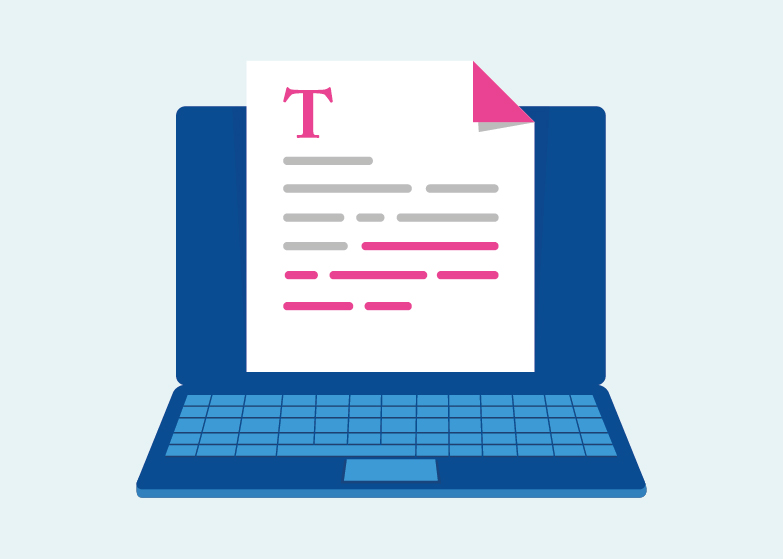
Academic Writer ®
Master academic writing with APA’s essential teaching and learning resource
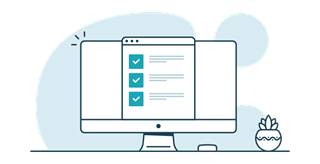
Course Adoption
Teaching APA Style? Become a course adopter of the 7th edition Publication Manual
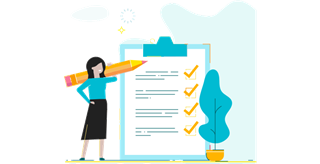
Instructional Aids
Guides, checklists, webinars, tutorials, and sample papers for anyone looking to improve their knowledge of APA Style
Have a language expert improve your writing
Run a free plagiarism check in 10 minutes, generate accurate citations for free.
- Knowledge Base
- Research paper
How to Create a Structured Research Paper Outline | Example
Published on August 7, 2022 by Courtney Gahan . Revised on August 15, 2023.
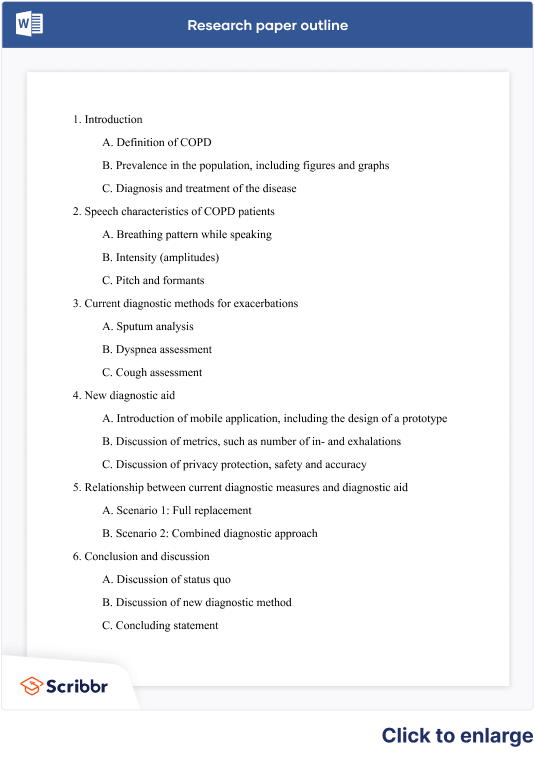
A research paper outline is a useful tool to aid in the writing process , providing a structure to follow with all information to be included in the paper clearly organized.
A quality outline can make writing your research paper more efficient by helping to:
- Organize your thoughts
- Understand the flow of information and how ideas are related
- Ensure nothing is forgotten
A research paper outline can also give your teacher an early idea of the final product.
Instantly correct all language mistakes in your text
Upload your document to correct all your mistakes in minutes
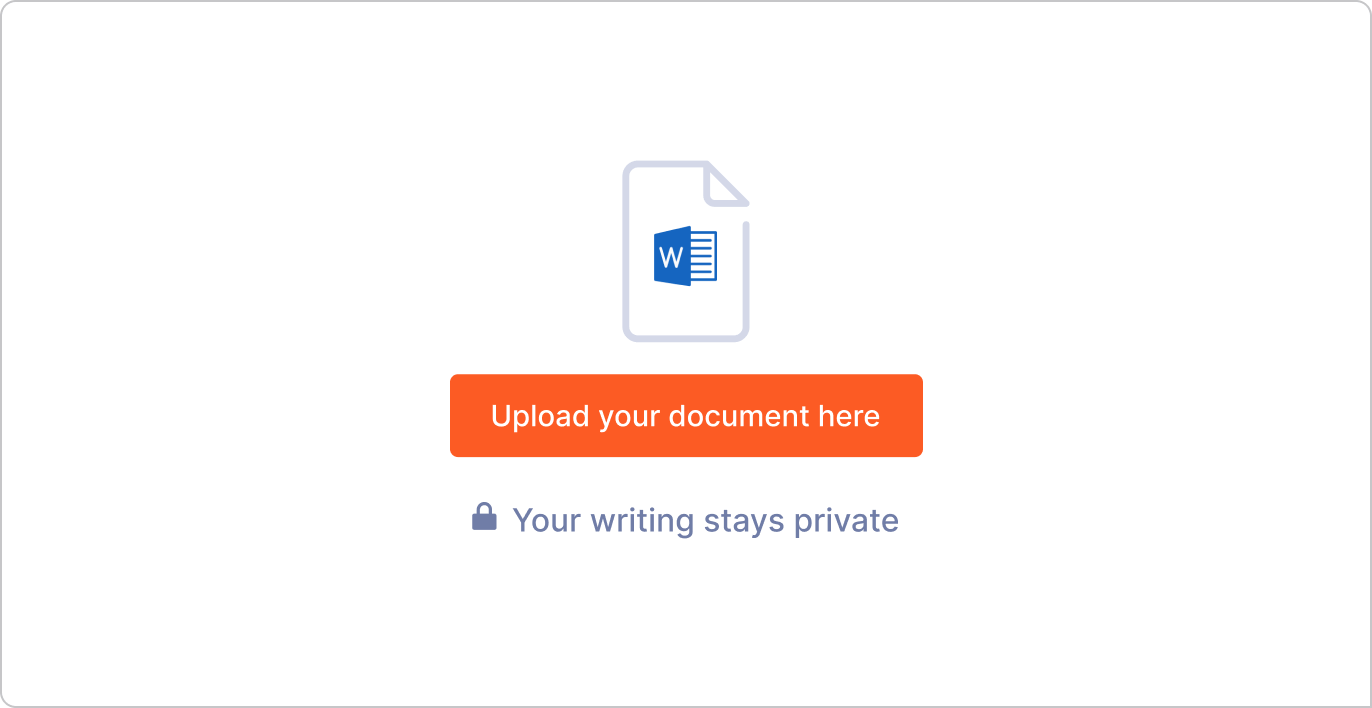
Table of contents
Research paper outline example, how to write a research paper outline, formatting your research paper outline, language in research paper outlines.
- Definition of measles
- Rise in cases in recent years in places the disease was previously eliminated or had very low rates of infection
- Figures: Number of cases per year on average, number in recent years. Relate to immunization
- Symptoms and timeframes of disease
- Risk of fatality, including statistics
- How measles is spread
- Immunization procedures in different regions
- Different regions, focusing on the arguments from those against immunization
- Immunization figures in affected regions
- High number of cases in non-immunizing regions
- Illnesses that can result from measles virus
- Fatal cases of other illnesses after patient contracted measles
- Summary of arguments of different groups
- Summary of figures and relationship with recent immunization debate
- Which side of the argument appears to be correct?
Receive feedback on language, structure, and formatting
Professional editors proofread and edit your paper by focusing on:
- Academic style
- Vague sentences
- Style consistency
See an example
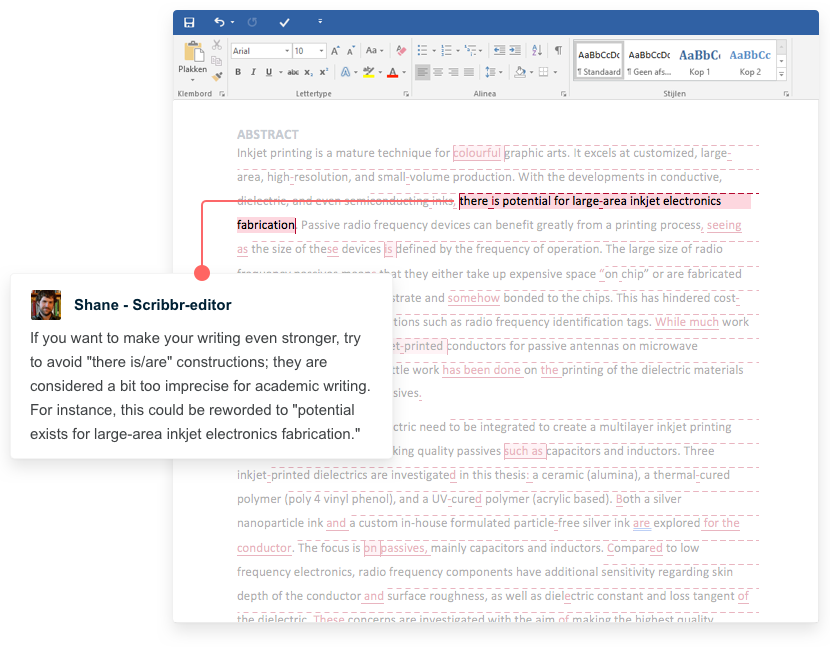
Follow these steps to start your research paper outline:
- Decide on the subject of the paper
- Write down all the ideas you want to include or discuss
- Organize related ideas into sub-groups
- Arrange your ideas into a hierarchy: What should the reader learn first? What is most important? Which idea will help end your paper most effectively?
- Create headings and subheadings that are effective
- Format the outline in either alphanumeric, full-sentence or decimal format
There are three different kinds of research paper outline: alphanumeric, full-sentence and decimal outlines. The differences relate to formatting and style of writing.
- Alphanumeric
- Full-sentence
An alphanumeric outline is most commonly used. It uses Roman numerals, capitalized letters, arabic numerals, lowercase letters to organize the flow of information. Text is written with short notes rather than full sentences.
- Sub-point of sub-point 1
Essentially the same as the alphanumeric outline, but with the text written in full sentences rather than short points.
- Additional sub-point to conclude discussion of point of evidence introduced in point A
A decimal outline is similar in format to the alphanumeric outline, but with a different numbering system: 1, 1.1, 1.2, etc. Text is written as short notes rather than full sentences.
- 1.1.1 Sub-point of first point
- 1.1.2 Sub-point of first point
- 1.2 Second point
To write an effective research paper outline, it is important to pay attention to language. This is especially important if it is one you will show to your teacher or be assessed on.
There are four main considerations: parallelism, coordination, subordination and division.
Parallelism: Be consistent with grammatical form
Parallel structure or parallelism is the repetition of a particular grammatical form within a sentence, or in this case, between points and sub-points. This simply means that if the first point is a verb , the sub-point should also be a verb.
Example of parallelism:
- Include different regions, focusing on the different arguments from those against immunization
Coordination: Be aware of each point’s weight
Your chosen subheadings should hold the same significance as each other, as should all first sub-points, secondary sub-points, and so on.
Example of coordination:
- Include immunization figures in affected regions
- Illnesses that can result from the measles virus
Subordination: Work from general to specific
Subordination refers to the separation of general points from specific. Your main headings should be quite general, and each level of sub-point should become more specific.
Example of subordination:
Division: break information into sub-points.
Your headings should be divided into two or more subsections. There is no limit to how many subsections you can include under each heading, but keep in mind that the information will be structured into a paragraph during the writing stage, so you should not go overboard with the number of sub-points.
Ready to start writing or looking for guidance on a different step in the process? Read our step-by-step guide on how to write a research paper .
Cite this Scribbr article
If you want to cite this source, you can copy and paste the citation or click the “Cite this Scribbr article” button to automatically add the citation to our free Citation Generator.
Gahan, C. (2023, August 15). How to Create a Structured Research Paper Outline | Example. Scribbr. Retrieved September 18, 2024, from https://www.scribbr.com/research-paper/outline/
Is this article helpful?
Courtney Gahan
Other students also liked, research paper format | apa, mla, & chicago templates, writing a research paper introduction | step-by-step guide, writing a research paper conclusion | step-by-step guide, get unlimited documents corrected.
✔ Free APA citation check included ✔ Unlimited document corrections ✔ Specialized in correcting academic texts
A systematic review of aspect-based sentiment analysis: domains, methods, and trends
- Open access
- Published: 17 September 2024
- Volume 57 , article number 296 , ( 2024 )
Cite this article
You have full access to this open access article
- Yan Cathy Hua ORCID: orcid.org/0000-0001-9155-9667 1 ,
- Paul Denny ORCID: orcid.org/0000-0002-5150-9806 1 ,
- Jörg Wicker ORCID: orcid.org/0000-0003-0533-3368 1 &
- Katerina Taskova ORCID: orcid.org/0000-0002-3217-7877 1
Aspect-based sentiment analysis (ABSA) is a fine-grained type of sentiment analysis that identifies aspects and their associated opinions from a given text. With the surge of digital opinionated text data, ABSA gained increasing popularity for its ability to mine more detailed and targeted insights. Many review papers on ABSA subtasks and solution methodologies exist, however, few focus on trends over time or systemic issues relating to research application domains, datasets, and solution approaches. To fill the gap, this paper presents a systematic literature review (SLR) of ABSA studies with a focus on trends and high-level relationships among these fundamental components. This review is one of the largest SLRs on ABSA. To our knowledge, it is also the first to systematically examine the interrelations among ABSA research and data distribution across domains, as well as trends in solution paradigms and approaches. Our sample includes 727 primary studies screened from 8550 search results without time constraints via an innovative automatic filtering process. Our quantitative analysis not only identifies trends in nearly two decades of ABSA research development but also unveils a systemic lack of dataset and domain diversity as well as domain mismatch that may hinder the development of future ABSA research. We discuss these findings and their implications and propose suggestions for future research.
Explore related subjects
- Artificial Intelligence
Avoid common mistakes on your manuscript.
1 Introduction
In the digital era, a vast amount of online opinionated text is generated daily through which people express views and feelings (i.e. sentiment) towards certain subjects, such as user reviews, social media posts, and open-ended survey question responses (Kumar and Gupta 2021 ). Understanding the sentiment of these opinionated text data is essential for gaining insights into people’s preferences and behaviours and supporting decision-making across a wide variety of domains (Sharma and Shekhar 2020 ; Wankhade et al 2022 ; Tubishat et al 2021 ; García-Pablos et al 2018 ; Poria et al 2016 ). The analyses of opinionated text usually aim at answering questions such as “ What subjects were mentioned? ”, “ What did people think of (a specific subject)? ”, and “ How are the subjects and/or opinions distributed across the sample? ” (e.g. (Dragoni et al 2019 ; Krishnakumari and Sivasankar 2018 ; Fukumoto et al 2016 ; Zarindast et al 2021 )). These objectives, along with today’s enormous volume of digital opinionated text, require an automated solution for identifying, extracting and classifying the subjects and their associated opinions from the raw text. Aspect-based sentiment analysis (ABSA) is one such solution.
1.1 Review focus and research questions
This work presents a systematic literature review (SLR) of existing ABSA studies with a large-scale sample and quantitative results. We focus on trends and high-level patterns instead of methodological details that were well covered by the existing surveys mentioned above. We aim to benefit both ABSA newcomers by introducing the basics of the topic, as well as existing ABSA researchers by sharing perspectives and findings that are useful to the ABSA community and can only be obtained beyond the immediate research tasks and technicalities.
We seek to answer the following sets of research questions (RQs):
RQ1. To what extent is ABSA research and its dataset resources dominated by the commercial (especially the product and service review) domain? What proportion of ABSA research focuses on other domains and dataset resources?
RQ2. What are the most common ABSA problem formulations via subtask combinations, and what proportion of ABSA studies only focus on a specific subtask?
RQ3. What is the trend in the ABSA solution approaches over time? Are linguistic and traditional machine-learning approaches still in use?
This review makes a number of unique contributions to the ABSA research field: (1) It is one of the largest scoped SLRs on ABSA, with a main review and a Phase-2 targeted review of a combined 727 primary studies published in 2008–2024, selected from 8550 search results without time constraint. (2) To our knowledge, it is the first SLR that systematically examines the ABSA data resource distribution in relation to research application domains and methodologies; and (3) Our review methodology adopted an innovative automatic filtering process based on PDF-mining, which enhanced screening quality and reliability. Our quantitative results not only revealed trends in nearly two decades of ABSA research literature but also highlighted potential systemic issues that could limit the development of future ABSA research.
1.2 Organisation of this review
In Sect. 2 (“Background”), we introduce ABSA and highlight the motivation and uniqueness of this review. Section 3 (“Methods”) outlines our SLR procedures, and Sect. 4 (“Results”) answers the research questions with the SLR results. We then discuss the key findings and acknowledge limitations in Sects. 5 and 6 (“Discussion” and “Conclusion”).
For those interested in more details, Appendix A provides an in-depth introduction to ABSA and its subtasks. Appendix B describes the full details of our Methods, and additional figures from the Results are provided in Appendix C .
2 Background
2.1 absa: a fine-grained sentiment analysis.
Aspect-based sentiment analysis (ABSA) is a sub-field of Sentiment Analysis (SA), which is a core task of natural language processing (NLP). SA, also known as “opinion mining” (García-Pablos et al 2018 ; Poria et al 2016 ; Liang et al 2022 ; López and Arco 2019 ; Tran et al 2020 ), solves the problem of identifying and classifying given text corpora’s affect or sentiment orientation (Akhtar et al 2020 ; Tubishat et al 2021 ) into polarity categories (e.g. “positive, neutral, negative”) (Brauwers and Frasincar 2023 ; Hu and Liu 2004a ), intensity/strength scores (e.g. from 1 to 5) (Wang et al 2017 ), or other categories. The “ identifying the subjects of opinions ” part of the quest relates to the granularity of SA. Traditional SA mostly focuses on document- or sentence-level sentiment and thus assumes a single subject of opinions (Nazir et al 2022b ; Liu et al 2020 ). In recent decades, the explosion of online opinion text has attracted increasing interest in distilling more targeted insights on specific entities or their aspects within each sentence through finer-grained SA (Nazir et al 2022b ; Liu et al 2020 ; Akhtar et al 2020 ; You et al 2022 ; Ettaleb et al 2022 ). This is the problem ABSA aims to solve.
2.2 ABSA and its subtasks
ABSA involves identifying the sentiments toward specific entities or their attributes, called aspects . These aspects can be explicitly mentioned in the text or implied from the context (“implicit aspects”), and can be grouped into aspect categories (Nazir et al 2022a ; Akhtar et al 2020 ; Maitama et al 2020 ; Xu et al 2020b ; Chauhan et al 2019 ; Akhtar et al 2018 ). Appendix A.1 presents a more detailed definition of ABSA, including its key components and examples.
A complete ABSA solution as described above traditionally involves a combination of subtasks, with the fundamental ones (Li et al 2022a ; Huan et al 2022 ; Li et al 2020 ; Fei et al 2023b ; Pathan and Prakash 2022 ) being Aspect (term) Extraction (AE), Opinion (term) Extraction (OE), and Aspect-Sentiment Classification (ASC), or in an aggregated form via Aspect-Category Detection (ACD) and Aspect Category Sentiment Analysis (ACSA).
The choice of subtasks in an ABSA solution reflects both the problem formulation and, to a large extent, the technologies and resources available at the time. The solutions to these fundamental ABSA subtasks evolved from pure linguistic and statistical solutions to the dominant machine learning (ML) approaches (Maitama et al 2020 ; Cortis and Davis 2021 ; Liu et al 2020 ; Federici and Dragoni 2016 ), usually with multiple subtask models or modules orchestrated in a pipeline (Li et al 2022b ; Nazir and Rao 2022 ). More recently, the rise of multi-task learning brought an increase in End-to-end (E2E) ABSA solutions that can better capture the inter-task relations via shared learning (Liu et al 2024 ), and many only involve a single model that provides the full ABSA solution via one composite task (Huan et al 2022 ; Li et al 2022b ; Zhang et al 2022b ). The most typical composite ABSA tasks include Aspect-Opinion Pair Extraction (AOPE) (Nazir and Rao 2022 ; Li et al 2022c ; Wu et al 2021 ), Aspect-Polarity Co-Extraction (APCE) (Huan et al 2022 ; He et al 2019 ), Aspect-Sentiment Triplet Extraction (ASTE) (Huan et al 2022 ; Li et al 2022b ; Du et al 2021 ; Fei et al 2023b ), and Aspect-Sentiment Quadruplet Extraction/Prediction (ASQE/ASQP) (Zhang et al 2022a ; Lim and Buntine 2014 ; Zhang et al 2021a , 2024a ). We provide a more detailed introduction to ABSA subtasks in Appendix A.2 .
2.3 The context- and domain-dependency challenges
The nature and the interconnection of its components and subtasks determine that ABSA is heavily domain- and context-dependent (Nazir et al 2022b ; Chebolu et al 2023 ; Howard et al 2022 ). Domain refers to the ABSA task (training or application) topic domains, and context can be either the “global” context of the document or the “local” context from the text surrounding a target word token or word chunks. At least in English, the same word or phrase could mean different things or bear different sentiments depending on the context and topic domains. For example, “a big fan” could be an electric appliance or a person, depending on the sentence and the domain; “cold” could be positive for ice cream but negative for customer service; and “DPS” (damage per second) could be either a gaming aspect or non-aspect in other domains. Thus, the ability to incorporate relevant context is essential for ABSA solutions; and those with zero or very small context windows, such as n-gram and Markov models, are rare in ABSA literature and can only tackle a limited range of subtasks (e.g. Presannakumar and Mohamed 2021 ).
Moreover, although many language models (e.g. Bidirectional Encoder Representations from Transformers (BERT, Devlin et al 2019 ), Generative pre-trained transformers (GPT, Brown et al 2020 ), recurrent neural network (RNN)-based models) already incorporated local context from the input-sequence and/or general context through pre-trained embeddings, they still performed unsatisfactorily on some ABSA domains and subtasks, especially Implicit AE (IAE), AE with multi-word aspects, AE and ACD on mixed-domain corpora, and context-dependent ASC (Phan and Ogunbona 2020 ; You et al 2022 ; Liang et al 2022 ; Howard et al 2022 ). Many studies showed that ABSA task performance benefits from expanding the feature space beyond the generic and input textual context. This includes incorporating domain-specific dataset/representations and additional input features such as Part-of-Speech (POS) tags, syntactic dependency relations, lexical databases, and domain knowledge graphs or ontologies (Howard et al 2022 ; You et al 2022 ; Liang et al 2022 ). Nonetheless, annotated datasets and domain-specific resources are costly to produce and limited in availability, and domain adaptation, as one solution to this, has been an ongoing challenge for ABSA (Chen and Qian 2022 ; Zhang et al 2022b ; Nazir et al 2022b ; Howard et al 2022 ; Satyarthi and Sharma 2023 ).
The above highlights the critical role of domain-specific datasets and resources in ABSA solution quality, especially for supervised approaches. On the other hand, it suggests the possibility that the prevalence of dataset-reliant solutions in the field, and a skewed ABSA dataset domain distribution, could systemically hinder ABSA solution performance and generalisability (Chen and Qian 2022 ; Fei et al 2023a ), thus confining ABSA research and solutions close to the resource-rich domains and languages. This idea underpins this literature review’s motivation and research questions.
2.4 Review rationale
This review is motivated by the following rationales:
First, the shift towards ML, especially supervised and/or DL solutions for ABSA, highlights the importance of dataset resources. In particular, annotated large benchmark datasets are crucial for the quality and development of ABSA research. Meanwhile, the finer granularity of ABSA also brings the persistent challenge of domain dependency described in Sect. 2.3 . The diversity of ABSA datasets and their domains can have a direct and systematic impact on research and applications.
The early seminal works in ABSA were motivated by commercial applications and focused on product and service reviews (Liu et al 2020 ; Rana and Cheah 2016 ; Do et al 2019 ), such as Ganu et al ( 2009 ), Hu and Liu ( 2004b ), and Pontiki et al ( 2014 , 2015 , 2016 ) that laid influential foundations with widely-used product and service review ABSA benchmark datasets (Rana and Cheah 2016 ; Do et al 2019 ). Nevertheless, the need for mining insights from opinions far exceeds this single domain. Many other areas, especially the public sector, also have an abundance of opinionated text data and can benefit from ABSA, such as helping policy-makers understand public attitudes and reactions towards events or changes (Sharma and Shekhar 2020 ), improving healthcare services and treatments via patient experience and concerns in clinical visits, symptoms, drug efficacy and side-effects (Cavalcanti and Prudêncio 2017 ; Gui and He 2021 ), and guiding educators in meeting teacher and learner needs and improving their experience (Wankhade et al 2022 ; Tubishat et al 2021 ; García-Pablos et al 2018 ; Poria et al 2016 ). While the more general SA research has been applied to “nearly every domain” (Nazir et al 2022b , p. 1), this does not seem to be the case for ABSA. Chebolu et al ( 2023 ) reviewed 62 public ABSA datasets released between 2004 and 2020 covering “over 25 domains” (Chebolu et al 2023 , p. 1). However, 53 out of these 62 datasets were reviews of restaurants, hotels, and digital products; only five were not related to commercial products or services, and merely one was on the public sector domain (university reviews).
The above-mentioned evidence raises questions: Will this dataset domain homogeneity be found with a larger sample of primary studies? Does this domain skewness reflect the concentration of ABSA research focus or merely the lack of dataset diversity? This motivated our RQ1 (“ To what extent is ABSA research and its dataset resources dominated by the commercial (especially the product and service review) domain? What proportion of ABSA research focuses on other domains and dataset resources? ”) Answers to these questions could inform and shape future ABSA research through individual research decisions and community resource collaboration.
Second, there are many good survey papers on ABSA, most focused on introducing the methodological details of common ABSA subtasks and solutions (e.g. Maitama et al 2020 ; Sabeeh and Dewang 2019 ; Rana and Cheah 2016 ; Soni and Rambola 2022 ; Ganganwar and Rajalakshmi 2019 ; Zhou et al 2019 ) or specific approaches such as DL methods for ABSA (e.g. Liu et al 2020 ; Do et al 2019 ; Wang et al 2021a ; Chen and Fnu 2022 ; Mughal et al 2024 ; Zhang et al 2022c ; Satyarthi and Sharma 2023 ). We list these surveys in Appendix A.3 as additional resources for the reader. Nonetheless, many of these reviews only explored each subtask and/or technique individually and often by iterating through reviewed studies, and few examined their combinations or changes over time and with quantitative evidence. For example, although the above-listed reviews (Liu et al 2020 ; Do et al 2019 ; Wang et al 2021a ; Chen and Fnu 2022 ) reported the rise of DL approaches in ABSA similar to that of NLP as a whole, it is unclear whether ABSA research was also increasingly dominated by the attention mechanism from the Transformer architecture (Vaswani et al 2017 ) and pre-trained large language models since 2018 (Manning 2022 ), and if linguistic and traditional ML approaches were still active. In addition, most of these surveys used a smaller and selected sample that could not support conclusions on trends. As the field matures, we believe it is necessary and important to examine trends and matters outside the problem solution itself, so as to inform research decisions, identify issues, and call for necessary community awareness and actions. We thus proposed RQ2 (“ What are the most common ABSA problem formulations via subtask combinations, and what proportion of ABSA studies only focus on a specific sub-task? ”) and RQ3 (“ What is the trend in the ABSA solution approaches over time? Are linguistic and traditional machine-learning approaches still in use? ”).
In order to identify patterns and trends for our RQs, a sufficiently sized representative sample and systematic approach are required. We chose to conduct an SLR, as this type of review aims to answer specific research questions from all available primary research evidence following well-defined review protocols (Kitchenham and Charters 2007 ). Moreover, none of the existing SLRs on ABSA share the same focus and RQs as ours: Among the 192 survey/review papers obtained from four major digital database searches detailed in Sect. 3 , only eight were SLRs on ABSA, within which four focused on non-English language(s) (Alyami et al 2022 ; Obiedat et al 2021 ; Hoti et al 2022 ; Rani and Kumar 2019 ), two on specific domains (software development, social media) (Cortis and Davis 2021 ; Lin et al 2022 ), one on a single subtask (Maitama et al 2020 ), and one mentioned ABSA subtasks as a side-note under the main topic of SA (Ligthart et al 2021 ).
In summary, this review aims to address gaps in the ABSA literature. The high-level nature of our research questions is best answered through a large-scale SLR to provide solid evidence. The next section presents our SLR approach and sample.
Following the guidance of Kitchenham and Charters ( 2007 ), we conducted this SLR with pre-planned scope, criteria, and procedures highlighted below. The complete SLR methods and process are detailed in Appendix B .
3.1 Main procedures
For the main SLR sample, we sourced the primary studies in October 2022 from four major peer-reviewed digital databases: ACM Digital Library, IEEE Xplore, Science Direct, and SpringerLink. First, we manually searched and extracted 4191 database results without publication-year constraints. Appendix B.1 provides more details of the search strategies and results. Next, we applied the inclusion and exclusion criteria listed in Table 1 via automatic Footnote 1 and manual screening steps and identified 519 in-scope peer-reviewed research publications for the review. The complete screening process, including that of the automatic screening, is described in Appendix B.2 . We then manually reviewed the in-scope primary studies and recorded data following a planned scheme. Lastly, we checked, cleaned, and processed the extracted data and performed quantitative analysis against our RQs.
3.2 Main SLR sample summary
Figure 1 shows the number of total reviewed vs. included studies across all publication years for the 4191 SLR search results. The search results include studies published between 1995 and 2023 ( \(\textrm{N}=1\) ), although all of the pre-2008 ones (2 from the 90s, 8 from 2003–2006, 17 from 2007) were not ABSA-focused and were excluded during automatic screening. The earliest in-scope ABSA study in the sample was published in 2008, followed by a very sparse period until 2013. The numbers of extracted and in-scope publications have both grown noticeably since 2014, a likely result of the emergence of deep learning approaches, especially sequence models such as RNNs (Manning 2022 ; Sutskever et al 2014 ). We also present a breakdown of the included studies by publication year and type in Figure 9 in Appendix C .
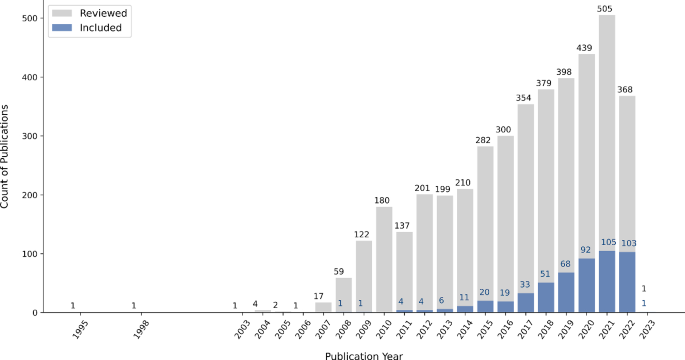
Number of studies by publication year: total reviewed ( \(\textrm{N}=4191\) ) vs. included ( \(\textrm{N}=519\) )
3.3 Note on “domain” mapping
In order to answer RQ1, we made the distinction between “research application domain” (“research domain” in short) and “dataset domain”, and manually examined and classified each study and its datasets into domain categories.
We considered each study’s research domain to be “non-specific” unless the study mentioned a specific application domain or use case as its motivation. For the dataset domain, we examined each dataset used by our sample, standardised its name, and recorded the domain from which it was drawn/selected based on the description provided by the author or the dataset source webpage. Datasets without a specific domain (e.g. Twitter tweets crawled without a specific domain filter) were labelled as “non-specific”.
We then manually grouped the research and dataset domains into 19 common categories used for analysis. More details and examples on domain mapping are available in Appendix B.3 .
3.4 Phase-2 targeted review on in-context learning
Additionally, generative “foundation models” (Bommasani et al 2022 ), defined as models with billions of parameters pre-trained on enormous general-purpose data and adaptable to diverse downstream NLP tasks, have become ubiquitous after our SLR data collection (e.g. ChatGPT OpenAI 2023 , released in November 2022). We use the term “foundation models” to distinguish them from the earlier pre-trained Large Language Models (LLMs) such as BERT (Devlin et al 2019 ), BART (Lewis et al 2020 ), and T5 (Raffel et al 2020 ), which have relatively fewer parameters and typically require fine-tuning for task adaptation (Zhang et al 2022c ). These generative foundation models brought a new paradigm of “In-context Learning” (ICL) (Brown et al 2020 , p. 4), where task adaptation can occur solely via conditioning the model on the text input instructions (“prompts”) with zero (“zero-shot ICL”) or few (“few-shot ICL”) examples and no model parameter changes (Brown et al 2020 ; Dong et al 2024 ). To capture and analyse this new development while balancing feasibility and currency, we conducted a Phase-2 targeted review in July 2024.
This Phase-2 targeted review focuses solely on the ICL implementations of pre-trained generative models for ABSA tasks, excluding those involving fine-tuning to draw a distinction from other non-ICL deep-learning approaches covered in the SLR. To extend the SLR sample beyond the original extraction time, we conducted a new database search Footnote 2 in July 2024 for studies published from 2022 onwards and removed the ones already included in the SLR sample. The new search results were screened using the SLR criteria described in Table 1 and then combined with the 519 SLR final samples. We then applied an additional filtering condition “Gen-LLM” to all the in-scope ABSA primary studies, which further selected publications with at least one occurrence of any of the following keywords outside the Reference section: “generative”, “in-context”, “in context learning”, “genai”, “bart”, “t5”, “flan-t5”, “gpt”, “chatgpt”, “llama”, and “mistral”. With the help of our automatic screening pipeline detailed in Appendix B.2 , we were able to efficiently auto-screen the new search results and re-screen the previous SLR sample for the ”Gen-LLM” keywords in less than one hour.
In total, the new search yielded 271 additional in-scope ABSA primary studies from 4359 search results. After applying the “Gen-LLM” filtering condition to the combined 790 in-scope ABSA primary studies, we obtained 208 Phase-2 samples for manual review, which comprised 91 studies from the new search and 117 from the earlier SLR sample, ranging from 2008 to 2024. The Phase-2 targeted review results are presented in Sect. 4.5 . Unless specified otherwise, the results below only refer to those of the SLR.
This section presents the SLR results corresponding to each of the RQs:
4.1 Results for RQ1
To answer RQ1, we examined the distribution of reviewed studies by their research (application) domains, dataset domains, and the relationship between the two. From the 519 reviewed studies, we recorded 218 datasets, 19 domain categories (15 research domains and 17 dataset domains), and obtained 1179 distinct “study-dataset” pairs and 630 unique “study & dataset-domain” combinations. The key results are summarised below and presented in Table 2 and Fig. 2 . We also list the datasets used by more than one reviewed study in the Appendix Table 15 .
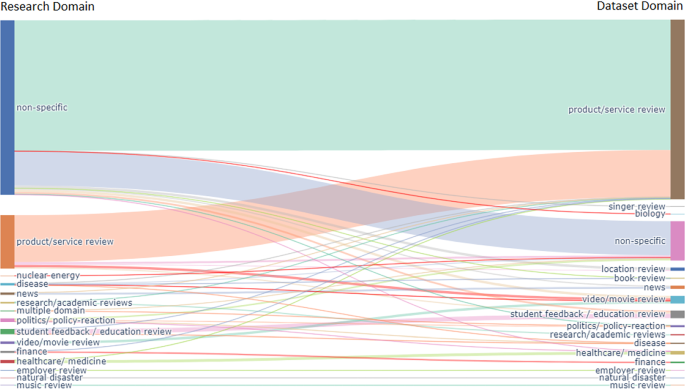
Distribution of unique “study–dataset” pairs ( \(\textrm{N}=1179\) , with 519 studies and 218 datasets) by research (application) domains (left) and dataset domains (right). Note (1) The top flow visualises a mismatch between the two domains: the majority of studies without a specific research domain used datasets from the product/service review domain. (2) The disproportionately small number of samples in both domains that were neither “non-specific” nor “product/service review”
In summary, our results answer RQ1 by showing that: (1) The majority (65.32%) of the reviewed studies were not for any specific application domain and only 24.28% targeted “product/service review”. (2) The dataset resources used in the sample were mostly domain-specific (84.44%) and dominated by the “product/service review” datasets (70.95%). (3) Both the research effort and dataset resources were scant in the non-commercial domains, especially the main public sector areas, with fewer than 13 studies across 14 years in each of the healthcare, policy, and education domains, where about half of the used datasets were created from scratch for the study.
Beyond RQ1, (1) and (2) above also suggest a significant mismatch between the research and dataset domains as visualised in Fig. 2 . Further, when filtering out datasets used by less than 10 studies, we discovered an alarming lack of dataset diversity as only 12 datasets remained, of which 10 were product/service reviews. When examining the three-way relationship among research domain, dataset domain, and dataset name, we further identified an over-representation (78.20%) of the four SemEval restaurant and laptop review benchmark datasets. This is illustrated in Fig. 4 .
4.1.1 Detailed results for RQ1
For research (application) domains indicated by the stated research use case or motivation, the majority (65.32%, \(\textrm{N}=339\) ) of the 519 reviewed studies have a “non-specific” research domain, followed by just a quarter (24.28%, \(\textrm{N}=126\) ) in the “product/service review” category. However, the number of studies in the rest of the research domains is magnitudes smaller in comparison, with only 12 studies (2.31%) in the third largest category “student feedback/education review” since 2008, followed by 8 in Politics/policy-reaction (1.54%), and only 7 in Healthcare/medicine (1.35%). Figure 3 revealed further insights from the trend of research domain categories with five or more reviewed studies. Interestingly, “product/service review” has been a persistently major category over time, and has only been consistently taken over by “non-specific” since 2015. The sharp increase of domain-“non-specific” studies since 2018 could be partly driven by the rise of pre-trained language models such as BERT and the greater sequence processing power from the Transformer architecture and the attention mechanism (Manning 2022 ), as more researchers explore the technicalities of ABSA solutions.
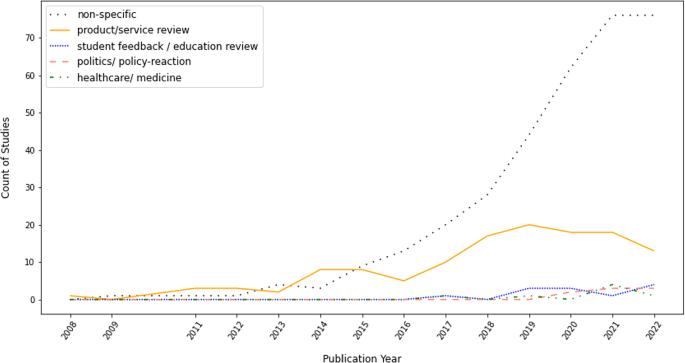
Number of in-scope studies by research (application) domain and publication year ( \(\textrm{N}=518\) ). This graph excludes the one 2023 study (extracted in October 2022) to avoid trend confusion
As to the dataset domains, Table 2 suggests that among the 630 unique “study & dataset-domain” pairs, the majority (70.95%, \(\textrm{N}=447\) ) are in the “product/service review” category, followed by 15.56% ( \(\textrm{N}=98\) ) in “Non-specific”. The third place is shared by two magnitude-smaller categories: “student feedback/ education review” (3.02%, \(\textrm{N}=19\) ) and “video/movie review” (3.02%, \(\textrm{N}=19\) ). The numbers of studies with datasets from the Healthcare/medicine (1.43%, \(\textrm{N}=9\) ) and Politics/policy-reaction (0.79%, \(\textrm{N}=5\) ) domains were again single-digit. Moreover, nearly half of the unique datasets in the public domains were created by the authors for the first time: 5/9 in Healthcare/medicine, 2/4 in Politics/policy-reaction, and 8/12 in Student feedback/ Education review.
Furthermore, to understand the dataset diversity across samples and domains, we grouped the 1179 unique “study-dataset” pairs by “research-domain, dataset-domain, dataset-name” combinations and zoomed into the 757 entries with ten or more study counts each. As shown in Table 3 and illustrated in Fig. 4 , among these 757 unique combinations, 95.77% ( \(\textrm{N}=725\) ) are in the “non-specific” research domain, of which 90.48% ( \(\textrm{N}=656\) ) used “product/service review” datasets. Most interestingly, these 757 entries only involve 12 distinct datasets of which 10 were product and service reviews, and 78.20% ( \(\textrm{N}=592\) ) are taken up by the four SemEval datasets from the early pioneer work (Pontiki et al 2014 , 2015 , 2016 ) mentioned in Sect. 2.4 : SemEval 2014 Restaurant, SemEval 2014 Laptop (these two alone account for 50.33% of all 757 entries), SemEval 2016 Restaurant, and SemEval 2015 Restaurant. This finding echos (Xing et al 2020 ; Chebolu et al 2023 ): “The SemEval challenge datasets... are the most extensively used corpora for aspect-based sentiment analysis” (Chebolu et al 2023 , p.4). Meanwhile, the top dataset used under “product/service review” research and dataset domains is the original product review dataset created by the researchers. Chebolu et al ( 2023 ) and Wikipedia ( 2023 ) provides a detailed introduction to the SemEval datasets.
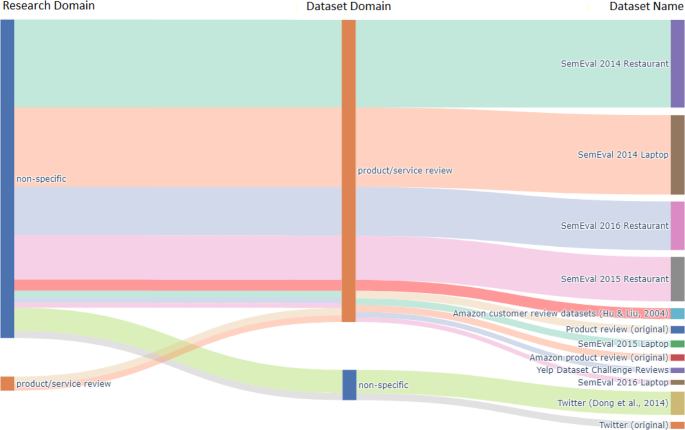
Number of studies per each research (application) domain (left), dataset domain (middle), and dataset (right) combination, filtered by datasets used by 10 or more in-scope studies ( \(\textrm{N}=757\) ). The three-way relationship highlights that not only did the majority of the sample studies with “non-specific” research domain use datasets from the ‘product/service review‘ domain, but their datasets were also dominated by only four SemEval datasets on two types of product and service reviews
It is noteworthy that among the 519 reviewed studies, 20 focused on cross-domain or domain-agnostic ABSA, and 19 of them did not have a specific research application domain. However, while all 20 studies used multiple datasets, 17 solely involved the “product/service review” domain category by using reviews of restaurants and different products, and 14 used at least one SemEval dataset. The only three studies that went beyond the “product/service review” dataset domain added in movie reviews, singer reviews, and generic tweets.
4.2 Results for RQ2
RQ2. What are the most common ABSA problem formulations via subtask combinations, and what proportion of ABSA studies only focus on a specific sub-task?
For RQ2, we examined the 13 recorded subtasks and 805 unique “study-subtask” pairs to identify the most explored ABSA subtasks and subtask combinations across the 519 reviewed studies. As shown in Fig. 5 a, 32.37% ( \(\textrm{N}=168\) ) of the studies developed full-ABSA solutions through the combination of AE and ASC, and a similar proportion (30.83%, \(\textrm{N}=160\) ) focused on ASC alone, usually formulating the research problem as contextualised sentiment analysis with given aspects and the full input text. Only 15.22% ( \(\textrm{N}=79\) ) of the studies solely explored the AE problem. This is consistent with the number of studies by individual subtasks shown in Fig. 5 b, where ASC is the most explored subtask, followed by AE and ACD.
Moreover, Fig. 6 reveals a small but noticeable rise in composite subtask ASTE since 2020 ( \(\textrm{N}=1\) , 5 and 10 in 2017, 2021, 2022) and a decline in ASC and AE around the same period. This could signify a problem formulation shift driven by deep-learning, especially multi-task learning methods for E2E ABSA. Our Phase-2 targeted review findings in Sect. 4.5 add more insights into this.
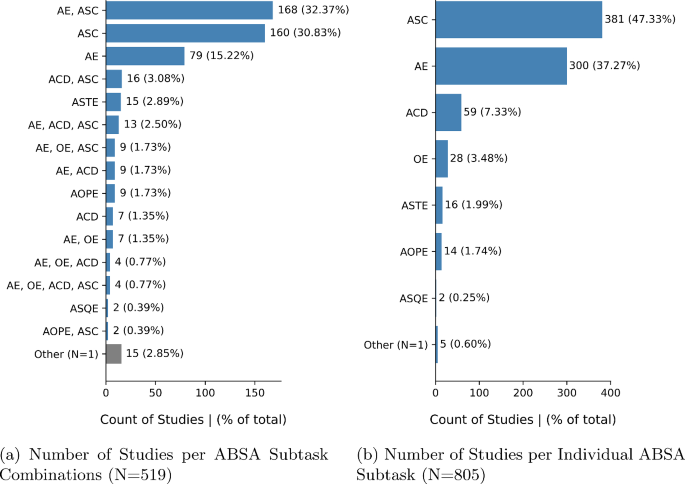
Number of studies by ABSA subtask
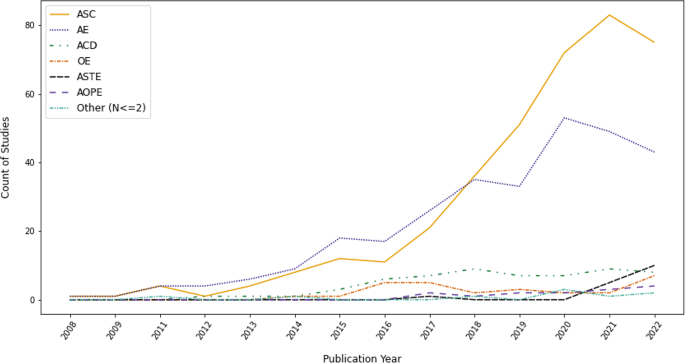
Distribution of unique “Study–ABSA subtask” pairs by publication year ( \(\textrm{N}=805\) ). This graph excludes the one 2023 study (extracted in October 2022) to avoid trend confusion
4.3 Results for RQ3
To answer RQ3, we examined the 519 in-scope studies along two dimensions, which we call “paradigm” and “approach”. We use “ paradigm ” to indicate whether a study employed techniques along the supervised-unsupervised dimension and other types, such as reinforcement learning. We classify non-machine-learning approaches under the “unsupervised” paradigm, as our focus is on dataset and resource dependency. By “ approach ”, we refer to the more specific type of techniques, such as deep learning (DL), traditional machine learning (traditional ML), linguistic rules (“rules” for short), syntactic features and relations (“syntactics” for short), lexicon lists or databases (“lexicon” for short), and ontology or knowledge-driven approaches (“ontology” for short).
Overall, the results suggest that our samples are dominated by fully- (60.89%) and partially-supervised (5.40%) ML methods that are more reliant on annotated datasets and prone to their impact. As to ABSA solution approaches, the sample shows that DL methods have rapidly overtaken traditional ML methods since 2017, particularly with the prevalent RNN family (55.91%) and its combination with the fast-surging attention mechanism (26.52%). Meanwhile, traditional ML and linguistic approaches have remained a small but steady force even in the most recent years. Context engineering through introducing linguistic and knowledge features to DL and traditional ML approaches was very common. More detailed results and richer findings are presented below.
4.3.1 Paradigms
Table 4 lists the number of studies per each of the main paradigms. Among the 519 reviewed studies, 66.28% ( \(\textrm{N}=344\) ) is taken up by those using somewhat- (i.e. fully-, semi- and weakly-) supervised paradigms that have varied levels of dependency on labelled datasets, where the fully-supervised ones alone account for 60.89% ( \(\textrm{N}=316\) ). Only 19.65% ( \(\textrm{N}=102\) ) of the studies do not require labelled data, which are mostly unsupervised (18.69%, \(\textrm{N}=97\) ). In addition, hybrid studies are the third largest group (14.07%, \(\textrm{N}=73\) ).
We further analysed the approaches under each paradigm and focused on three for more details: deep learning (DL), traditional machine learning (ML), and Linguistic and Statistical Approaches. The results are detailed below and presented in Fig. 7 and Tables 5 , 6 .
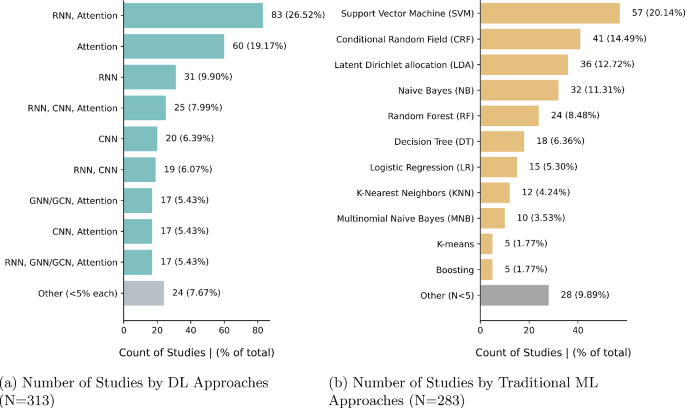
Number of studies using DL and traditional ML approaches
4.4 Approaches
As shown in Fig. 7 a and Table 5 , among the 519 reviewed studies, 60.31% ( \(\textrm{N}=313\) ) employed DL approaches, and 30.83% ( \(\textrm{N}=160\) ) are DL-only. The DL-only approach is particularly prominent among fully-supervised (47.15%, \(\textrm{N}=149\) ) and semi-supervised (31.82%, \(\textrm{N}=7\) ) studies. Supplementing DL with syntactical features is also the second most popular approach in fully-supervised studies (16.77%, \(\textrm{N}=53\) ).
DL Approaches
Figure 7 a suggests that the 313 studies involving DL approaches are dominated by Recurrent Neural Network (RNN)-based solutions (55.91%, \(\textrm{N}=175\) ), of which nearly half used a combination of RNN and the attention mechanism (26.52%, \(\textrm{N}=83\) ), followed by attention-only (19.17%, \(\textrm{N}=60\) ) and RNN-only (9.90%, \(\textrm{N}=31\) ) models. The RNN family mainly consists of Long Short-Term Memory (LSTM), Bidirectional LSTM (BiLSTM), and Gated Recurrent Unit (GRU). These neural-networks are featured by sequential processing that captures temporal dependencies of text tokens, and can thus incorporate surrounding text as context for prediction (Liu et al 2020 ; Satyarthi and Sharma 2023 ). On the other hand, the sequential nature poses challenges with parallelisation and the exploding and vanishing gradient problems associated with long sequences (Vaswani et al 2017 ; Liu et al 2020 ). Although LSTM and GRU can mitigate these issues somewhat through cell state and memory controls, efficiency and long-dependency challenges still hinder their performance (Vaswani et al 2017 ; Liu et al 2020 ; Satyarthi and Sharma 2023 ). The attention mechanism complements RNNs by dynamically updating weights across the input sequence based on each element’s relevance to the current task, and thus guides the model to focus on the most relevant elements (Vaswani et al 2017 ).
In addition, convolutional and graph-neural approaches [e.g. convolutional neural networks (CNN), graph neural networks (GNN), graph convolutional networks (GCN)] also play smaller but noticeable roles in DL-based ABSA studies. While CNN was commonly used as an alternative to the sequence models such as RNNs (Liu et al 2020 ; J et al 2021 ; Zhang et al 2020 ), the graph-based networks (GNN, GCN) were mainly used to model the non-linear relationships such as external conceptual knowledge (e.g. Liang et al 2022 ) and syntactic dependency structures (e.g. Fei et al 2023a , b ; Li et al 2022c ) that are not well captured by the sequential networks like RNNs and the flat structure of the attention modules. As a result, they inject richer context into the overall learning process (Du et al 2021 ; Xu et al 2020a ; Wang et al 2022 ).
Figure 8 depicts the trend of the main approaches across the publication years. We excluded the one study pre-published for 2023 to avoid confusing trends. It is clear that DL approaches have risen sharply and taken dominance since 2017, mainly driven by the rapid growth in RNN- and attention-based studies. This coincides with the appearance of the Transformer architecture in 2017 (Vaswani et al 2017 ) and the resulting pre-trained models such as BERT (Devlin et al 2019 ) that were a popular embedding choice to be used alongside RNNs in DL and hybrid approaches (e.g., Li et al 2021 ; Zhang et al 2022a ). GNN/GCN-based approaches remain small in number but have noticeable growth since 2020 ( \(\textrm{N}=2\) , 2, 16, 24 in each of 2019–2022, respectively), suggesting an increased effort to dynamically integrate relational context into the learning process within the DL framework.
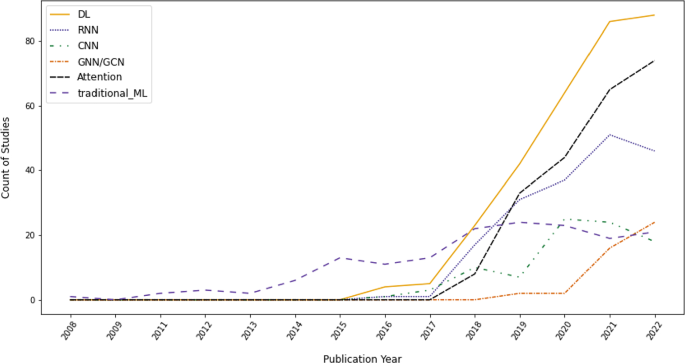
Distribution of studies per method by publication year ( \(\textrm{N}=1017\) with 519 unique studies). This graph excludes the one 2023 study (extracted in October 2022) to avoid trend confusion
Traditional ML approaches
Interestingly, as shown in Fig. 8 traditional ML approaches remain a steady force over the decades despite the rapid rise of DL methods. Table 5 and Fig. 7 b provide some insight into this: Among the 519 reviewed studies, while 60.31% employed DL approaches as mentioned in the previous sub-section, over half (54.53%, \(\textrm{N}=283\) ) also included traditional ML approaches, with the top 3 being Support Vector Machine (SVM; 20.14%, \(\textrm{N}=57\) ), Conditional Random Field (CRF; 14.49%, \(\textrm{N}=41\) ), and Latent Dirichlet allocation (LDA; 12.72%, \(\textrm{N}=36\) ). Table 5 suggests that among the major paradigms, traditional ML were often used in combination with DL approaches for fully-supervised studies (7.28%, \(\textrm{N}=23\) ), and along with linguistic rules, syntactic features, and/or lexicons and ontology in hybrid studies (27.40%, \(\textrm{N}=20\) ). Across all paradigms, traditional ML-only approaches are relatively rare (max \(\textrm{N}=7\) ).
Linguistic and statistical approaches
While Table 5 illustrates the prevalence of fusing ML approaches with linguistic and statistical features or modules, there were 67 studies (12.91% out of the total 519) on pure linguistic or statistical approaches. As shown in Table 6 , although small in number, these non-ML approaches have persisted over time. The most popular combination (34.33%, \(\textrm{N}=23\) ) was rules built on syntactic features (e.g. POS tags and dependency parse trees) and used along lexicon resources (e.g. domain-specific aspect lists, SentiWordNet, Footnote 3 MPQA Footnote 4 ). A typical example is using POS tags and/or lexicon resources to narrow the scope of aspect or opinion term candidates, applying further rules based on POS tags or dependency relations for AE or OE, and/or using lexicon resources for candidate pruning, categorisation, or sentiment labelling (e.g. Asghar et al 2019 ; Dragoni et al 2019 ; Nawaz et al 2020 ). The second top combination (23.88%, \(\textrm{N}=16\) ) is the above-mentioned one plus ontology (e.g. domain-specific ontology, ConceptNet, Footnote 5 WordNet Footnote 6 ) to bring in external knowledge of concepts and relations (e.g. Federici and Dragoni 2016 ; Marstawi et al 2017 ). Pure statistical methods were relatively rare (5.97%, \(\textrm{N}=4\) ), and mainly included frequency-based methods such as N-gram and TF-IDF, and other statistical modelling methods that were not commonly seen in the ML field.
4.5 ICL and generative approach in ABSA - Phase-2 targeted review results
ICL is a subgenre of the DL approach. However, we discuss the relevant results in this separate subsection due to the Phase-2 review’s more focused sample and finer granularity. Despite the trending popularity of the ICL approach in NLP research and applications since 2022 (Dong et al 2024 ), our results suggest that the ABSA research community is just beginning to explore it with caution. Among the 208 ABSA studies from 2008 to 2024 containing at least one occurrence of the “Gen-LLM” keywords, only five (all published in 2024) applied ICL to both composite and traditional ABSA tasks. All of these studies were exploring the performance of foundation models via ICL against other approaches, rather than focusing on an ICL ABSA solution. Table 7 summarises the models, ABSA tasks, and key findings from these studies. Overall, four of the five studies found that zero-shot and even 5-shot ICL on foundation models (mainly GPTs) could not reach the performance of fine-tuned or fully trained DL models, especially those leveraging pre-trained LLMs to fine-tune a contextual-embedding.
In addition, we identified an emerging trend by examining the Phase-2 review non-ICL samples: Those employing fine-tuned generative LLMs mostly formulated the ABSA tasks as Sequence-to-Sequence (Seq2Seq) text generation problems, with a particular focus on composite tasks such as ASTE and ASQE. As shown in Table 8 , within the 208 samples, a total of 18 studies (all from the new search) published in 2022–2024 applied pre-trained generative LLMs with fine-tuning. The majority of these studies used models based on T5 ( \(\textrm{N}=9\) ) and BART ( \(\textrm{N}=5\) ) with the full Transformer (Vaswani et al 2017 ) encoder-decoder architecture, followed by encoder-only ( \(\textrm{N}=3\) , BERT and RoBERTa Liu et al 2019 ) and decoder-only ( \(\textrm{N}=1\) , GPT-2 Radford et al 2019 ) models. All but two of these 18 studies were on composite ABSA tasks, mainly ASTE and ASQE. Moreover, two studies (Yu et al 2023b ; Zhang et al 2024b ) also leveraged the generative capability of these LLMs to augment training data to enrich the fine-tuned embedding.
Compared with this Seq2Seq generation approach, the common applications of pre-trained LLMs in earlier studies from the main SLR sample often formulate the ABSA task as a classification problem (Zhang et al 2022c ). These studies mostly use encoder-only LLMs for their pre-trained representations to fine-tune a contextual embedding (Zhang et al 2022c ), which is then connected to other context-injection or relationship-learning modules and a classifier output layer. For instance, Zhang et al ( 2022a ) employed pre-trained BERT with BiLSTM, a feed-forward neural network (FFNN), and CRF. Li et al ( 2021 ) used pre-trained BERT as an encoder and a decoder featuring a GRU. In contrast, the Seq2Seq generative approach can be illustrated by the signature “Generative Aspect-based Sentiment analysis (GAS)” proposed by Zhang et al ( 2021b ), which leveraged the LLM’s pre-trained and fine-tuned encoder module for context-aware embedding and used the fine-tuned decoder module to generate text representations of the label sets (e.g., triplets) or as annotations next to the original input text (Zhang et al 2021b , 2022c ).
5 Discussion
This review was motivated by the literature gap in capturing trends in ABSA research to answer higher-level questions beyond technical details, and the concern that the domain-dependent nature could predispose ABSA research to systemic hindrance from a combination of resource-reliant approaches and skewed resource domain distribution. By systematically reviewing the two waves of 727 in-scope primary studies published between 2008 and 2024, our quantitative analysis results identified trends in ABSA solution approaches, confirmed the above-mentioned concern, and provided detailed insights into the relevant issues. In this section, we examine the primary findings, share ideas for future research, and reflect on the limitations of this review.
5.1 Significant findings and trends
5.1.1 the out-of-sync research and dataset domains.
Under RQ1, we examined the distributions of and relationships between our sample’s research (application) domains and dataset domains. The results showed strong skewness in both types of domains and a significant mismatch between them: While the majority (65.32%, \(\textrm{N}=339\) ) of the 519 studies did not aim for a specific research domain, a greater proportion (70.95%, \(\textrm{N}=447\) ) used datasets from the “product/service review” domain. A closer inspection of the link between the two domains revealed a clear mismatch: Among the 757 unique “research-domain, dataset-domain, dataset-name” combinations with ten or more studies: 90.48% ( \(\textrm{N}=656\) ) of the studies in the “non-specific” research domain (95.77%, \(\textrm{N}=725\) ) used datasets from the “product/service review” domain. This suggests that the lack of non-commercial-domain datasets could have forced generic technical studies to use benchmark datasets from a single popular domain. Given ABSA problem’s domain-dependent nature, this could have indirectly hindered the solution development and evaluation across domains.
The results also showed that the other important and prevalent ABSA application domains such as education, medicine/healthcare, and public policy, were clearly under-researched and under-resourced. Among the reviewed samples from these three public-sector domains, about half of their datasets were created for the studies by their authors, indicating a lack of public dataset resources, hence the cost and challenge of developing ABSA research in these areas. As a likely consequence, even the most researched domain among these three had only 12 studies (2.31% out of 519) since 2008. The dataset resource scarcity in these public sector domains deserves more research community attention and support, especially given these domains’ overall low research resources vs. the high cost and domain knowledge required for quality data annotation. In particular, for domains such as “Student feedback/education review” that often face strict data privacy and consent restrictions, it is crucial that the ABSA research community focus on creating ethical and open-access datasets to leverage community resources.
5.1.2 The dominance and limitations of the SemEval datasets
The results under RQ1 also revealed further issues with dataset diversity, even within the dominant “product/service review” domain. Out of the 757 unique “research-domain, dataset-domain, dataset-name” combinations with ten or more studies, 78.20% ( \(\textrm{N}=592\) ) are taken up by the four popular SemEval datasets: The SemEval 2014 Restaurant and Laptop datasets alone account for 50.33% of all 757 entries, and the other two (SemEval 2015 and 2016 Restaurant).
The level of dominance of the SemEval datasets is alerting, not only because of their narrow domain range, but also for the inheritance and impact of the SemEval datasets’ limitations. Several studies (e.g. Chebolu et al 2023 ; Xing et al 2020 ; Jiang et al 2019 ; Fei et al 2023a ) suggest that these datasets fail to capture sufficient complexity and granularity of the real-world ABSA scenarios, as they primarily only include single-aspect or multi-aspect-but-same-polarity sentences, and thus mainly reflect sentence-level ABSA tasks and ignored subtasks such as multi-aspect multi-sentiment ABSA. The experiment results from Xing et al ( 2020 ), Jiang et al ( 2019 ) and Fei et al ( 2023a ) consistently showed that all 35 ABSA models (including those that were state-of-the-art at the time) (9 in Xing et al 2020 , 16 in Jiang et al 2019 , 10 in Fei et al 2023a ) that were trained and performed well on the SemEval 2014 ABSA datasets showed various extents of performance drop (by up to 69.73% in Xing et al 2020 ) when tested on same-source datasets created for more complex ABSA subtasks and robustness challenges. Given that the SemEval datasets are heavily used as both training data and “benchmark” to measure ABSA solution performance, their limitations and prevalence are likely to form a self-reinforcing loop that confines ABSA research. To break free from this dataset-performance self-reinforcing loop, it is critical that the ABSA research community be aware of this issue, and develop and adopt datasets and practices that are robustness-oriented, such as the automatic data-generation framework and the resulting Aspect Robustness Test Set (ARTS) developed by Xing et al ( 2020 ) for probing model robustness in distinguishing target and non-target aspects, and the Multi-Aspect Multi-Sentiment (MAMS) dataset created by Jiang et al ( 2019 ) to reflect more realistic challenges and complexities in aspect-term sentiment analysis (ATSA) and aspect-category sentiment analysis (ACSA) tasks.
5.1.3 The reliance on labelled-datasets
The domain and dataset issues discussed above would not be as problematic if most ABSA studies employed methods that are dataset-agnostic. However, our results under RQ3 show the opposite. Only 19.65% ( \(\textrm{N}=102\) , with 97 being unsupervised) of the 519 reviewed studies do not require labelled data, whereas 66.28% ( \(\textrm{N}=344\) ) are somewhat-supervised, and fully-supervised studies alone account for 60.89% ( \(\textrm{N}=316\) ).
As demonstrated in Sect. 2.3 , the domain can directly affect whether a chunk of text is considered an aspect or the relevant sentiment term, and plays a crucial role in contextual inferences such as implicit aspect extraction and multi-aspect multi-sentiment pairing. The domain knowledge reflected via ABSA labelled datasets can further shape the linguistic rules, lexicons, and knowledge graphs for non-machine-learning approaches; and define the underpinning feature space, representations, and acquired relationships and inferences for trained machine-learning models. When applying a solution built on datasets from a domain that is very remote from or much narrower than the intended application domain, it is predictable that the solution performance would be capped at subpar and even fail at more context-heavy tasks (Phan and Ogunbona 2020 ; You et al 2022 ; Liang et al 2022 ; Howard et al 2022 ; Chen and Qian 2022 ; Zhang et al 2022b ; Nazir et al 2022b ). Thus, domain transfer is crucially necessary for balancing the uneven ABSA research and resource distributions across domains. However, our finding that 17 out of the 20 reviewed cross-domain or domain-agnostic ABSA studies solely used datasets from the “product/service review” domain raised questions about these approaches’ generalisability and robustness in other domains, as well as whether such dataset choices became another reinforcement of concentrating research effort and benchmarks within this one dominant domain.
The rapid rise of deep learning (DL) in ABSA research could further add to the challenge of overcoming the negative impact of this domain mismatch and dataset limitations via the non-linear multi-layer dissemination of bias in the representation and learned relations, thus making problem-tracking and solution-targeting difficult. In reality, of the 519 reviewed studies, 60.31% ( \(\textrm{N}=313\) ) employed DL approaches, and nearly half (47.15%, \(\textrm{N}=149\) ) of the fully-supervised studies and 30.83% ( \(\textrm{N}=160\) ) of all reviewed studies were DL-only.
Moreover, RNN-based solutions dominate the DL approaches (55.91%, \(\textrm{N}=175\) ), mainly with the RNN-attention combination (26.52%, \(\textrm{N}=83\) ) and RNN-only (9.90%, \(\textrm{N}=31\) ) models. RNN and its variants such as LSTM, BiLSTM, and GRU are known for their limitations in capturing long-distance relations due to their sequential nature and the subsequent memory constraints (Vaswani et al 2017 ; Liu et al 2020 ). Although the addition of the attention mechanism enhances the model’s focus on more important features such as aspect terms (Vaswani et al 2017 ; Liu et al 2020 ), traditional attention weights calculation struggles with multi-word aspects or multi-aspect sentences (Liu et al 2020 ; Fan et al 2018 ). In addition, whilst 16.77% ( \(N=53\) ) of the fully-supervised studies introduced syntactical features to their DL solutions, additional features also increased the input size. According to Prather et al ( 2020 ), sequential models, even the state-of-the-art LLMs, showed impaired performance as the input grew longer and could not always benefit from additional features.
5.1.4 The potential of generative LLMs and foundation models
Lastly, the Phase-2 targeted review highlights the ABSA community’s caution toward the direct adoption of generative foundation models, with only five out of 208 recent studies testing the ICL approach and most yielding subpar results compared to other methods. However, most of these studies only tested zero-shot instructions with simple model settings. It is worth further exploring the potential of foundational models and ICL in ABSA by focusing more on instruction and example engineering, model parameter optimisation, and task re-formulation (Dong et al 2024 ).
On the other hand, the fine-tuning of smaller generative LLMs has seen increasing adoption through the “ABSA as Seq2Seq text generation” approach, demonstrating promising task performance. Although this generative approach can incorporate data augmentation and self-training to reduce reliance on labelled datasets, the cost of fine-tuning, the need for labelled base data, and the domain-transfer problem remain significant challenges (Zhang et al 2022c ). In this context, the task adaptability and multi-domain pre-trained knowledge of foundation models could provide potential solutions.
As Zhang et al ( 2022c ) noted, progress in applying pre-trained LLMs and foundation models to ABSA could be impeded by dataset resources constraints. To match the parameter size of these models, more diverse, complex, and larger datasets are required for effective fine-tuning or comprehensive testing. In low-resource domains where dataset resources are already limited, this requirement could further complicate the adoption of these technologies (Satyarthi and Sharma 2023 ).
5.2 Ideas for future research
Overall, by adopting a “systematic perspective, i.e., model, data, and training” (Fei et al 2023a , p.28) combined with a quantitative approach, we identified high-level trends unveiling the development and direction of ABSA research, and found clear evidence of large-scale issues that affect the majority of the existing ABSA research. The skewed domain distributions of resources and benchmarks could also restrict the choice of new studies. On the other hand, this evidence also highlights areas that need more attention and exploration, including: ABSA solutions and resource development for the less-studied domains (e.g. education and public health), low-resource and/or data-agnostic ABSA, domain adaptation, alternative training schemes such as adversarial (e.g. Fei et al 2023a ; Chen et al 2021 ) and reinforcement learning (e.g. Vasanthi et al 2022 ; Wang et al 2021b ), and more effective feature and knowledge injection. Future research could contribute to addressing these issues by focusing on ethically producing and sharing more diverse and challenging datasets in minority domains such as education and public health, improving data synthesis and augmentation techniques, exploring methods that are less data-dependent and resource-intensive, and leveraging the rapid advancements in pre-trained LLMs and foundation models.
In addition, our results also revealed emerging trends and new ideas. The relatively recent growth of end-to-end models and composite ABSA subtasks provide opportunities for further exploration and evaluation. The fact that hybrid approaches with non-machine-learning techniques and non-textual features remain steady forces in the field after nearly three decades suggests valuable characteristics that are worth re-examining under the light of new paradigms and techniques. Moreover, the small number of Phase-2 samples using ICL and fine-tuning generative LLM approaches may suggest that we have only captured early adopters. More thorough exploration of these approaches and continued tracking of their development alongside other methods are necessary to understand how the ABSA community can leverage the resources and capabilities embedded within LLMs and foundation models.
Lastly, it is crucial that the community invest in solution robustness, especially for machine-learning approaches (Xing et al 2020 ; Jiang et al 2019 ; Fei et al 2023a ). This could mean critical examination of the choice of evaluation metrics, tasks, and benchmarks, and being conscious of their limitations vs. the real-world challenges. The “State-Of-The-Art” (SOTA) performance based on certain benchmark datasets should never become the motivation and holy grail of research, especially in fields like ABSA where the real use cases are often complex and even SOTA models do not generalise far beyond the training datasets. More attention and effort should be paid to analysing the limitations and mistakes of ABSA solutions, and drawing from the ideas of other disciplines and areas to fill the gaps.
5.3 Limitations
We acknowledge the following limitations of this review: First, our sample scope is by no means exhaustive, as it only includes primary studies from four peer-reviewed digital databases and only those published in the English language. Although this can be representative of a core proportion of ABSA research, it does not generalise beyond this without assumptions. The “peer-reviewed” criteria also meant that we overlooked preprint servers such as arXiv.org that more closely track the latest development of ML and NLP research. Second, no search string is perfect. Our database search syntax and auto-screening keywords represent our best effort in capturing ABSA primary studies, but may have missed some relevant ones, especially with the artificial “total pages \(< 3\) ” and “total keyword (except SA, OM) outside Reference \(< 5\) ” exclusion criteria. Moreover, our search completeness might have been affected by the performance of the database search engines. This is evidenced by the significant number of extracted search results that were entirely irrelevant to the search keywords, as well as our abandonment of the 2024 SpringerLink search due to interface issues. Enhancements in digital database search capabilities could significantly improve the effectiveness and reliability of future literature review studies, particularly SLRs. Third, we may have missed datasets, paradigms, and approaches that are not clearly described in the primary studies, and our categorisation of them is also subject to the limitations of our knowledge and decisions. Future review studies could consider a more innovative approach to enhance analytical precision and efficiency, such as applying ABSA and text summarisation alongside the screening and reviewing process. Fourth, we did not compare solution performance across studies due to the review focus, sample size, and the variability in experimental settings across studies. Evaluating the effectiveness of comparable methods and the suitability of evaluation metrics would enhance our findings and offer more valuable insights.
6 Conclusion
ABSA research is riding the wave of the explosion of online digital opinionated text data and the rapid development of NLP resources and ideas. However, its context- and domain-dependent nature and the complexity and inter-relations among its subtasks pose challenges to improving ABSA solutions and applying them to a wider range of domains. In this review, we systematically examined existing ABSA literature in terms of their research application domain, dataset domain, and research methodologies. The results suggest a number of potential systemic issues in the ABSA research literature, including the predominance of the “product/service review” dataset domain among the majority of studies that did not have a specific research application domain, coupled with the prevalence of dataset-reliant methods such as supervised machine learning. We discussed the implication of these issues to ABSA research and applications, as well as their implicit effect in shaping the future of this research field through the mutual reinforcement between resources and methodologies. We suggested areas that need future research attention and proposed ideas for exploration.
Our PDF mining for automatic review screening code is available at https://doi.org/10.5281/zenodo.12872948 .
This new database search followed the same procedures and criteria as the SLR, except that we aborted the SpringerLink search due to persistent database interface search result navigation issues during our data collection period.
https://github.com/aesuli/SentiWordNet .
https://mpqa.cs.pitt.edu/ .
https://conceptnet.io/ .
https://wordnet.princeton.edu/ .
https://alt.qcri.org/semeval2014/task4/ .
https://alt.qcri.org/semeval2015/task12/ .
https://pypi.org/project/PyMuPDF/ .
https://pdfminersix.readthedocs.io/en/latest/faq.html .
Akhtar MS, Ekbal A, Bhattacharyya P (2016) Aspect based sentiment analysis in Hindi: Resource creation and evaluation. In: Calzolari N, Choukri K, Declerck T, et al (eds) Proceedings of the Tenth International Conference on Language Resources and Evaluation (LREC’16). European Language Resources Association (ELRA), Portoroˇz, Slovenia, pp 2703–2709. https://aclanthology.org/L16-1429
Akhtar MS, Gupta D, Ekbal A et al (2017) Feature selection and ensemble construction: a two-step method for aspect based sentiment analysis. Knowl Based Syst 125:116–135. https://doi.org/10.1016/j.knosys.2017.03.020 . https://linkinghub.elsevier.com/retrieve/pii/S095070511730148X
Akhtar MS, Ekbal A, Bhattacharyya P (2018) Aspect based sentiment analysis: category detection and sentiment classification for Hindi. In: Gelbukh A (ed) Computational linguistics and intelligent text processing, vol 9624. Lecture Notes in Computer Science. Springer, Cham, pp 246–257. https://doi.org/10.1007/978-3-319-75487-1_19
Chapter Google Scholar
Akhtar MS, Garg T, Ekbal A (2020) Multi-task learning for aspect term extraction and aspect sentiment classification. Neurocomputing 398:247–256. https://doi.org/10.1016/j.neucom.2020.02.093 . https://linkinghub.elsevier.com/retrieve/pii/S0925231220302897
Almatrafi O, Johri A (2022) Improving MOOCs using information from discussion forums: an opinion summarization and suggestion mining approach. IEEE Access 10:15565–15573. https://doi.org/10.1109/ACCESS.2022.3149271 . https://ieeexplore.ieee.org/document/9706374/
Alyami S, Alhothali A, Jamal A (2022) Systematic literature review of Arabic aspect-based sentiment analysis. J King Saud Univ Comput Inf Sci 34(9):6524–6551. https://doi.org/10.1016/j.jksuci.2022.07.001 . https://linkinghub.elsevier.com/retrieve/pii/S1319157822002282
Amin MM, Mao R, Cambria E et al (2024) A wide evaluation of chatgpt on affective computing tasks. IEEE Trans Affect Comput 1–9. https://doi.org/10.1109/TAFFC.2024.3419593 . https://ieeexplore.ieee.org/document/10572294
Asghar MZ, Khan A, Zahra SR et al (2019) Aspect-based opinion mining framework using heuristic patterns. Cluster Comput 22(S3):7181–7199. https://doi.org/10.1007/s10586-017-1096-9
Article Google Scholar
Baccianella S, Esuli A, Sebastiani F (2010) SentiWordNet 3.0: An enhanced lexical resource for sentiment analysis and opinion mining. In: Calzolari N, Choukri K, Maegaard B et al (eds) Proceedings of the seventh international conference on language resources and evaluation (LREC’10). European Language Resources Association (ELRA), Valletta, Malta. http://www.lrec-conf.org/proceedings/lrec2010/pdf/769_Paper.pdf
Bommasani R, Hudson DA, Adeli E et al (2022) On the opportunities and risks of foundation models. arXiv:2108.07258
Brauwers G, Frasincar F (2023) A survey on aspect-based sentiment classification. ACM Comput Surv 55(4):1–37. https://doi.org/10.1145/3503044
Brown TB, Mann B, Ryder N et al (2020) Language models are few-shot learners. arXiv:2005.14165
Cambria E, Poria S, Bajpai R et al (2016) SenticNet 4: a semantic resource for sentiment analysis based on conceptual primitives. In: Matsumoto Y, Prasad R (eds) Proceedings of COLING 2016, the 26th international conference on computational linguistics: technical papers. The COLING 2016 Organizing Committee, Osaka, Japan, pp 2666–2677. https://aclanthology.org/C16-1251
Castellanos M, Dayal U, Hsu M, et al (2011) LCI: A social channel analysis platform for live customer intelligence. In: Proceedings of the 2011 ACM SIGMOD international conference on management of data, SIGMOD’11. Association for Computing Machinery, New York, pp 1049–1058. https://doi.org/10.1145/1989323.1989436
Cavalcanti D, Prudêncio R (2017) Aspect-based opinion mining in drug reviews. In: Oliveira E, Gama J, Vale Z et al (eds) Progress in artificial intelligence, vol 10423. Lecture Notes in Computer Science. Springer, Cham, pp 815–827. https://doi.org/10.1007/978-3-319-65340-2_66
Chauhan GS, Agrawal P, Meena YK (2019) Aspect-based sentiment analysis of students’ feedback to improve teaching-learning process. In: Satapathy SC, Joshi A (eds) Information and communication technology for intelligent systems, vol 107. Smart Innovation, Systems and Technologies. Springer Singapore, Singapore, pp 259–266. https://doi.org/10.1007/978-981-13-1747-7_25
Chebolu SUS, Dernoncourt F, Lipka N et al (2023) Survey of aspect-based sentiment analysis datasets. arXiv:2204.05232
Chen S, Fnu G (2022) Deep learning techniques for aspect based sentiment analysis. In: 2022 14th International conference on computer research and development (ICCRD), pp 69–73. https://doi.org/10.1109/ICCRD54409.2022.9730443 . https://ieeexplore.ieee.org/document/9730443
Chen Z, Liu B (2014) Topic modeling using topics from many domains, lifelong learning and big data. In: Proceedings of the 31st International Conference on International Conference on Machine Learning - Volume 32. JMLR.org, ICML’14, p II–703–II–711. https://proceedings.mlr.press/v32/chenf14.html
Chen Z, Qian T (2022) Retrieve-and-edit domain adaptation for end2end aspect based sentiment analysis. IEEE/ACM Trans Audio Speech Lang Process 30:659–672. https://doi.org/10.1109/TASLP.2022.3146052 . https://ieeexplore.ieee.org/document/9693267/
Chen M, Wu W, Zhang Y et al (2021) Combining adversarial training and relational graph attention network for aspect-based sentiment analysis with BERT. In: 2021 14th international congress on image and signal processing, BioMedical engineering and informatics (CISP-BMEI), pp 1–6. https://doi.org/10.1109/CISP-BMEI53629.2021.9624384 . https://ieeexplore.ieee.org/document/9624384
Chen F, Yang Z, Huang Y (2022) A multi-task learning framework for end-to-end aspect sentiment triplet extraction. Neurocomputing 479:12–21. https://doi.org/10.1016/j.neucom.2022.01.021 . https://linkinghub.elsevier.com/retrieve/pii/S0925231222000406
Chu JSG, Evans JA (2021) Slowed canonical progress in large fields of science. Proc Natl Acad Sci 118(41):e2021636118. https://doi.org/10.1073/pnas.2021636118 . https://pnas.org/doi/full/10.1073/pnas.2021636118
Cortis K, Davis B (2021) Over a decade of social opinion mining: a systematic review. Artif Intell Rev 54(7):4873–4965. https://doi.org/10.1007/s10462-021-10030-2
Cruz I, Gelbukh AF, Sidorov G (2014) Implicit aspect indicator extraction for aspect based opinion mining. Int J Comput Linguistics Appl 5(2):135–152. https://www.semanticscholar.org/paper/Implicit-Aspect-Indicator-Extraction-for-Aspect-Cruz-Gelbukh/8768fc3374b27c0ac023f5bf60da9ab50714b37e
Dang TV, Hao D, Nguyen N (2024) Vi-AbSQA: multi-task prompt instruction tuning model for Vietnamese aspect-based sentiment quadruple analysis. ACM Trans Asian Low-Resour Lang Inf Process. https://doi.org/10.1145/3676886 , just Accepted
Da’u A, Salim N, Rabiu I et al (2020) Weighted aspect-based opinion mining using deep learning for recommender system. Expert Syst Appl 140:112871. https://doi.org/10.1016/j.eswa.2019.112871 . https://linkinghub.elsevier.com/retrieve/pii/S0957417419305810
Devlin J, Chang M, Lee K et al (2019) BERT: pre-training of deep bidirectional transformers for language understanding. In: Burstein J, Doran C, Solorio T (eds) Proceedings of the 2019 conference of the North American chapter of the association for computational linguistics: human language technologies, NAACL-HLT 2019, Minneapolis, MN, USA, June 2–7, 2019, volume 1 (long and short papers). Association for Computational Linguistics, pp 4171–4186. https://doi.org/10.18653/V1/N19-1423
Do HH, Prasad P, Maag A et al (2019) Deep learning for aspect-based sentiment analysis: a comparative review. Expert Syst Appl 118:272–299. https://doi.org/10.1016/j.eswa.2018.10.003 . https://www.sciencedirect.com/science/article/pii/S0957417418306456
Dong L, Wei F, Tan C, et al (2014) Adaptive recursive neural network for target-dependent Twitter sentiment classification. In: Toutanova K, Wu H (eds) Proceedings of the 52nd Annual Meeting of the Association for Computational Linguistics (Volume 2: Short Papers). Association for Computational Linguistics, Baltimore, Maryland, pp 49–54. https://doi.org/10.3115/v1/P14-2009 . https://aclanthology.org/P14-2009
Dong Q, Li L, Dai D et al (2024) A survey on in-context learning. arXiv:2301.00234
Dragoni M, Federici M, Rexha A (2019) An unsupervised aspect extraction strategy for monitoring real-time reviews stream. Inf Process Manag 56(3):1103–1118. https://doi.org/10.1016/j.ipm.2018.04.010 . https://linkinghub.elsevier.com/retrieve/pii/S0306457317305174
Du C, Wang J, Sun H et al (2021) Syntax-type-aware graph convolutional networks for natural language understanding. Appl Soft Computi 102:107080. https://doi.org/10.1016/j.asoc.2021.107080 . https://linkinghub.elsevier.com/retrieve/pii/S156849462100003X
Ettaleb M, Barhoumi A, Camelin N et al (2022) Evaluation of weakly-supervised methods for aspect extraction. Proc Comput Sci 207:2688–2697. https://doi.org/10.1016/j.procs.2022.09.327 . https://linkinghub.elsevier.com/retrieve/pii/S1877050922012169
Fan F, Feng Y, Zhao D (2018) Multi-grained attention network for aspect-level sentiment classification. In: Conference on empirical methods in natural language processing. https://api.semanticscholar.org/CorpusID:53080156
Federici M, Dragoni M (2016) A knowledge-based approach for aspect-based opinion mining. In: Sack H, Dietze S, Tordai A et al (eds) Semantic web challenges, vol 641. Communications in Computer and Information Science. Springer, Cham, pp 141–152. https://doi.org/10.1007/978-3-319-46565-4_11
Fei H, Chua TS, Li C et al (2023) On the robustness of aspect-based sentiment analysis: rethinking model, data, and training. ACM Trans Inf Syst 41(2):1–32. https://doi.org/10.1145/3564281 . https://dl.acm.org/doi/10.1145/3564281
Fei H, Ren Y, Zhang Y et al (2023) Nonautoregressive encoder-decoder neural framework for end-to-end aspect-based sentiment triplet extraction. IEEE Trans Neural Netw Learn Syst 34(9):5544–5556. https://doi.org/10.1109/TNNLS.2021.3129483 . https://ieeexplore.ieee.org/document/9634849/
Fernando J, Khodra ML, Septiandri AA (2019) Aspect and opinion terms extraction using double embeddings and attention mechanism for Indonesian hotel reviews. In: 2019 International conference of advanced informatics: concepts, theory and applications (ICAICTA). IEEE, Yogyakarta, pp 1–6. https://doi.org/10.1109/ICAICTA.2019.8904124 . https://ieeexplore.ieee.org/document/8904124/
FiQA (2018) Financial opinion mining and question answering. https://sites.google.com/view/fiqa/home
Freitas C, Motta E, Milidi´u RL, et al (2014) Sparkling vampire...lol! annotating opinions in a book review corpus. New language technologies and linguistic research: a two-way Road pp 128–146. https://www.researchgate.net/publication/271836545_Sparkling_Vampire_lol_Annotating_Opinions_in_a_Book_Review_Corpus
Fukumoto F, Sugiyama H, Suzuki Y et al (2016) Exploiting guest preferences with aspect-based sentiment analysis for hotel recommendation. In: Fred A, Dietz JL, Aveiro D et al (eds) Knowledge discovery, knowledge engineering and knowledge management, vol 631. Communications in Computer and Information Science. Springer, Cham, pp 31–46. https://doi.org/10.1007/978-3-319-52758-1_3
Ganganwar V, Rajalakshmi R (2019) Implicit aspect extraction for sentiment analysis: a survey of recent approaches. Proc Comput Sci 165:485–491. https://doi.org/10.1016/j.procs.2020.01.010 . https://www.sciencedirect.com/science/article/pii/S1877050920300181 , 2nd International Conference on Recent Trends in Advanced Computing ICRTAC-DISRUP - TIV INNOVATION, 2019 November 11–12, 2019
Ganu G, Elhadad N, Marian A (2009) Beyond the stars: improving rating predictions using review text content. In: International workshop on the web and databases. https://api.semanticscholar.org/CorpusID:18345070
García-Pablos A, Cuadros M, Rigau G (2018) W2vlda: Almost unsupervised system for aspect based sentiment analysis. Expert Syst Appl 91:127–137. https://doi.org/10.1016/j.eswa.2017.08.049 . https://linkinghub.elsevier.com/retrieve/pii/S0957417417305961
Go A (2009) Twitter sentiment classification using distant supervision. CS224N Project Report, Stanford, 1-12. https://api.semanticscholar.org/CorpusID:18635269
Gojali S, Khodra ML (2016) Aspect based sentiment analysis for review rating prediction. In: 2016 International conference on advanced informatics: concepts, theory and application (ICAICTA). IEEE, Penang, pp 1–6. https://doi.org/10.1109/ICAICTA.2016.7803110 . http://ieeexplore.ieee.org/document/7803110/
Gong Z, Li B (2022) Emotional text generation with hard constraints. In: 2022 4th International conference on frontiers technology of information and computer (ICFTIC), pp 68–73. https://doi.org/10.1109/ICFTIC57696.2022.10075091 . https://ieeexplore.ieee.org/document/10075091
Gui L, He Y (2021) Understanding patient reviews with minimum supervision. Artif Intell Med 120:102160. https://doi.org/10.1016/j.artmed.2021.102160 . https://www.sciencedirect.com/science/article/pii/S0933365721001536
Gunes O (2016) Aspect term and opinion target extraction from web product reviews using semi-Markov conditional random fields with word embeddings as features. In: Proceedings of the 6th international conference on web intelligence, mining and semantics. ACM, Nìmes, pp 1–5. https://doi.org/10.1145/2912845.2936809
Guo L, Jiang S, Du W et al (2018) Recurrent neural CRF for aspect term extraction with dependency transmission. In: Zhang M, Ng V, Zhao D et al (eds) Natural language processing and Chinese computing, vol 11108. Lecture Notes in Computer Science. Springer, Cham, pp 378–390. https://doi.org/10.1007/978-3-319-99495-6_32
He R, McAuley J (2016) Ups and downs: Modeling the visual evolution of fashion trends with one-class collaborative filtering. In: Proceedings of the 25th International Conference on World Wide Web. International World Wide Web Conferences Steering Committee, Republic and Canton of Geneva, CHE, WWW ’16, p 507–517. https://doi.org/10.1145/2872427.2883037
He R, Lee WS, Ng HT et al (2019) An interactive multi-task learning network for end-to-end aspect-based sentiment analysis. In: Proceedings of the 57th annual meeting of the association for computational linguistics. Association for Computational Linguistics, Florence, pp 504–515. https://doi.org/10.18653/v1/P19-1048 . https://www.aclweb.org/anthology/P19-1048
Hoang CD, Dinh QV, Tran NH (2022) Aspect-category-opinion-sentiment extraction using generative transformer model. In: 2022 RIVF international conference on computing and communication technologies (RIVF), pp 1–6. https://doi.org/10.1109/RIVF55975.2022.10013820 . https://ieeexplore.ieee.org/document/10013820
Hoti MH, Ajdari J, Hamiti M et al (2022) Text mining, clustering and sentiment analysis: a systematic literature review. In: 2022 11th Mediterranean conference on embedded computing (MECO). IEEE, Budva, Montenegro, pp 1–6. https://doi.org/10.1109/MECO55406.2022.9797203 . https://ieeexplore.ieee.org/document/9797203/
Howard P, Ma A, Lal V et al (2022) Cross-domain aspect extraction using transformers augmented with knowledge graphs. In: Proceedings of the 31st ACM international conference on information & knowledge management. ACM, Atlanta, pp 780–790. https://doi.org/10.1145/3511808.3557275
Huan H, He Z, Xie Y et al (2022) A multi-task dual-encoder framework for aspect sentiment triplet extraction. IEEE Access 10:103187–103199. https://doi.org/10.1109/ACCESS.2022.3210180 . https://ieeexplore.ieee.org/document/9903619/
Hu M, Liu B (2004a) Mining and summarizing customer reviews. In: Proceedings of the tenth ACM SIGKDD international conference on Knowledge discovery and data mining. ACM, Seattle, pp 168–177. https://doi.org/10.1145/1014052.1014073
Hu M, Liu B (2004b) Mining opinion features in customer reviews. In: AAAI conference on artificial intelligence. https://api.semanticscholar.org/CorpusID:5724860
J AK, Trueman TE, Cambria E (2021) A convolutional stacked bidirectional LSTM with a multiplicative attention mechanism for aspect category and sentiment detection. Cogn Comput 13(6):1423–1432. https://doi.org/10.1007/s12559-021-09948-0
Jiang Q, Chen L, Xu R et al (2019) A challenge dataset and effective models for aspect-based sentiment analysis. In: Proceedings of the 2019 conference on empirical methods in natural language processing and the 9th international joint conference on natural language processing (EMNLP-IJCNLP). Association for Computational Linguistics, Hong Kong, pp 6279–6284. https://doi.org/10.18653/v1/D19-1654 . https://www.aclweb.org/anthology/D19-1654
Jo Y, Oh AH (2011) Aspect and sentiment unification model for online review analysis. In: Proceedings of the Fourth ACM International Conference on Web Search and Data Mining. Association for Computing Machinery, New York, NY, USA, WSDM ’11, p 815–824. https://doi.org/10.1145/1935826.1935932
Kang T, Kim S, Yun H et al (2022) Gated relational encoder-decoder model for target-oriented opinion word extraction. IEEE Access 10:130507–130517. https://doi.org/10.1109/ACCESS.2022.3228835 . https://ieeexplore.ieee.org/document/9982601
Kitchenham BA, Charters S (2007) Guidelines for performing systematic literature reviews in software engineering. https://www.elsevier.com/__data/promis_misc/525444systematicreviewsguide.pdf . Backup Publisher: Keele University and Durham University Joint Report
Krishnakumari K, Sivasankar E (2018) Scalable aspect-based summarization in the hadoop environment. In: Aggarwal VB, Bhatnagar V, Mishra DK (eds) Big data analytics, vol 654. Advances in Intelligent Systems and Computing. Springer Singapore, Singapore, pp 439–449. https://doi.org/10.1007/978-981-10-6620-7_42
Kumar A, Gupta D (2021) Sentiment analysis as a restricted NLP problem:. In: Pinarbasi F, Taskiran MN (eds) Advances in business information systems and analytics. IGI Global, pp 65–96. https://doi.org/10.4018/978-1-7998-4240-8.ch004 . http://services.igi-global.com/resolvedoi/resolve.aspx?doi=10.4018/978-1-7998-4240-8.ch004
Kumar A, Seth S, Gupta S et al (2022) Sentic computing for aspect-based opinion summarization using multi-head attention with feature pooled pointer generator network. Cogn Comput 14(1):130–148. https://doi.org/10.1007/s12559-021-09835-8
Lee SK, Kim JH (2023) Sener: Sentiment element named entity recognition for aspect-based sentiment analysis. In: ICASSP 2023—2023 IEEE international conference on acoustics, speech and signal processing (ICASSP), pp 1–5. https://doi.org/10.1109/ICASSP49357.2023.10095101 . https://ieeexplore.ieee.org/document/10095101
Lee C, Lee H, Kim K et al (2024) An efficient fine-tuning of generative language model for aspect-based sentiment analysis. In: 2024 IEEE international conference on consumer electronics (ICCE), pp 1–4. https://doi.org/10.1109/ICCE59016.2024.10444216 . https://ieeexplore.ieee.org/document/10444216
Lewis M, Liu Y, Goyal N et al (2020) BART: Denoising sequence-to-sequence pre-training for natural language generation, translation, and comprehension. In: Jurafsky D, Chai J, Schluter N et al (eds) Proceedings of the 58th annual meeting of the association for computational linguistics. Association for Computational Linguistics, Online, pp 7871–7880. https://doi.org/10.18653/v1/2020.acl-main.703 . https://aclanthology.org/2020.acl-main.703
Li X, Wang B, Li L et al (2020) Deep2s: Improving aspect extraction in opinion mining with deep semantic representation. IEEE Access 8:104026–104038. https://doi.org/10.1109/ACCESS.2020.2999673 . https://ieeexplore.ieee.org/document/9107147/
Li Z, Li L, Zhou A et al (2021) JTSG: A joint term-sentiment generator for aspect-based sentiment analysis. Neurocomputing 459:1–9. https://doi.org/10.1016/j.neucom.2021.06.045 . https://www.sciencedirect.com/science/article/pii/S0925231221009693
Li J, Zhao Y, Jin Z et al (2022a) SK2: Integrating implicit sentiment knowledge and explicit syntax knowledge for aspect-based sentiment analysis. In: Proceedings of the 31st ACM international conference on information & knowledge management. ACM, Atlanta, pp 1114–1123. https://doi.org/10.1145/3511808.3557452
Li Y, Lin Y, Lin Y et al (2022) A span-sharing joint extraction framework for harvesting aspect sentiment triplets. Knowl Based Syst 242:108366. https://doi.org/10.1016/j.knosys.2022.108366 . https://linkinghub.elsevier.com/retrieve/pii/S0950705122001381
Li Y, Wang C, Lin Y et al (2022) Span-based relational graph transformer network for aspect-opinion pair extraction. Knowl Inf Syst 64(5):1305–1322. https://doi.org/10.1007/s10115-022-01675-8
Li S, Zhang Y, Lan Y et al (2023) From implicit to explicit: a simple generative method for aspect-category-opinion-sentiment quadruple extraction. In: 2023 international joint conference on neural networks (IJCNN), pp 1–8. https://doi.org/10.1109/IJCNN54540.2023.10191098 . https://ieeexplore.ieee.org/document/10191098
Liang B, Su H, Gui L et al (2022) Aspect-based sentiment analysis via affective knowledge enhanced graph convolutional networks. Knowl Based Syst 235:107643. https://doi.org/10.1016/j.knosys.2021.107643 . https://linkinghub.elsevier.com/retrieve/pii/S0950705121009059
Ligthart A, Catal C, Tekinerdogan B (2021) Systematic reviews in sentiment analysis: a tertiary study. Artif Intell Rev 54(7):4997–5053. https://doi.org/10.1007/s10462-021-09973-3
Lil Z, Yang Z, Li X et al (2023) Two-stage aspect sentiment quadruple prediction based on MRC and text generation. In: 2023 IEEE International conference on systems, man, and cybernetics (SMC), pp 2118–2125. https://doi.org/10.1109/SMC53992.2023.10394369 . https://ieeexplore-ieee-org.ezproxy.auckland.ac.nz/document/10394369
Lim KW, Buntine W (2014) Twitter opinion topic model: extracting product opinions from tweets by leveraging hashtags and sentiment lexicon. In: Proceedings of the 23rd ACM international conference on conference on information and knowledge management. ACM, Shanghai, pp 1319–1328. https://doi.org/10.1145/2661829.2662005
Lin B, Cassee N, Serebrenik A et al (2022) Opinion mining for software development: a systematic literature review. ACM Trans Softw Eng Methodol 31(3):1–41. https://doi.org/10.1145/3490388
Liu Q, Gao Z, Liu B, et al (2015) Automated rule selection for aspect extraction in opinion mining. In: Proceedings of the 24th International Conference on Artificial Intelligence. AAAI Press, IJCAI' 15, p 1291–1297. https://doi.org/10.5555/2832415.2832429
Liu Y, Ott M, Goyal N et al (2019) Roberta: a robustly optimized BERT pretraining approach. arXiv:1907.11692
Liu H, Chatterjee I, Zhou M et al (2020) Aspect-based sentiment analysis: a survey of deep learning methods. IEEE Trans Comput Soc Syst 7(6):1358–1375. https://doi.org/10.1109/TCSS.2020.3033302 . https://ieeexplore.ieee.org/document/9260162/
Liu J, Chen T, Guo H et al (2024) Exploiting duality in aspect sentiment triplet extraction with sequential prompting. IEEE Trans Knowl Data Eng 1–12. https://doi.org/10.1109/TKDE.2024.3391381 . https://ieeexplore.ieee.org/document/10505831
López D, Arco L (2019) Multi-domain aspect extraction based on deep and lifelong learning. In: Nyström I, Hernández Heredia Y, Milián Núñez V (eds) Progress in pattern recognition, image analysis, computer vision, and applications, vol 11896. Lecture Notes in Computer Science. Springer, Cham, pp 556–565. https://doi.org/10.1007/978-3-030-33904-3_52
Luo H, Li T, Liu B et al (2019) Improving aspect term extraction with bidirectional dependency tree representation. IEEE/ACM Trans Audio Speech Lang Process 27(7):1201–1212. https://doi.org/10.1109/TASLP.2019.2913094 . https://ieeexplore.ieee.org/document/8698340/
Ma Y, Chen G, Wei Q (2017) Finding users preferences from large-scale online reviews for personalized recommendation. Electron Commer Res 17(1):3–29. https://doi.org/10.1007/s10660-016-9240-9
Maitama JZ, Idris N, Abdi A et al (2020) A systematic review on implicit and explicit aspect extraction in sentiment analysis. IEEE Access 8:194166–194191. https://doi.org/10.1109/ACCESS.2020.3031217 . https://ieeexplore.ieee.org/document/9234464/
Manning CD (2022) Human language understanding & reasoning. Daedalus 151(2):127–138. https://doi.org/10.1162/daed_a_01905 . https://direct.mit.edu/daed/article/151/2/127/110621/Human-Language-Understanding-amp-Reasoning
Marstawi A, Sharef NM, Aris TNM, et al (2017) Ontology-based aspect extraction for an improved sentiment analysis in summarization of product reviews. In: Proceedings of the 8th international conference on computer modeling and simulation, ICCMS’17. Association for Computing Machinery, New York, pp 100–104. https://doi.org/10.1145/3036331.3036362
McAuley J, Leskovec J, Jurafsky D (2012) Learning attitudes and attributes from multi-aspect reviews. In: Proceedings of the 2012 IEEE 12th International Conference on Data Mining. IEEE Computer Society, USA, ICDM ’12, p 1020–1025. https://doi.org/10.1109/ICDM.2012.110
McAuley J, Targett C, Shi Q, Van Den Hengel A (2015) Image-based recommendations on styles and substitutes. In Proceedings of the 38th international ACM SIGIR conference on research and development in information retrieval Association for Computing Machinery, New York, NY, USA, SIGIR'15, p 43–52. https://doi.org/10.1145/2766462.2767755
Mitchell M, Aguilar J, Wilson T, et al (2013) Open domain targeted sentiment. In: Yarowsky D, Baldwin T, Korhonen A, et al (eds) Proceedings of the 2013 Conference on Empirical Methods in Natural Language Processing. Association for Computational Linguistics, Seattle, Washington, USA, pp 1643–1654. https://aclanthology.org/D13-1171
Mughal N, Mujtaba G, Shaikh S et al (2024) Comparative analysis of deep natural networks and large language models for aspect-based sentiment analysis. IEEE Access 12:60943–60959. https://doi.org/10.1109/ACCESS.2024.3386969 . https://ieeexplore.ieee.org/document/10504711
Nawaz A, Awan AA, Ali T et al (2020) Product’s behaviour recommendations using free text: an aspect based sentiment analysis approach. Clust Comput 23(2):1267–1279. https://doi.org/10.1007/s10586-019-02995-1
Nazir A, Rao Y (2022) IAOTP: An interactive end-to-end solution for aspect-opinion term pairs extraction. In: Proceedings of the 45th international ACM SIGIR conference on research and development in information retrieval. ACM, Madrid, pp 1588–1598. https://doi.org/10.1145/3477495.3532085
Nazir A, Rao Y, Wu L et al (2022) IAF-LG: an interactive attention fusion network with local and global perspective for aspect-based sentiment analysis. IEEE Trans Affect Comput 13(4):1730–1742. https://doi.org/10.1109/TAFFC.2022.3208216 . https://ieeexplore.ieee.org/document/9896931/
Nazir A, Rao Y, Wu L et al (2022) Issues and challenges of aspect-based sentiment analysis: a comprehensive survey. IEEE Trans Affect Comput 13(2):845–863. https://doi.org/10.1109/TAFFC.2020.2970399 . https://ieeexplore.ieee.org/document/8976252/
Obiedat R, Al-Darras D, Alzaghoul E et al (2021) Arabic aspect-based sentiment analysis: a systematic literature review. IEEE Access 9:152628–152645. https://doi.org/10.1109/ACCESS.2021.3127140 . https://ieeexplore.ieee.org/document/9611271/
OpenAI (2023) Chatgpt (mar 14 version) [large language model]. https://chat.openai.com/chat
Pathan AF, Prakash C (2022) Cross-domain aspect detection and categorization using machine learning for aspect-based opinion mining. Int J Inf Manag Data Insights 2(2):100099. https://doi.org/10.1016/j.jjimei.2022.100099 . https://www.sciencedirect.com/science/article/pii/S2667096822000428
Peng H, Ma Y, Li Y, et al (2018) Learning multi-grained aspect target sequence for chinese sentiment analysis. Knowledge-Based Syst 148:167–176. https://doi.org/10.1016/j.knosys.2018.02.034 . https://www.sciencedirect.com/science/article/pii/S0950705118300972
Phan MH, Ogunbona PO (2020) Modelling context and syntactical features for aspect-based sentiment analysis. In: Proceedings of the 58th annual meeting of the association for computational linguistics. Association for Computational Linguistics, Online, pp 3211–3220. https://doi.org/10.18653/v1/2020.acl-main.293 . https://www.aclweb.org/anthology/2020.acl-main.293
Pontiki M, Galanis D, Pavlopoulos J et al (2014) SemEval-2014 task 4: aspect based sentiment analysis. In: Nakov P, Zesch T (eds) Proceedings of the 8th international workshop on semantic evaluation (SemEval 2014). Association for Computational Linguistics, Dublin, pp 27–35. https://doi.org/10.3115/v1/S14-2004 . https://aclanthology.org/S14-2004
Pontiki M, Galanis D, Papageorgiou H et al (2015) SemEval-2015 task 12: aspect based sentiment analysis. In: Nakov P, Zesch T, Cer D et al (eds) Proceedings of the 9th international workshop on semantic evaluation (SemEval 2015). Association for Computational Linguistics, Denver, pp 486–495. https://doi.org/10.18653/v1/S15-2082 . https://aclanthology.org/S15-2082
Pontiki M, Galanis D, Papageorgiou H et al (2016) SemEval-2016 task 5: aspect based sentiment analysis. In: Bethard S, Carpuat M, Cer D et al (eds) Proceedings of the 10th international workshop on semantic evaluation (SemEval-2016). Association for Computational Linguistics, San Diego, pp 19–30. https://doi.org/10.18653/v1/S16-1002 . https://aclanthology.org/S16-1002
Poria S, Chaturvedi I, Cambria E et al (2016) Sentic LDA: Improving on LDA with semantic similarity for aspect-based sentiment analysis. In: 2016 International joint conference on neural networks (IJCNN). IEEE, Vancouver, pp 4465–4473. https://doi.org/10.1109/IJCNN.2016.7727784 . http://ieeexplore.ieee.org/document/7727784/
Prather J, Becker BA, Craig M et al (2020) What do we think we think we are doing?: Metacognition and self-regulation in programming. In: Proceedings of the 2020 ACM conference on international computing education research. ACM, Virtual Event New Zealand, pp 2–13. https://doi.org/10.1145/3372782.3406263
Presannakumar K, Mohamed A (2021) An enhanced method for review mining using n-gram approaches. In: Raj JS, Iliyasu AM, Bestak R et al (eds) Innovative data communication technologies and application, vol 59. Lecture Notes on Data Engineering and Communications Technologies. Springer Singapore, Singapore, pp 615–626. https://doi.org/10.1007/978-981-15-9651-3_51
Radford A, Wu J, Child R et al (2019) Language models are unsupervised multitask learners. https://api.semanticscholar.org/CorpusID:160025533
Raffel C, Shazeer N, Roberts A et al (2020) Exploring the limits of transfer learning with a unified text-to-text transformer. J Mach Learn Res 21(1). https://dl.acm.org/doi/abs/10.5555/3455716.3455856
Rahman MA, Kumar Dey E (2018) Datasets for aspect-based sentiment analysis in bangla and its baseline evaluation. Data 3(2). https://doi.org/10.3390/data3020015 . https://www.mdpi.com/2306-5729/3/2/15
Rana TA, Cheah YN (2016) Aspect extraction in sentiment analysis: comparative analysis and survey. Artif Intell Rev 46:459–483. https://api.semanticscholar.org/CorpusID:24401592
Rani S, Kumar P (2019) A journey of Indian languages over sentiment analysis: a systematic review. Artif Intell Rev 52(2):1415–1462. https://doi.org/10.1007/s10462-018-9670-y
Ruskanda FZ, Widyantoro DH, Purwarianti A (2019) Sequential covering rule learning for language rule-based aspect extraction. In: 2019 International conference on advanced computer science and information systems (ICACSIS). IEEE, Bali, pp 229–234. https://doi.org/10.1109/ICACSIS47736.2019.8979743 . https://ieeexplore.ieee.org/document/8979743/
Sabeeh A, Dewang RK (2019) Comparison, classification and survey of aspect based sentiment analysis. In: Luhach AK, Singh D, Hsiung PA et al (eds) Advanced informatics for computing research. Springer Singapore, Singapore, pp 612–629. https://doi.org/10.1007/978-981-13-3140-4_55
Saeidi M, Bouchard G, Liakata M, et al (2016) SentiHood: Targeted aspect based sentiment analysis dataset for urban neighbourhoods. In: Matsumoto Y, Prasad R (eds) Proceedings of COLING 2016, the 26th International Conference on Computational Linguistics: Technical Papers. The COLING 2016 Organizing Committee, Osaka, Japan, pp 1546–1556. https://aclanthology.org/C16-1146
Sanders NJ (2011) Sanders-twitter sentiment corpus. Sanders Analytics LLC
Satyarthi S, Sharma S (2023) Identification of effective deep learning approaches for classifying sentiments at aspect level in different domain. In: 2023 IEEE International conference on paradigm shift in information technologies with innovative applications in global scenario (ICPSITIAGS), pp 496–508. https://doi.org/10.1109/ICPSITIAGS59213.2023.10527695 . https://ieeexplore-ieee-org.ezproxy.auckland.ac.nz/document/10527695
Sharma A, Shekhar H (2020) Intelligent learning based opinion mining model for governmental decision making. Proc Comput Sci 173:216–224. https://doi.org/10.1016/j.procs.2020.06.026 . https://linkinghub.elsevier.com/retrieve/pii/S1877050920315301
Socher R, Perelygin A, Wu J, et al (2013) Recursive deep models for semantic compositionality over a sentiment treebank. In: Yarowsky D, Baldwin T, Korhonen A, et al (eds) Proceedings of the 2013 Conference on Empirical Methods in Natural Language Processing. Association for Computational Linguistics, Seattle, Washington, USA, pp 1631–1642. https://aclanthology.org/D13-1170
Soni PK, Rambola R (2022) A survey on implicit aspect detection for sentiment analysis: terminology, issues, and scope. IEEE Access 10:63932–63957. https://doi.org/10.1109/ACCESS.2022.3183205 . https://ieeexplore.ieee.org/document/9796523
Suchrady RZ, Purwarianti A (2023) Indo LEGO-ABSA: a multitask generative aspect based sentiment analysis for Indonesian language. In: 2023 International conference on electrical engineering and informatics (ICEEI), pp 1–6. https://doi.org/10.1109/ICEEI59426.2023.10346852 . https://ieeexplore-ieee-org.ezproxy.auckland.ac.nz/document/10346852
Sutskever I, Vinyals O, Le QV (2014) Sequence to sequence learning with neural networks. In: Proceedings of the 27th international conference on neural information processing systems, NIPS’14, vol 2. MIT Press, Montreal, pp 3104–3112
Su H, Wang X, Li J et al (2024) Enhanced implicit sentiment understanding with prototype learning and demonstration for aspect-based sentiment analysis. IEEE Trans Comput Soc Syst 1–16. https://doi.org/10.1109/TCSS.2024.3368171 . https://ieeexplore-ieee-org.ezproxy.auckland.ac.nz/document/10584152
Team TPD (2023) pandas-dev/pandas: Pandas. https://doi.org/10.5281/ZENODO.3509134 . https://zenodo.org/record/3509134
Toprak C, Jakob N, Gurevych I (2010) Sentence and expression level annotation of opinions in user-generated discourse. In: Proceedings of the 48th Annual Meeting of the Association for Computational Linguistics. Association for Computational Linguistics, USA, ACL ’10, p 575–584. https://doi.org/10.5555/1858681.1858740
Tran TU, Hoang HTT, Huynh HX (2020) Bidirectional independently long short-term memory and conditional random field integrated model for aspect extraction in sentiment analysis. In: Satapathy SC, Bhateja V, Nguyen BL et al (eds) Frontiers in intelligent computing: theory and applications, vol 1014. Advances in Intelligent Systems and Computing. Springer Singapore, Singapore, pp 131–140. https://doi.org/10.1007/978-981-13-9920-6_14
Tubishat M, Idris N, Abushariah M (2021) Explicit aspects extraction in sentiment analysis using optimal rules combination. Futur Gener Comput Syst 114:448–480. https://doi.org/10.1016/j.future.2020.08.019 . https://linkinghub.elsevier.com/retrieve/pii/S0167739X1933081X
Vasanthi A, Kumar H, Karanraj R (2022) An RL approach for ABSA using transformers. In: 2022 6th International conference on trends in electronics and informatics (ICOEI), pp 354–361. https://doi.org/10.1109/ICOEI53556.2022.9776915 . https://ieeexplore.ieee.org/document/9776915
Vaswani A, Shazeer N, Parmar N, et al (2017) Attention is all you need. In: Proceedings of the 31st international conference on neural information processing systems, NIPS’17. Curran Associates Inc., Red Hook, pp 6000–6010. https://doi.org/10.5555/3295222.3295349
Wang H, Lu Y, Zhai C (2010) Latent aspect rating analysis on review text data: a rating regression approach. In: Proceedings of the 16th ACM SIGKDD International Conference on Knowledge Discovery and Data Mining. Association for Computing Machinery, New York, NY, USA, KDD ’10, p 783–792. https://doi.org/10.1145/1835804.1835903
Wang H, Lu Y, Zhai C (2011) Latent aspect rating analysis without aspect keyword supervision. In: Proceedings of the 17th ACM SIGKDD International Conference on Knowledge Discovery and Data Mining. Association for Computing Machinery, New York, NY, USA, KDD ’11, p 618–626. https://doi.org/10.1145/2020408.2020505
Wang Y, Huang Y, Wang M (2017) Aspect-based rating prediction on reviews using sentiment strength analysis. In: Benferhat S, Tabia K, Ali M (eds) Advances in artificial intelligence: from theory to practice, vol 10351. Lecture Notes in Computer Science. Springer, Cham, pp 439–447. https://doi.org/10.1007/978-3-319-60045-1_45
Wang W, Pan SJ, Dahlmeier D (2018) Memory networks for fine-grained opinion mining. Artif Intell 265:1–17. https://doi.org/10.1016/j.artint.2018.09.002 . https://linkinghub.elsevier.com/retrieve/pii/S000437021830599X
Wang J, Xu B, Zu Y (2021a) Deep learning for aspect-based sentiment analysis. In: 2021 International conference on machine learning and intelligent systems engineering (MLISE), pp 267–271. https://doi.org/10.1109/MLISE54096.2021.00056 . https://ieeexplore.ieee.org/document/9611705
Wang L, Zong B, Liu Y et al (2021b) Aspect-based sentiment classification via reinforcement learning. In: 2021 IEEE international conference on data mining (ICDM), pp 1391–1396. https://doi.org/10.1109/ICDM51629.2021.00177 . https://ieeexplore.ieee.org/document/9679112
Wang X, Liu P, Zhu Z et al (2022) Interactive double graph convolutional networks for aspect-based sentiment analysis. In: 2022 International joint conference on neural networks (IJCNN). IEEE, Padua, Italy, pp 1–7. https://doi.org/10.1109/IJCNN55064.2022.9892934 . https://ieeexplore.ieee.org/document/9892934/
Wang Z, Xia R, Yu J (2024) Unified ABSA via annotation-decoupled multi-task instruction tuning. IEEE Trans Knowl Data Eng 1–13. https://doi.org/10.1109/TKDE.2024.3392836 . https://ieeexplore.ieee.org/document/10507027
Wankhade M, Rao ACS, Kulkarni C (2022) A survey on sentiment analysis methods, applications, and challenges. Artif Intel Rev 55(7):5731–5780. https://doi.org/10.1007/s10462-022-10144-1
Wikipedia (2023) SemEval. https://en.wikipedia.org/wiki/SemEval
William, Khodra ML (2022) Generative opinion triplet extraction using pretrained language model. In: 2022 9th International conference on advanced informatics: concepts, theory and applications (ICAICTA), pp 1–6. https://doi.org/10.1109/ICAICTA56449.2022.9933004 . https://ieeexplore.ieee.org/document/9933004
Wu S, Fei H, Ren Y et al (2021) High-order pair-wise aspect and opinion terms extraction with edge-enhanced syntactic graph convolution. IEEE/ACM Trans Audio Speech Lang Process 29:2396–2406. https://doi.org/10.1109/TASLP.2021.3095672 . https://ieeexplore.ieee.org/document/9478183/
Xing X, Jin Z, Jin D et al (2020) Tasty burgers, soggy fries: probing aspect robustness in aspect-based sentiment analysis. In: Proceedings of the 2020 conference on empirical methods in natural language processing (EMNLP). Association for Computational Linguistics, Online, pp 3594–3605. https://doi.org/10.18653/v1/2020.emnlp-main.292 . https://www.aclweb.org/anthology/2020.emnlp-main.292
Xu K, Zhao H, Liu T (2020) Aspect-specific heterogeneous graph convolutional network for aspect-based sentiment classification. IEEE Access 8:139346–139355. https://doi.org/10.1109/ACCESS.2020.3012637 . https://ieeexplore.ieee.org/document/9152016/
Xu Q, Zhu L, Dai T et al (2020) Non-negative matrix factorization for implicit aspect identification. J Ambient Intell Humaniz Comput 11(7):2683–2699. https://doi.org/10.1007/s12652-019-01328-9
Yan K, Tang L, Wu M et al (2023) Aspect-based sentiment analysis method using text generation. In: Proceedings of the 2023 7th international conference on big data and internet of things, BDIOT’23. Association for Computing Machinery, New York, pp 156–161. https://doi.org/10.1145/3617695.3617709
Yauris K, Khodra ML (2017) Aspect-based summarization for game review using double propagation. In: 2017 International conference on advanced informatics, concepts, theory, and applications (ICAICTA). IEEE, Denpasar, pp 1–6. https://doi.org/10.1109/ICAICTA.2017.8090997 . http://ieeexplore.ieee.org/document/8090997/
You L, Han F, Peng J et al (2022) ASK-RoBERTa: a pretraining model for aspect-based sentiment classification via sentiment knowledge mining. Knowl Based Syst 253:109511. https://doi.org/10.1016/j.knosys.2022.109511 . https://linkinghub.elsevier.com/retrieve/pii/S0950705122007584
Yu C, Wu T, Li J et al (2023a) Syngen: A syntactic plug-and-play module for generative aspect-based sentiment analysis. In: ICASSP 2023–2023 IEEE international conference on acoustics, speech and signal processing (ICASSP), pp 1–5. https://doi.org/10.1109/ICASSP49357.2023.10094591 . https://ieeexplore.ieee.org/document/10094591
Yu Y, Zhao M, Zhou S (2023b) Boosting aspect sentiment quad prediction by data augmentation and self-training. In: 2023 International joint conference on neural networks (IJCNN), pp 1–8. https://doi.org/10.1109/IJCNN54540.2023.10191634 . https://ieeexplore.ieee.org/document/10191634
Zarindast A, Sharma A, Wood J (2021) Application of text mining in smart lighting literature—an analysis of existing literature and a research agenda. Int J Inf Manag Data Insights 1(2):100032. https://doi.org/10.1016/j.jjimei.2021.100032 . https://linkinghub.elsevier.com/retrieve/pii/S2667096821000252
Zhang Y, Xu B, Zhao T (2020) Convolutional multi-head self-attention on memory for aspect sentiment classification. IEEE/CAA J Automatica Sinica 7(4):1038–1044. https://doi.org/10.1109/JAS.2020.1003243 . https://ieeexplore.ieee.org/document/9128078/
Zhang W, Deng Y, Li X et al (2021a) Aspect sentiment quad prediction as paraphrase generation. In: Moens MF, Huang X, Specia L et al (eds) Proceedings of the 2021 conference on empirical methods in natural language processing. Association for Computational Linguistics, Online and Punta Cana, Dominican Republic, pp 9209–9219. https://doi.org/10.18653/v1/2021.emnlp-main.726 . https://aclanthology.org/2021.emnlp-main.726
Zhang W, Li X, Deng Y et al (2021b) Towards generative aspect-based sentiment analysis. In: Zong C, Xia F, Li W et al (eds) Proceedings of the 59th annual meeting of the association for computational linguistics and the 11th international joint conference on natural language processing (volume 2: short papers). Association for Computational Linguistics, Online, pp 504–510. https://doi.org/10.18653/v1/2021.acl-short.64 . https://aclanthology.org/2021.acl-short.64
Zhang H, Chen Z, Chen B et al (2022) Complete quadruple extraction using a two-stage neural model for aspect-based sentiment analysis. Neurocomputing 492:452–463. https://doi.org/10.1016/j.neucom.2022.04.027 . https://www.sciencedirect.com/science/article/pii/S0925231222003939
Zhang W, Li X, Deng Y et al (2022b) A survey on aspect-based sentiment analysis: tasks, methods, and challenges. arXiv:2203.01054
Zhang W, Li X, Deng Y et al (2022) A survey on aspect-based sentiment analysis: tasks, methods, and challenges. IEEE Trans on Knowl and Data Eng 35(11):11019–11038. https://doi.org/10.1109/TKDE.2022.3230975
Zhang X, Xu J, Cai Y et al (2023) Detecting dependency-related sentiment features for aspect-level sentiment classification. IEEE Trans Affect Comput 14(1):196–210. https://doi.org/10.1109/TAFFC.2021.3063259 . https://ieeexplore.ieee.org/document/9368987/
Zhang W, Zhang X, Cui S, et al (2024a) Adaptive data augmentation for aspect sentiment quad prediction. In: ICASSP 2024—2024 IEEE international conference on acoustics, speech and signal processing (ICASSP), pp 11176–11180. https://doi.org/10.1109/ICASSP48485.2024.10447700
Zhang W, Zhang X, Cui S et al (2024b) Adaptive data augmentation for aspect sentiment quad prediction. In: ICASSP 2024—2024 IEEE international conference on acoustics, speech and signal processing (ICASSP), pp 11176–11180. https://doi.org/10.1109/ICASSP48485.2024.10447700 . https://ieeexplore-ieee-org.ezproxy.auckland.ac.nz/document/10447700
Zhao H, Yang M, Bai X et al (2024) A survey on multimodal aspect-based sentiment analysis. IEEE Access 12:12039–12052. https://doi.org/10.1109/ACCESS.2024.3354844 . https://ieeexplore.ieee.org/document/10401113
Zhou J, Huang JX, Chen Q et al (2019) Deep learning for aspect-level sentiment classification: survey, vision, and challenges. IEEE Access 7:78454–78483. https://doi.org/10.1109/ACCESS.2019.2920075 . https://ieeexplore.ieee.org/document/8726353
Zhou C, Wu Z, Song D et al (2024) Span-pair interaction and tagging for dialogue-level aspect-based sentiment quadruple analysis. In: Proceedings of the ACM on web conference 2024, WWW’24. Association for Computing Machinery, New York, pp 3995–4005. https://doi.org/10.1145/3589334.3645355
Download references
Open Access funding enabled and organized by CAUL and its Member Institutions.
Author information
Authors and affiliations.
School of Computer Science, The University of Auckland, Auckland, New Zealand
Yan Cathy Hua, Paul Denny, Jörg Wicker & Katerina Taskova
You can also search for this author in PubMed Google Scholar
Contributions
Y. C. H designed, conducted, and wrote this review. P. D., J. W., and K. T. guided the design of the review methodology and review protocol, and reviewed and provided feedback on the manuscript.
Corresponding author
Correspondence to Yan Cathy Hua .
Ethics declarations
Conflict of interest.
The authors have no Conflict of interest as defined by Springer, or other interests that might be perceived to influence the results and/or discussion reported in this paper.
Additional information
Publisher's note.
Springer Nature remains neutral with regard to jurisdictional claims in published maps and institutional affiliations.
Appendix A: Aspect-based sentiment analysis (ABSA)
1.1 appendix a.1: definition and examples.
Aspect-based sentiment analysis (ABSA) is a sub-domain of fine-grained SA (Nazir et al 2022a ). ABSA focuses on identifying the sentiments towards specific entities or their attributes/ features called aspects (Nazir et al 2022a ; Akhtar et al 2020 ). An aspect can be explicitly expressed in the text ( explicit aspect ) or absent from the text but implied from the context ( implicit aspects ) (Maitama et al 2020 ; Xu et al 2020b ). Moreover, the aspect-level sentiment could differ across aspects and be different from the overall sentiment of the sentence or the document (e.g. Akhtar et al 2020 , 2017 ; Li et al 2022a ). Some studies further distinguish aspect into aspect term and aspect category , with the former referring to the aspect expression in the input text (e.g. “pizza”), and the latter a latent construct that is usually a high-level category across aspect terms (e.g. “food”) that are either identified or given (Chauhan et al 2019 ; Akhtar et al 2018 ).
The following examples illustrate the ABSA terminologies:
(From a restaurant review Footnote 7 ): “ The restaurant was expensive, but the menu was great. ” This sentence has one explicit aspect “menu” (sentiment term: “great”, sentiment polarity: positive), one implicit aspect “price” (sentiment term: “expensive”, sentiment polarity: negative). Depending on the target/given categories, the aspects can be further classified into categories, such as “menu” into “general” and “price” into “price”.
(From a laptop review Footnote 8 ): “ It is extremely portable and easily connects to WIFI at the library and elsewhere. ” This sentence has two implicit aspects: “portability” (sentiment term: “portable”, sentiment polarity: positive), “connectivity” (sentiment term: “easily”, sentiment polarity: positive). The aspects can be further classified into categories, such as both under “laptop” (as opposed to “software” or “support”).
(Text from a course review): “ It was too difficult and had an insane amount of work, I wouldn’t recommend it to new students even though the tutorial and the lecturer were really helpful .” The two explicit aspects in Example 3 are “tutorial” and “lecturer” (sentiment terms: “helpful”, polarities: positive). The implicit aspects are “content” (sentiment term: “too difficult”, sentiment polarity: negative), “workload” (sentiment term: “insane amount”, sentiment polarity: negative), and “course” (sentiment term: “would not recommend”, sentiment polarity: negative). An illustration of aspect categories would be assigning the aspect “lecturer” to the more general category “staff” and “tutorial” to the category “course component”.
As demonstrated above, the fine granularity makes ABSA more targetable and informative than document- or sentence-level SA. Thus, ABSA can precede downstream applications such as attribute weighting in overall review ratings (e.g. Da’u et al 2020 ), aspect-based opinion summarisation (e.g. Yauris and Khodra 2017 ; Kumar et al 2022 ; Almatrafi and Johri 2022 ), and automated personalised recommendation systems (e.g. Ma et al 2017 ; Nawaz et al 2020 ).
Compared with document- or sentence-level SA, while being the most detailed and informative, ABSA is also the most complex and challenging (Huan et al 2022 ). The most noticeable challenges include the number of ABSA subtasks, their interrelations and context dependencies, and the generalisability of solutions across topic domains.
1.2 Appendix A.2: ABSA Subtasks
A full ABSA solution has more subtasks than coarser-grained SA. The most fundamental ones (Li et al 2022a ; Huan et al 2022 ; Li et al 2020 ; Fei et al 2023b ) include:
Aspect (term) extraction/identification (AE) , which has a slight variation in meaning depending on the overall ABSA approach. Some authors (e.g. (Zhang et al 2023 ; Luo et al 2019 ; Ruskanda et al 2019 )) consider AE as identifying the attribute or entity that is the target of an opinion expressed in the text and sometimes call it “opinion target extraction” (Guo et al 2018 ). In these cases, opinion terms were often identified in order to find their target aspect terms. Others (e.g. Akhtar et al 2020 ; Gunes 2016 ; Li et al 2020 ; Ettaleb et al 2022 ; Tran et al 2020 ) define AE as identifying the key or all attributes of entities mentioned in the text. Implicit-Aspect Extraction (IAE) is often mentioned as a task by itself due to its technical challenge.
Opinion (term) Extraction/Identification (OE) , which relates to identifying the “opinion terms” or the sentiment expression of a specific entity/aspect (e.g. Li et al 2022a ; Wang et al 2018 ; Fernando et al 2019 ; Fei et al 2023b ). In Example 1 above, an OE task would extract the sentiment terms “great” (associated with the aspect term “menu”) and “expensive” (associated with the implicit aspect “price”).
Aspect-Sentiment Classification (ASC) , which refers to obtaining the sentiment polarity category (e.g. negative, neutral, positive, conflict) or sentiment score (e.g. 1 to 5 or \(-1\) to 1 along the scale from negative to positive) associated with a given aspect or aspect category (e.g. Akhtar et al 2020 ; Gojali and Khodra 2016 ; Castellanos et al 2011 ). This is often done via evaluating the associated opinion term(s), and sentiment lexicon resources such as the SentiWordNet (Baccianella et al 2010 ) and SenticNet (Cambria et al 2016 ) can be used to assign polarity scores (Gojali and Khodra 2016 ). Sentiment scores can be further aggregated across opinion terms for the same aspect, or across aspect terms to generate higher-level ratings, such as aspect-category ratings within or across documents (Gojali and Khodra 2016 ; Castellanos et al 2011 ).
As an extension of AE, some studies also involve Aspect-Category Detection (ACD) and Aspect Category Sentiment Analysis (ACSA) when the focus of sentiment analysis is on (often pre-defined) latent topics or concepts and requires classifying aspect terms into categories (Pathan and Prakash 2022 ).
Traditional full ABSA solutions often perform the subtasks in a pipeline manner (Li et al 2022b ; Nazir and Rao 2022 ) using one or more of the linguistic (e.g. lexicons, syntactic rules, dependency relations), statistical (e.g. n-gram, Hidden Markov Model (HMM)), and machine-learning approaches (Maitama et al 2020 ; Cortis and Davis 2021 ; Federici and Dragoni 2016 ). For instance, for AE and OE, some studies used linguistic rules and sentiment lexicons to first identify opinion terms and then the associated aspect terms of each opinion term, or vice versa (e.g. You et al 2022 ; Cavalcanti and Prudêncio 2017 ), and then moved on to ASC or ACD using a supervised model or unsupervised clustering and/or ontology (Nawaz et al 2020 ; Gojali and Khodra 2016 ). Hybrid approaches are common given the task combinations in a pipeline.
With the rise of multi-task learning and deep learning (Chen et al 2022 ), an increasing number of studies explore ABSA under an End-to-end (E2E) framework that performs multiple fundamental ABSA subtasks in one model to better capture the inter-task relations (Liu et al 2024 ), and some combine them into a single composite task (Huan et al 2022 ; Li et al 2022b ; Zhang et al 2022b ). These composite tasks are most commonly formulated as a sequence- or span-based tagging problem (Huan et al 2022 ; Li et al 2022b ; Nazir and Rao 2022 ). The most common composite tasks are: Aspect-Opinion Pair Extraction (AOPE) , which directly outputs {aspect, opinion} pairs from text input (Nazir and Rao 2022 ; Li et al 2022c ; Wu et al 2021 ) such as “ \(\langle \) menu, great \(\rangle \) ” from Example 1; Aspect-Polarity Co-Extraction (APCE) (Huan et al 2022 ; He et al 2019 ), which outputs {aspect, sentiment polarity} pairs such as “ \(\langle \) menu, positive \(\rangle \) ”; Aspect-Sentiment Triplet Extraction (ASTE) (Huan et al 2022 ; Li et al 2022b ; Du et al 2021 ; Fei et al 2023b ), which outputs {aspect, opinion, sentiment category} triplets, such as “ \(\langle \) menu, great, positive \(\rangle \) ”; and Aspect-Sentiment Quadruplet Extraction/Prediction (ASQE/ASQP) (Zhang et al 2022a ; Lim and Buntine 2014 ; Zhang et al 2021a , 2024a ) that outputs {aspect, opinion, aspect category, sentiment category} quadruplets, such as “ \(\langle \) menu, great, general, positive \(\rangle \) ”.
1.3 Appendix A.3: Other ABSA reviews
As this review focuses on trends instead of detailed solutions and methodologies, we refer interested readers to existing review papers that provide comprehensive and in-depth summaries of common ABSA subtask solutions and approaches, for example:
Explicit and implicit AE : Rana and Cheah ( 2016 ), Ganganwar and Rajalakshmi ( 2019 ), Soni and Rambola ( 2022 ), Maitama et al ( 2020 ).
Deep learning (DL) methods for ABSA : Do et al ( 2019 ), Liu et al ( 2020 ), Wang et al ( 2021a ), Chen and Fnu ( 2022 ), Zhang et al ( 2022c ), Mughal et al ( 2024 ). Specifically:
DL methods for ASC : Zhou et al ( 2019 ), Satyarthi and Sharma ( 2023 ).
E2E ABSA, composite tasks, and pre-trained Large Language Models (LLMs) in ABSA : Zhang et al ( 2022c ) provided a comprehensive review and shared extensive reading lists and dataset resource links via https://github.com/IsakZhang/ABSA-Survey . Mughal et al ( 2024 ) introduced common benchmark datasets, including more challenging ones for composite ABSA tasks. They also reviewed and tested the ABSA task performance of representative RNN-based models and pre-trained LLMs.
Multimodal ABSA : Zhao et al ( 2024 ).
Appendix B: Full SLR methodology
This section provides a complete, detailed description of the SLR methodology and procedures.
1.1 Appendix B.1: Research identification
To obtain the files for review, we conducted database searches between 24–25 October 2022, when we manually queried and exported a total of 4191 research papers’ PDF and BibTeX (or the equivalent) files via the web interfaces of four databases. Table 9 details the search string, search criteria, and the PDF files exported from each database.
Given the limited search parameters allowed in these digital databases, we adopted a “search broad and filter later” strategy. These database search strings were selected based on pilot trials to capture the ABSA topic name, the relatively prevalent yet unique ABSA subtask term (“extraction”), and the interchangeable use between ABSA and opinion mining; while avoiding generating false positives from the highly active, broader field of SA. The “filter later” step was carried out during the “selection of primary studies” stage introduced in the next section, which aimed at excluding cases where the keywords are only mentioned in the reference list or sparsely mentioned as a side context, and opinion mining studies that were at document or sentence levels.
1.2 Appendix B.2: Selection of primary studies
After obtaining the 4191 initial search results, we conducted a pilot manual file examination of 100 files to refine the pre-defined inclusion and exclusion criteria. We found that some search results only contained the search keywords in the reference list or Appendix, which was also reported in Prather et al ( 2020 ). In addition, there are a number of papers that only mentioned ABSA-specific keywords in their literature review or introduction sections, and the studies themselves were on coarser-grained sentiment analysis or opinion mining. Lastly, there were instances of very short research reports that provided insufficient details of the primary studies. Informed by these observations, we refined our inclusion and exclusion criteria to those in Table 1 in Sect. 3 . Note that we did not include popularity criteria such as citation numbers so we can better identify novel practices and avoid mainstream method over-dominance introduced by the citation chain (Chu and Evans 2021 ).
To implement the inclusion and exclusion criteria, we first applied PDF mining to automatically exclude files that meet the exclusion criteria, and then refined the selection with manual screening under the exclusion and inclusion criteria. Both of these processes are detailed below. Our PDF mining for automatic review screening code is also available at https://doi.org/10.5281/zenodo.12872948 .
The automatic screening consists of a pipeline with two Python packages: Pandas (Team 2023 ) and PyMuPDF. Footnote 9 We first used Pandas to extract into a dataframe (i.e. table) all exported papers’ file locations and key BibTex or equivalent information including title, year, page number, DOI, and ISBN. Next, we used PyMuPDF to iterate through each PDF file and add to the dataframe multiple data fields: whether the file was successfully decoded Footnote 10 for text extraction (if marked unsuccessful, the file was marked for manual screening), the occurrence count of each Regex keyword pattern listed below, and whether each keyword occurs after the section headings that fit into Regex patterns that represent variations of “references” and “bibliography” (referred to as “non-target sections” below). We then marked the files for exclusion by evaluating the eight criteria listed under “Auto-excluded” in Table 10 against the information recorded in the dataframe. Each of the auto-exclusion results from Steps 1–4 and 7 in Table 10 were manually checked, and those under Steps 5, 6, and 8 were spot-checked. These steps excluded 3277 out of the 4194 exported files.
Below are the regex patterns used for automatic keyword extraction and occurrence calculation:
PDF search keyword Regex list: [’absa’, ’aspect \(\backslash \) W+base \(\backslash \) w*’, ’aspect \(\backslash \) W+extrac \(\backslash \) w*’, ’aspect \(\backslash \) W+term \(\backslash \) w*’, ’aspect \(\backslash \) W+level \(\backslash \) w*’, ’term \(\backslash \) W+level’, ’sentiment \(\backslash \) W+analysis’, ’opinion \(\backslash \) W+mining’]
For the 914 files filtered through the auto-exclusion process, we manually screened them individually according to the inclusion and exclusion criteria. As shown in the second half of Table 10 , this final screening step refined the review scope to 519 papers.
1.3 Appendix B.3: Data extraction and synthesis
In the final step of the SLR, we manually reviewed each of the 519 in-scope publications and recorded information according to a pre-designed data extraction form. The key information recorded includes each study’s research focus, research application domain (“research domain” below), ABSA subtasks involved, name or description of all the datasets directly used, model name (for machine-learning solutions), architecture, whether a certain approach or paradigm is present in the study (e.g. supervised learning, deep learning, end-to-end framework, ontology, rule-based, syntactic-components), and the specific approach used (e.g. attention mechanism, Naïve Bayes classifier) under the deep learning and traditional machine learning categories.
After the data extraction, we performed data cleaning to identify and fix recording errors and inconsistencies, such as data entry typos and naming variations of the same dataset across studies. Then we created two mappings for the research and dataset domains described below.
For each reviewed study, its research domain was defaulted to “non-specific” unless the study mentioned a specific application domain or use case as its motivation, in which case that domain description was recorded instead.
The dataset domain was recorded and processed at the individual dataset level, as many reviewed studies used multiple datasets. We standardised the recorded dataset names, checked and verified the recorded dataset domain descriptions provided by the authors or the source web-pages, and then manually categorised each domain description into a domain category. For published/well-known datasets, we unified the recorded naming variations and checked the original datasets or their descriptions to verify the domain descriptions. For datasets created (e.g. web-crawled) by the authors of the reviewed studies, we named them following the “[source] [domain] (original)” format, e.g. “Yelp restaurant review (original) ”, or “Twitter (original)” if there was no distinct domain, and did not differentiate among the same-name variations. In all of the above cases, if a dataset was not created with a specific domain filter (e.g. general Twitter tweets), then it was classified as “non-specific”.
The recorded research and dataset domain descriptions were then manually grouped into 19 common domain categories. We tried to maintain consistency between the research and dataset domain categories. The following are two examples of possible mapping outcomes:
A study on a full ABSA solution without mentioning a specific application domain and using Yelp restaurant review and Amazon product review datasets would be assigned a research domain of “non-specific” and a dataset domain of “product/service review”.
A study mentioning “helping companies improve product design based on customer reviews” as the motivation would have a research domain of “product/service review”, and if they used a product review dataset and Twitter tweets crawled without filtering, the dataset domains would be “product/service review” and “non-specific”.
After applying the above-mentioned standardisation and mappings, we analysed the synthesised data quantitatively using the Pandas (Team 2023 ) library to obtain an overview of the reviewed studies and explore the answers to our RQs.
Appendix C: Additional results
See Figs. 9 , 10 and Tables 11 , 12 , 13 , 14 , 15 .
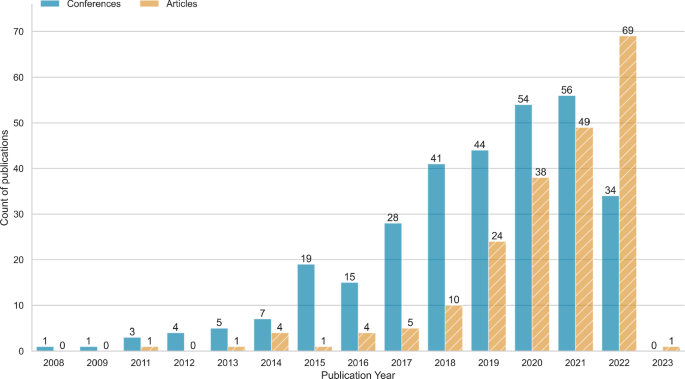
Number of included studies by publication year and type ( \(\textrm{N}=519\) ). Note Although our original search scope included journal articles, conference papers, newsletters, and magazine articles, the final 519 in-scope studies consist of only journal articles and conference papers. Conference papers noticeably outnumbered journal articles in all years until 2022, with the gap closing since 2016. We think this trend could be due to multiple factors, such as the fact that our search was conducted in late October 2022 when some conference publications were still not available; the publication lag for journal articles due to a longer processing period; and potentially a change in publication channels that is outside the scope of this review

Number of included studies with the top 5 dataset languages by publication year
Rights and permissions
Open Access This article is licensed under a Creative Commons Attribution 4.0 International License, which permits use, sharing, adaptation, distribution and reproduction in any medium or format, as long as you give appropriate credit to the original author(s) and the source, provide a link to the Creative Commons licence, and indicate if changes were made. The images or other third party material in this article are included in the article's Creative Commons licence, unless indicated otherwise in a credit line to the material. If material is not included in the article's Creative Commons licence and your intended use is not permitted by statutory regulation or exceeds the permitted use, you will need to obtain permission directly from the copyright holder. To view a copy of this licence, visit http://creativecommons.org/licenses/by/4.0/ .
Reprints and permissions
About this article
Hua, Y.C., Denny, P., Wicker, J. et al. A systematic review of aspect-based sentiment analysis: domains, methods, and trends. Artif Intell Rev 57 , 296 (2024). https://doi.org/10.1007/s10462-024-10906-z
Download citation
Accepted : 06 August 2024
Published : 17 September 2024
DOI : https://doi.org/10.1007/s10462-024-10906-z
Share this article
Anyone you share the following link with will be able to read this content:
Sorry, a shareable link is not currently available for this article.
Provided by the Springer Nature SharedIt content-sharing initiative
- Aspect-based sentiment analysis
- Systematic literature review
- Natural language processing
- Find a journal
- Publish with us
- Track your research
ORIGINAL RESEARCH article
From personality types to social labels: the impact of using mbti on social anxiety among chinese youth.
- School of Journalism and Communication, Shanghai International Studies University, Shanghai, China
Introduction: As the Myers-Briggs Type Indicator (MBTI) gains popularity among Chinese young people, it has undergone a gradual transition from being perceived as a personality assessment tool to being regarded as a social label. The objective of this study was to ascertain whether the use of the MBTI as a social label has an impact on social anxiety among Chinese youth groups.
Methods: A questionnaire survey was conducted on social media platforms to recruit Chinese youth aged 18 to 35. A total of 247 males and 222 females participated in the study, and the data was analyzed quantitatively using SPSS software and the Process macro plugin.
Results: The study found no strong correlation between MBTI as a social label and social anxiety. Moreover, this study introduced ego identity, belonging, and impression management as mediating variables and found that, under the influence of ego identity and impression management, the use of MBTI has a significant impact on social anxiety.
Discussion: The research reveals the complex role of MBTI among Chinese youth and provides a new perspective for understanding the impact of online social labels on the mental health of youth groups. Of course, this study also has limitations in terms of sample size and variable control. Future research should expand the sample size, introduce more potential influencing factors, and further validate and expand the existing conclusions.
1 Introduction
The Myers-Briggs Type Indicator (MBTI) is a measurement tool for psychological type theory proposed and developed by Isabel Briggs Myers and her mother Katharine Cook Briggs. It has gained widespread international influence and application prospects, particularly in areas such as leadership potential ( Pestana and Codina, 2019 ; Pestana and Codina, 2020 ), management behavior ( Furnham and Stringfield, 1993 ; Wu et al., 2011 ), and career planning ( McCaulley and Martin, 1995 ). The design of MBTI is primarily based on the psychological type theory proposed by Jung (1971) , who believed that people’s psychological tendencies can be divided into two basic attitudes: extraverted [E] and introverted [I], which further extend to four psychological functions, namely thinking [T], feeling [F], sensing [S], and intuition [N]. Twenty years after Jung proposed the psychological type theory, Myers and Briggs introduced the fourth dimension: judging [J] and perceiving [P] ( Quenk, 2009 ). The MBTI test separates human personality types into four dimensions and ultimately combines them into 16 personality types.
Although the MBTI still has limitations in psychological measurement aspects such as reliability and usefulness ( Boyle, 1995 ), it remains popular among Chinese youth. As of December 16, 2023, the real-time popularity of MBTI-related topics on the Xiaohongshu app (China’s version of Instagram) in the past 180 days has reached 31.2817 million. Discussions on this topic mainly revolve around interpersonal communication, indicating that MBTI has transformed from a personality type test to a means of interpersonal interaction. Recent studies have also found that some researchers have begun to focus on the importance of MBTI personality types in online social activities ( Zhang, 2024 ) and how the spread of MBTI has become a social culture ( Wang et al., 2024 ). However, their research lacks a quantitative perspective to explain this issue. With the popularity of online social media, people can communicate with others through social software (such as Twitter, Facebook, Instagram) anytime and anywhere without leaving their homes. However, as the frequency of social media use increases, people are gradually experiencing more intense social anxiety ( Yang et al., 2023 ).
Therefore, this study, using MBTI as an entry point, will explore its impact on social anxiety among Chinese youth through a survey method. Additionally, the use of MBTI may promote ego identity and further trigger group identity, which will also affect impression management made by individuals to conform to MBTI traits ( Zhang, 2024 ). Therefore, this study will also explore “ego identity,” “belonging” and “impression management” as mediating variables.
2 Literature review
2.1 using mbti as a social label and social anxiety.
Social Anxiety (SA) differs from general anxiety symptoms; it primarily occurs during interpersonal interactions and is characterized by intense fear of others’ evaluations in social situations ( Morrison and Heimberg, 2013 ; Counsell et al., 2017 ). When social anxiety escalates to a certain degree, it can lead to Social Anxiety Disorder (SAD). People with SAD often feel shy when encountering strangers and may want to withdraw from unfamiliar social environments. They are often ambivalent in nature, desiring social interaction while avoiding it due to fears of being disliked, perceived as stupid, or boring ( Stein and Stein, 2008 ). With China’s economic, cultural, and social development, social anxiety among Chinese youth is also increasing ( Xin et al., 2022 ). Some scholars argue that urbanization and employment pressures have, to some extent, reduced interpersonal connections, disrupting China’s traditional acquaintance society and thereby increasing the likelihood of social anxiety ( Chi and Xin, 2020 ; Xin et al., 2021 ).
The internet has become a crucial platform for interpersonal communication, and posting daily updates online has become a common habit among contemporary Chinese youth. Among these practices, the use of MBTI is gradually replacing the previous 12 astrological types, becoming an important social label for Chinese youth on the internet. There is a difference between social tags and social labels on the internet. Social tags mainly emphasize the technical aspects of social media, fulfilling various user needs such as information classification, search and navigation, and information extraction ( Suchanek et al., 2008 ). However, as social media evolves, more people are starting to label themselves and others, referred to as social labels. Social label theory focuses on the role of these labels in criminal and deviant behavior; once labeled, individuals are prone to eliciting negative stereotypes from themselves and others ( Becker, 1963 ). Social label theory emphasizes how societal environments, by defining or stereotyping individuals as deviants, can trigger a series of deviant behaviors ( Bernburg, 2009 ). In other words, labels are essentially constructs of social norms; they do not inherently exist ( Becker, 1963 ). In social label theory, once labeled as a norm-breaker, individuals are tagged as “deviants.” In practice, MBTI personality types also serve as a form of social label; when individuals exhibit personality traits differing from their MBTI type, they may be labeled as “deviants” by the MBTI-using community, leading to gradual isolation. This intensification may increase social anxiety as they are tagged with undesirable labels ( Stein and Stein, 2008 ). Therefore, to embody their MBTI personality type, individuals must conform to behaviors aligned with this type, driving themselves to integrate into the group and gain acceptance. It can be argued that to cater to group needs, individuals may experience social anxiety. However, this theoretical derivation remains untested. To fill this gap, the study attempts to validate this derivation. Thus, the first hypothesis of the study is:
H1 : Using MBTI as a social label positively influences social anxiety.
2.2 Using MBTI as a social label, ego identity, and social anxiety
The concept of ego identity was proposed by Erikson (1956) , who discussed the formation of self-identity within the framework of personality development. Although Erikson did not provide a clear definition of ego identity, it is generally understood that it needs to be discussed within a psychoanalytic perspective ( Bourne, 1978 ). The formation of ego identity is a crucial aspect of personality development. To further measure it, Marcia (1966) proposed the dimensions of exploration and commitment, categorizing ego identity into four types based on whether exploration leads to commitment: achievement, moratorium, foreclosure, and diffusion. Marcia’s theory provided the possibility for empirical research on Erikson’s ego identity theory, leading to the development of many semi-structured interview techniques and standardized measurement techniques, and transitioning Erikson’s identity theory into a clearly verifiable theory. Previous research has demonstrated a positive correlation between using MBTI and ego identity ( Hua and Zhou, 2023 ), suggesting that external personality information can influence ego identity to some extent. When MBTI serves as a social label, it provides a clear symbol for achieving ego identity. These symbols from the external world enable individuals to better achieve ego identity ( Brown, 1998 ).
In the context of the internet, there is research on the relationship between ego identity and social anxiety. The findings reveal that for male youth, there is a positive correlation between immature identity status, frequent internet use, and social anxiety, while no such relationship is found for female youth ( Mazalin and Moore, 2004 ). Once a mature identity status is achieved through MBTI among youth, is there still a positive correlation with social anxiety? Perhaps achieving ego identity through MBTI may reduce social anxiety, but if confined within the MBTI social label, will ego identity still reduce social anxiety? Based on theoretical derivations and the emergence of new questions, the study suggests the following hypotheses:
H2: Using MBTI as a social label positively influences ego identity.
H3: Ego identity achieved through MBTI positively influences social anxiety.
H4: Ego identity plays a mediating role between using MBTI as a social label and social anxiety.
2.3 Using MBTI as a social label, belonging, and social anxiety
Belonging is considered a subjective sense of value and respect, established on the basis of shared experiences, beliefs, or personal characteristics ( Mahar et al., 2013 ). Belonging is primarily understood from the perspective of interpersonal relationships as an important measure of whether there is a lack of mental health ( Anant, 1966 ). When belonging is lacking, it is likely to trigger anxiety in individuals ( Baumeister and Tice, 1990 ). Belonging, as a universal human need, serves to establish bonds with others. Achieving belonging involves two criteria: the need for frequent and enjoyable interactions with a few others and the requirement for these interactions to occur within a temporarily stable, enduring, and mutually beneficial emotional framework ( Baumeister and Leary, 1995 ). Using MBTI as a social label has the potential to enhance belonging, primarily due to MBTI’s classification function, which divides people into 16 personality types. Classification helps individuals understand their social environment and plays a positive role in guiding individual behavior within it ( Bruner, 1957 ; Bodenhausen et al., 2003 ). Classification also facilitates understanding the relationships between one’s own group and other groups and promotes the search for shared values among groups ( Galinsky et al., 2003 ). Through MBTI personality types, people can more easily distinguish themselves from others and ultimately achieve group categorization based on personality types. Because this categorization is based on personality types, there is similarity or proximity among group members, which helps people establish a bond and form relatively stable and enjoyable interactions ( Baumeister and Leary, 1995 ). Furthermore, classification defines a common social identity for individuals, which helps foster a sense of belonging and unity, promoting trust and cooperation among people ( Claridge, 2020 ). Groups formed by shared identities prevent individuals from being isolated, but this also means that individuals must commit to group expectations and obligations, strive to serve group interests, and avoid actions that undermine group goals ( Ellemers et al., 2002 ; Claridge, 2020 ). Therefore, individuals who use MBTI as a social label are compelled to behave in accordance with group expectations and obligations to avoid being detached from the group, which indirectly leads to social anxiety. It can be argued that while belonging may alleviate social anxiety to some extent, when assigned a social label, this belonging may lead to deeper social anxiety. To further validate the above theoretical derivation, the study proposes the following hypotheses:
H5: Using MBTI as a social label positively affects belonging.
H6: The belonging obtained from using MBTI positively affects social anxiety.
H7: This belonging plays a mediating role between using MBTI as a social label and social anxiety.
2.4 Using MBTI as a social label, impression management, and social anxiety
Humans have a universal and ongoing concern about self-presentation, sometimes acting to leave a particular impression on others ( Leary, 1995 ). In interpersonal relationships, impression management controls the formation of others’ impressions by creating an image that others expect to see Leary and Kowalski (1990) . Through interpersonal interactions, impression management can establish, maintain, or refine an individual’s image in the minds of others ( Tedeschi, 2013 ). Impression management consists of two processes: the motivation for impression management and impression construction. The former emphasizes the extent to which people want to control others’ perceptions of them, while the latter refers to the type of impression people try to construct ( Leary and Kowalski, 1990 ). There are mainly five strategies of impression management: ingratiation, self-promotion, exemplification, supplication and intimidation, which may not necessarily yield positive effects ( Jones and Pittman, 1982 ; Turnley and Bolino, 2001 ). When MBTI is used as a social label, it is likely to generate motivation for impression management during interpersonal interactions ( Zhang, 2024 ). The motivation for impression management arises from the goal relevance of the impression, the value of the desired goal, and the discrepancy between the desired image and the current image ( Leary and Kowalski, 1990 ). Past understandings of impression management were often based on the need to deceive others or meet social expectations, but research has confirmed that impression management in social interactions is not for deception but rather self-control oriented towards interpersonal relationships ( Uziel, 2010 , 2014 ). Previous studies have shown that individuals with high levels of social anxiety tend to have stronger self-control abilities during interactions with others ( Kashdan et al., 2011 ). When self-control weakens during or after social interactions, it is positively correlated with social anxiety ( Kashdan et al., 2011 ; BlaCkhart et al., 2015 ). If individual self-control is for the sake of impression management, it will bring additional burdens to social anxiety ( Finkel et al., 2006 ; BlaCkhart et al., 2015 ). However, when focusing on MBTI as a social label, is it also the case that self-control in impression management, within the context of interpersonal relationships, mediates social anxiety? This question remains unclear. Therefore, the study proposes the following hypotheses:
H8: Using MBTI as a social label positively affects self-control in impression management.
H9: The self-control in impression management obtained from using MBTI positively affects social anxiety.
H10: This self-control in impression management plays a mediating role between using MBTI as a social label and social anxiety.
See Figure 1 for the research model diagram.
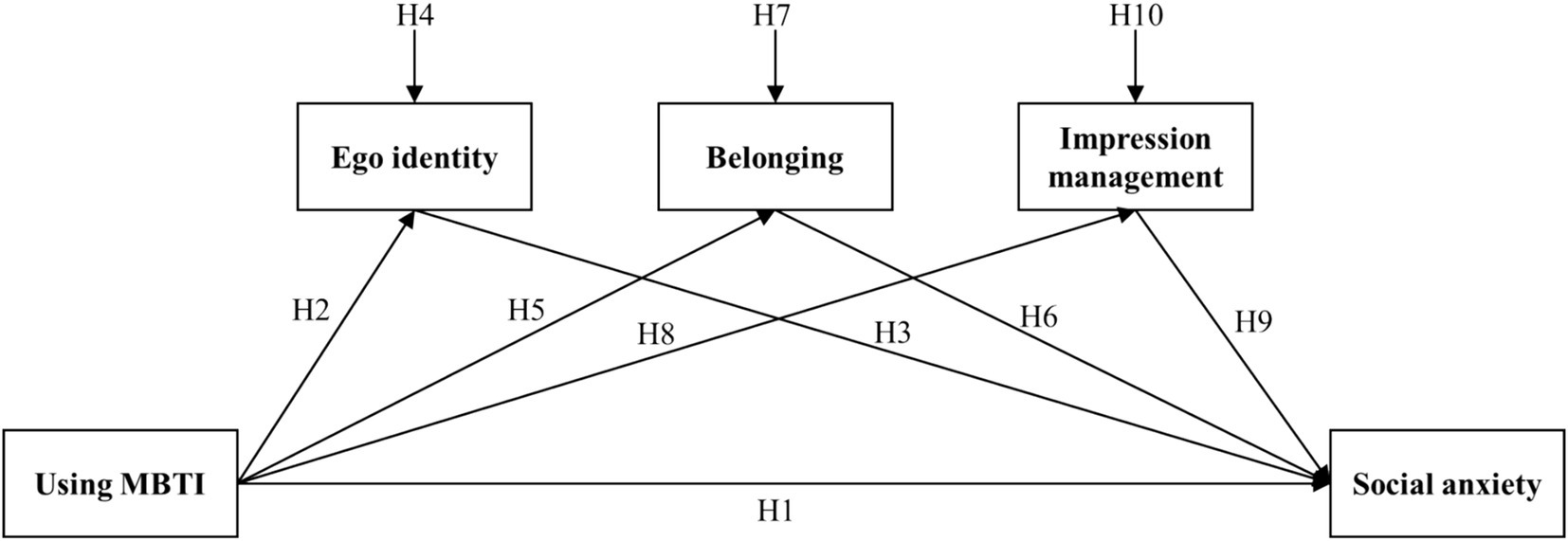
Figure 1 . Hypotheses proposed in the study.
3 Research methods and data collection
3.1 research design.
In summary, ego identity, belonging, and impression management are the research dimensions that may lead to social anxiety among youth groups during the use of MBTI. This study adopts the questionnaire survey method, which utilizes questionnaires as a tool to collect data and is widely used in current social surveys ( Moser and Kalton, 1971 ). The study employs ego identity, belonging, and impression management as mediating variables to explore the direct and indirect relationships between MBTI use and social anxiety among youth groups through questionnaire surveys. The survey targets young social media users aged 18 to 35. Since there is no unified standard for defining the age range of youth groups, and according to the World Health Organization’s definition of youth aged 18–44, this study selects participants aged 18–35 based on China’s national conditions and previous research on youth groups ( Liu et al., 2022 ).
Participants in the study were mainly recruited through the dissemination of information on social media platforms widely used in China, such as WeChat, Weibo, QQ, Xiaohongshu, Douban, etc. After eliminating invalid questionnaires with obvious indications of minimal MBTI use, short response times, and low answer discrimination, a total of 469 valid questionnaires were obtained, with an effective rate of 84.01%. The sample mainly covers relatively economically developed regions in China, such as Shanghai, Guangdong, and Zhejiang, and includes 247 males (52.7%) and 222 females (47.3%). Their age groups are 18–25 years old (29.2%), 26–30 years old (43.7%), and 31–35 years old (27.1%). The study also investigated their educational background. Specific demographic variables are shown in Table 1 .
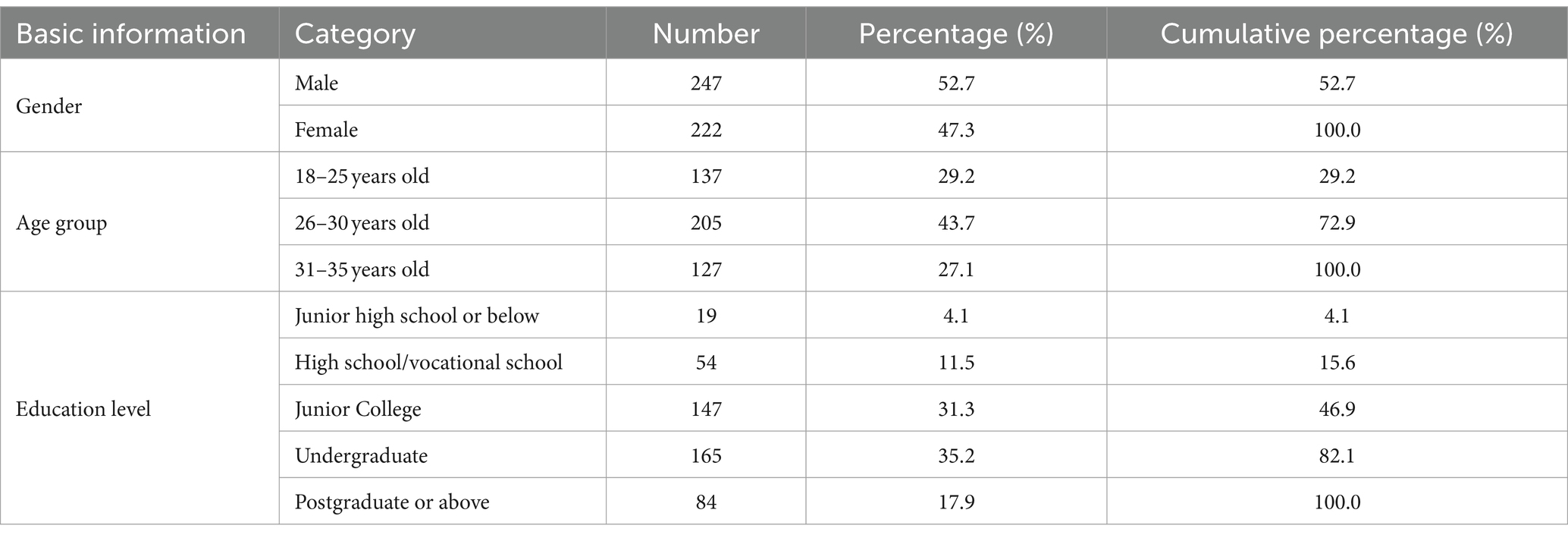
Table 1 . Basic information of participants.
3.2 Measurement scales
3.2.1 mbti usage measurement.
We adopted the Contagion-Behavioral Response (CBR) scale to measure the usage of MBTI among Chinese youth groups. The CBR scale measures reporting behaviors related to reading and basic application of MBTI information ( Lopez et al., 2021 ), without covering a deeper understanding of MBTI information. Therefore, this study modified some items to measure individuals’ understanding of MBTI and the frequency of usage behaviors such as liking, following, and sharing, to better understand participants’ MBTI usage. Participants were required to indicate their level of agreement with each item on a five-point Likert Scale (1 = Never, 5 = Always), and the degree of MBTI usage was assessed by averaging all scores.
3.2.2 Ego identity measurement
Adolescence is a critical juncture for establishing cognition of ego identity and social identity. Currently, MBTI has become a widely influential personality analysis model. Although its reliability and validity are questioned in academic circles, as a new form of social label, it has a significant impact on adolescents’ social ego identity, further affecting social anxiety among youth. Serafini and Adams (2002) constructed an identity scale based on Loevinger (1957) test method to measure the five identity functions proposed by Adams and Marshall (1996) , and verified the validity of the scale from internal consistency, structural validity, and external functionality. We adopted this scale in this study to explore whether participants’ self-cognition changed after using MBTI (1 = Never, 5 = Always).
3.2.3 Belonging measurement
This study references the social connection scale and social assurance scale developed by Lee and Robbins (1995) based on self-psychology theory. These two scales can measure the social belonging of youth groups in MBTI usage from different dimensions such as psychological distance and group consciousness (1 = Strongly agree, 6 = Strongly disagree). The reliability estimates of the scales are 0.91 and 0.82, respectively, indicating good reliability.
3.2.4 Impression management measurement
In the process of labeling MBTI among youth groups, basic social needs often stimulate their motivation for impression management. The two-component model of impression management suggests that impression management is a two-stage process, including impression management motivation and impression construction. Impression management motivation is defined as “the extent to which people are motivated to control how others perceive them” ( Leary and Kowalski, 1990 ). In the process of using MBTI labels for social interaction, youth groups are likely to experience social anxiety based on the need for impression management. This study drew on the self-control measurement scale developed by Tangney et al. (2004) , which improves upon the self-control questionnaire proposed by Brandon et al. (1990) by incorporating individual difference factors into the measurement of self-control, demonstrating good reliability. Participants in this study were required to make internal judgments and choices related to impression management dimensions based on their true thoughts after using MBTI (1 = Strongly disagree, 5 = Strongly agree).
3.2.5 Social anxiety scale
The use of MBTI among youth groups is influenced by social anxiety to some extent. Different personality classifications can easily lead to phenomena such as social group division and individual isolation. Schlenker believes that people’s concern about receiving unfavorable evaluations from others is related to many social psychological phenomena, including conformity, prosocial behavior, self-presentation, self-serving attribution, social anxiety, self-handicapping, attitude change, etc. ( Schlenker and Leary, 1982 ). The Fear of Negative Evaluation (FNE) scale proposed by Watson and Friend (1969) is the most commonly used measure to determine the degree of fear of receiving negative evaluations from others, with social anxiety being an important indicator. However, the utility of this scale is limited by its length. Therefore, Mark R proposed a short version of the scale ( Leary, 1983 ), which has only 12 items but is highly correlated with the original scale. This study borrows from this scale and modifies the items according to the participants’ context, measuring the degree of social anxiety in MBTI usage from the perspective of the influence of others’ evaluations on self-evaluation (1 = Strongly disagree, 5 = Strongly agree).
3.3 Data analysis
The data organization tool for this study was Excel, while the data analysis tools were SPSS 27.0 and the Process 4.1 macro plugin developed by Hayes (2013) . Due to inconsistencies in the scales of individual variables, the data were first standardized using z-score normalization in SPSS 27.0 before analyzing. Subsequently, all items underwent reliability and validity tests. Based on the standardized item data, the average value of different variables were calculated, followed by the computation of correlations between variables. Finally, model 4 in the Process 4.1 macro plugin was used for parallel mediator variable analysis. For all mediator variables, the Bootstrap test was employed to further verify their mediating effects.
3.3.1 Reliability and validity tests
The items selected for the study were validated in the researcher’s papers and exhibited good reliability and validity ( Leary, 1983 ; Lee and Robbins, 1995 ; Serafini and Adams, 2002 ; Tangney et al., 2004 ; Lopez et al., 2021 ). To further examine the reliability and validity of the survey data, Cronbach’s Alpha coefficient, KMO value, and Bartlett’s Test of Sphericity were calculated using SPSS 27.0. The results showed that the Cronbach’s Alpha coefficients for all dimensions ranged from 0.867 to 0.955, all higher than the threshold of 0.7, indicating high internal consistency and ideal reliability of the scales in the questionnaire. The KMO values for all dimensions ranged from 0.888 to 0.977, all exceeding the threshold of 0.7, and the sig. Values for Bartlett’s Test of Sphericity were all 0.000, indicating high data validity. Meanwhile, due to the diverse sources of data in this study, a Harman’s single-factor analysis of variance was also conducted on the data. The results showed that the eigenvalues of 12 factors were greater than 1, and the variance explained by the first common factor was 26.23%, less than the standard value of 40%. Therefore, there was no common method bias, and the results were valid for subsequent data analysis. The results of reliability and validity analysis for each variable are shown in Table 2 .

Table 2 . Reliability and validity of each dimension of the scale.
3.3.2 Correlation analysis
To further investigate whether there were correlations between the dimensions, Pearson correlation coefficient measurements were conducted on all dimensions. As shown in Table 3 , there were significant and positive correlations between Using MBTI, Ego Identity, Belonging, Impression Management, and Social Anxiety. Among them, Ego Identity had the highest correlation with Using MBTI ( r = 0.754), followed by Belonging ( r = 0.533), Social Anxiety ( r = 0.324), and Impression Management ( r = 0.295). It is evident that all variables were positively correlated, and both Ego Identity and Belonging had r values >0.5, indicating significant correlations with Using MBTI.
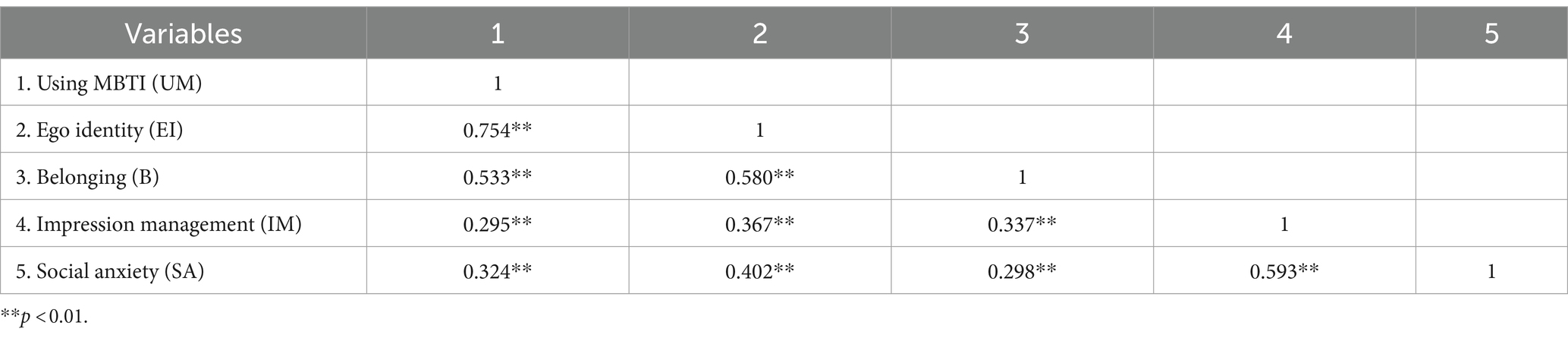
Table 3 . Correlation analysis between variables.
3.3.3 Regression analysis of the mediation model
The study used the Process macro program 4.1 developed by Hayes to perform regression analysis on the variables, selecting model 4. The regression analysis is shown in Table 4 ( Hayes, 2013 ). The results indicated that Using MBTI had a significant positive effect on Ego Identity ( β = 0.705, p < 0.001), Belonging ( β = 0.530, p < 0.001), and Impression Management ( β = 0.332, p < 0.001). Ego Identity ( β = 0.272, p < 0.01) and Impression Management ( β = 0.600, p < 0.001) had a significant positive impact on Social Anxiety. This suggests that Ego Identity and Impression Management partially mediate the relationship between Using MBTI and Social Anxiety. Therefore, hypotheses H2, H3, H5, H8, and H9 were supported, while H4 and H10 received initial support and required further testing. Hypotheses H1, H6, and H7 were not supported.
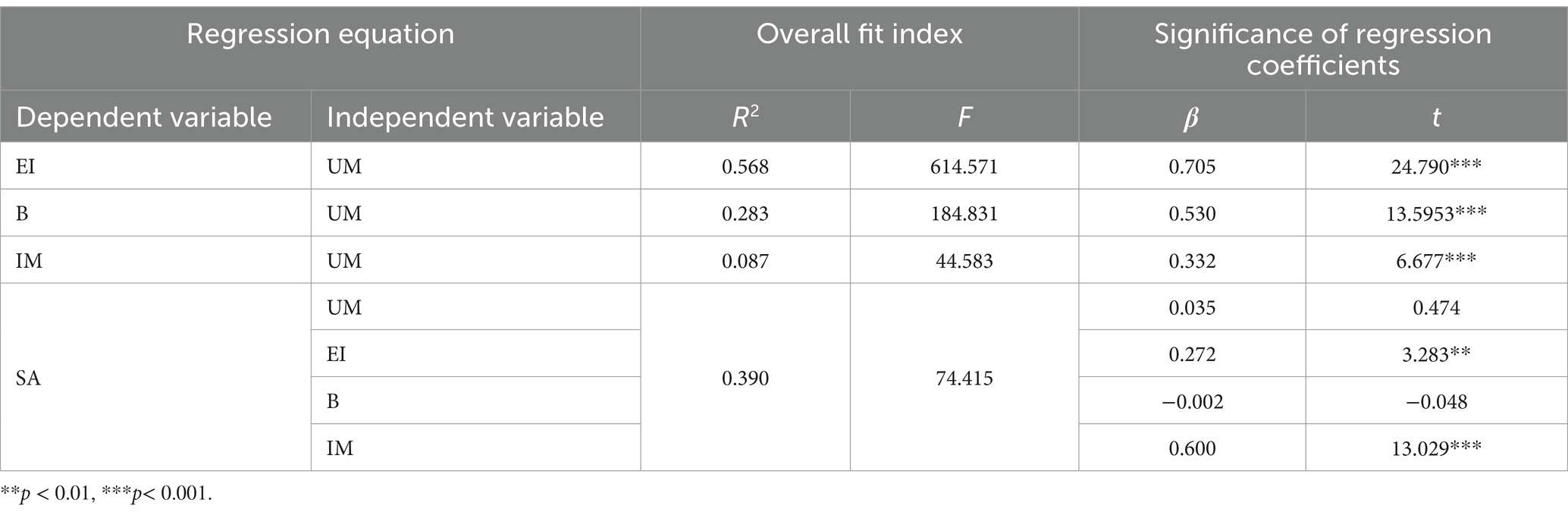
Table 4 . Regression analysis of variable relationships.
To further estimate the performance indicators of the model, a Bootstrap test was conducted on the mediator variables, as shown in Table 5 . In the process where Using MBTI affects Social Anxiety through Ego Identity, Belonging, and Impression Management, both Ego Identity and Impression Management did not include 0 in the Bootstrap 95% confidence interval, indicating significant mediating effects of these two variables. Belonging included 0, further confirming that hypothesis H7 was not supported. Therefore, hypotheses H4 and H10 were supported. According to the research data, the mediation model effect is shown in Figure 2 .

Table 5 . Analysis of mediation effects.
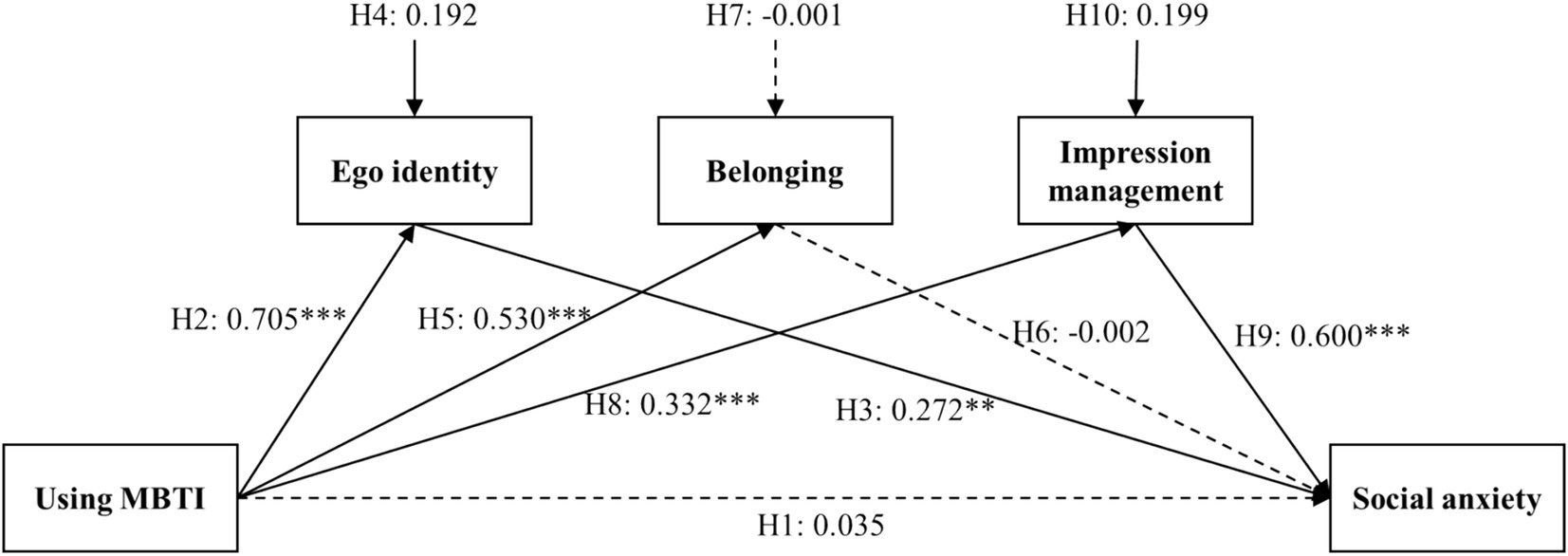
Figure 2 . Model diagram of the association between using MBTI and social anxiety. Solid lines indicate supported hypotheses, and dashed lines indicate unsupported hypotheses. ** p < 0.01, *** p < 0.001.
4 Discussion and conclusion
4.1 analysis of research findings, 4.1.1 the relationship between using mbti and social anxiety.
The correlation between using MBTI as their social label and social anxiety in Chinese youth is weak, and the results from the Process 4.1 macro plugin are also insignificant. This outcome roughly confirms that the relationship between the two is not close, and it is clear that using MBTI as a social label does not lead to social anxiety. In previous research, scholars measured whether using MBTI by adolescent groups would bring mental health issues, and their results showed that it could enhance the level of subjective well-being, thereby reducing anxiety and depression ( Hua and Zhou, 2023 ), but they did not discuss it in social interactions. The results of this study further indicate that using MBTI as a social label may not bring any harm. The possible reason for this outcome is that during the long-term use by Chinese youth, MBTI has primarily served to further strengthen self-cognition, without excessive focus on whether interactions with others would bring adverse consequences. This is also an important reason why social anxiety is difficult to arise. In other words, simply acquiring and using a social label may not impact social anxiety.
4.1.2 Differences in the mediating effects of ego identity, belonging, and impression management
Due to the insignificance of the direct effect, the mediating effects influencing social anxiety become crucial. Next, we discuss several mediating effects proposed in the research hypothesis.
4.1.2.1 Significant mediating effect of ego identity
Previous research clearly indicates that using MBTI will have a positive impact on ego identity, and immature self-cognition is more likely to trigger social anxiety ( Mazalin and Moore, 2004 ; Ruth, 2013 ; Hua and Zhou, 2023 ). Does this mean that acquiring ego identity through MBTI will alleviate social anxiety to some extent? The survey data suggests that this may not be the case. The emergence of MBTI has gradually clarified ego identity. The past self may have been relatively chaotic, with greater interpretive space, where different viewpoints would be seen as individual differences rather than linked to personality types. However, after ego identity is clarified, it may not immediately bring excessive harm, but long-term interactions could potentially disrupt this relatively stable state. Chinese youth primarily use MBTI as a social label on social media, engaging in frequent and prolonged interactions with others. It is highly likely that some personality traits proposed by MBTI cannot fully explain individual behavior, even leading to doubts about MBTI. Ultimately, MBTI still has limitations in reliability and usefulness ( Boyle, 1995 ). Individuals in this state may experience a relatively contradictory state: Can MBTI as a social label truly help me understand myself and others? Is my interaction with others based on MBTI still meaningful? Or, having roughly figured out this person through MBTI, will they not have a good outcome with me? Is it necessary to socialize with them? Is judging a person through MBTI too one-sided? The emergence of these questions will influence the generation of social anxiety to some extent.
4.1.2.2 Significant mediating effect of impression management
From the perspective of interpersonal relationships, impression management is more about self-control. When self-control weakens, it is unsurprising that social anxiety arises ( Uziel, 2014 ). The data clearly shows that impression management has a relatively large impact on social anxiety, and its mediating effect is the strongest. This is primarily because, while MBTI serves as a social label, it also becomes a shackle in interpersonal interactions. People need to self-control according to the impressions that most people have of this personality label. Even if these impressions are stereotypes, prejudices, and discriminations, they have to do so ( Gilbert et al., 1998 ; Fiske et al., 2009 ). This is because those who hold these impressions do not think there is anything wrong with them, as they avoid cognitive dissonance by constantly denying new cognition ( Aronson, 1969 ; Jonas et al., 2001 ). However, from a personal perspective, these impressions will exert greater pressure on individuals. They may not be like what is described in MBTI but are forced to become someone with that personality trait. Individuals with stronger self-cognition may think, “This is not the real me, but I have to abide by these so-called ‘rules’ when socializing with others.” In this case, the increasing social pressure will further lead to social anxiety. That is, in social interactions with others, the mask needs to be present all the time. Once self-control weakens and the mask breaks, it will lead to others’ dislike. It is out of this concern that people have to pretend and control themselves, which also reflects the impact of impression management on social anxiety.
4.1.2.3 Insignificant mediating effect of belonging
In traditional Chinese beliefs, social interaction is a process of gaining energy. It helps individuals seek solace from groups and feel happiness and joy. That is, people desire to socialize with others and acquire a sense of belonging ( Baumeister and Leary, 1995 ). By using MBTI as a social label, people can quickly find their unique groups, thereby alleviating the possibility of social anxiety to some extent. The research hypothesis, when proposing this question, focused on people’s desire to maintain social interactions and the subsequent induction of social anxiety under a strong sense of belonging. However, the research hypothesis may have neglected the factors of accelerated urbanization and increased personnel mobility in China. A large number of Chinese youth, due to work, study, life, and other needs, have to leave familiar societies and enter unfamiliar ones. This has also led to relatively weak connections between people. Using MBTI can establish a sense of belonging, thereby escaping the state of being a relatively isolated individual. This also makes people more urgent to find suitable organizations or groups than to worry about social anxiety. Organizations formed based on personality labels also achieve a sharing of responsibilities to some extent. When individuals feel disliked, even foolish or boring, people are likely to attribute it to this personality label group rather than criticize the individual. In other words, by finding a group that provides a sense of belonging, individual responsibilities are shared by group members, leading to the “Diffusion of Responsibility” phenomenon ( Darley and Latané, 1968 ). The above analysis clarifies the important reason for the insignificant mediating effect of belonging.
4.2 Research value
4.2.1 theoretical value.
This research considers MBTI as a social label and explores its impact on social anxiety and the underlying mechanisms. Past research on MBTI at the social level mostly remained at theoretical speculation, often lacking empirical data support ( Wang et al., 2024 ; Zhang, 2024 ). This study further explores the association mechanisms between using MBTI in social interactions and ego identity, belonging, impression management, and social anxiety from an empirical perspective. However, does frequent use of MBTI as a social label bring harm? To further investigate this issue, this research explores social anxiety as the dependent variable. This is primarily due to the increasing prevalence of social anxiety among Chinese youth ( Xin et al., 2022 ). Will using MBTI become a new factor contributing to social anxiety among Chinese youth? Although the results do not support the hypothesis that directly using MBTI as a social label impacts social anxiety, they confirm that the mediating effects of ego identity and impression management will influence social anxiety. This research also contributes to viewing MBTI from different perspectives and provides a theoretical foundation for research on the social mental health of youth groups. Furthermore, the proposition of the impact of MBTI-based social interactions on social anxiety further confirms the strong external validity of different variables and dimensions.
4.2.2 Practical value
Since MBTI as a social label may exacerbate or alleviate social anxiety in specific contexts, this helps mental health professionals and social media platforms formulate more precise intervention measures. Mental health professionals can provide more personalized psychological guidance to youth groups, thereby guiding them to correctly understand and use MBTI and reduce unnecessary psychological pressure. Social media platforms can optimize content recommendation and user interaction mechanisms to reduce social anxiety caused by the abuse of social labels.
4.3 Limitations and research prospects
Although this research has achieved certain outcomes, there are also some limitations. First, the diversity and representativeness of the sample may be insufficient. Future research should strive to expand the sample scope to enhance universality. Second, variable control may not be sufficient. The labeled social interaction mode of MBTI is regulated by many factors. In the future, more factors that may affect social anxiety should be introduced for deeper research. Looking ahead, a combination of multiple methods, cross-cultural comparative studies, and long-term tracking studies will help more comprehensively reveal the impact of using MBTI on social anxiety.
Data availability statement
The datasets presented in this study can be found in online repositories. The names of the repository/repositories and accession number(s) can be found in the article/ Supplementary material .
Ethics statement
Written informed consent was obtained from the individual(s) for the publication of any potentially identifiable images or data included in this article.
Author contributions
WW: Writing – original draft, Writing – review & editing, Conceptualization, Data curation, Validation. WH: Funding acquisition, Investigation, Writing – review & editing. GZ: Data curation, Validation, Writing – review & editing. WD: Date curation, Methodology, Conceptualization, Funding acquisition, Writing – review & editing.
The author(s) declare that no financial support was received for the research, authorship, and/or publication of this article.
Acknowledgments
We are very grateful to Professor Weijia Deng of Shanghai International Studies University for her guidance on this manuscript.
Conflict of interest
The authors declare that the research was conducted in the absence of any commercial or financial relationships that could be construed as a potential conflict of interest.
Publisher’s note
All claims expressed in this article are solely those of the authors and do not necessarily represent those of their affiliated organizations, or those of the publisher, the editors and the reviewers. Any product that may be evaluated in this article, or claim that may be made by its manufacturer, is not guaranteed or endorsed by the publisher.
Supplementary material
The Supplementary material for this article can be found online at: https://www.frontiersin.org/articles/10.3389/fpsyg.2024.1419492/full#supplementary-material
Adams, G. R., and Marshall, S. K. (1996). A developmental social psychology of identity: understanding the person-in-context. J. Adolesc. 19, 429–442. doi: 10.1006/jado.1996.0041
PubMed Abstract | Crossref Full Text | Google Scholar
Anant, S. S. (1966). Need to belong. Canadas mental. Health 14, 21–27.
Google Scholar
Aronson, E. (1969). “The theory of cognitive dissonance: a current perspective” in Advances in experimental social psychology . Ed. L. Berkowitz, (Academic Press), 1–34.
Baumeister, R. F., and Leary, M. R. (1995). The need to belong: desire for interpersonal attachments as a fundamental human motivation. Psychological bulletin , 117, 497–529.
Baumeister, R. F., and Tice, D. M. (1990). Point-counterpoints: anxiety and social exclusion. J. Soc. Clin. Psychol. 9, 165–195. doi: 10.1521/jscp.1990.9.2.165
Crossref Full Text | Google Scholar
Becker, H. (1963). Outsiders: studies in the sociology of deviance (New York) 1982 . Berkeley: Art Worlds.
Bernburg, J. G. (2009). Labeling theory. Handbook Crime Deviance , eds. M. D. Krohn, A. J. Lizotte, and G. P. Hall. (New York, NY: Springer New York), 187–207.
BlaCkhart, G. C., Williamson, J., and Nelson, L. (2015). Social anxiety in relation to self-control depletion following social interactions. J. Soc. Clin. Psychol. 34, 747–773. doi: 10.1521/jscp.2015.34.9.747
Bodenhausen, G. V., Macrae, C. N., and Hugenberg, K. (2003). “Activating and inhibiting social identities: implications for perceiving the self and others” in Foundations of social cognition: A festschrift in honor of Robert S. Wyer, Jr , Mahwah, NJ, US: Lawrence Erlbaum Associates Publishers. 131–154.
Bourne, E. (1978). The state of research on ego identity: a review and appraisal: part I. J. Youth Adolesc. 7, 223–251. doi: 10.1007/BF01537976
Boyle, G. J. (1995). Myers-Briggs type indicator (MBTI): some psychometric limitations. Aust. Psychol. 30, 71–74. doi: 10.1111/j.1742-9544.1995.tb01750.x
Brandon, J. E., Oescher, J., and Loftin, J. M. (1990). The Self-Control Questionnaire: An assessment. Health Values: Health Behavior, Education & Promotion . 14, 3–9.
Brown, J. (1998). The self (1st ed.). New York, NY, US: Psychology Press.
Bruner, J. S. (1957). On perceptual readiness. Psychol. Rev. 64, 123–152. doi: 10.1037/h0043805
Chi, L., and Xin, Z. (2020). Cross-temporal changes of social psychology in different populations. Psychology 8, 95–103. doi: 10.16842/j.cnki.issn2095-5588.2020.02.004
Claridge, T. (2020). Identity and belonging. Acad. Manag. Rev. 23:242. doi: 10.5281/zenodo.8053300
Counsell, A., Furtado, M., Iorio, C., Anand, L., Canzonieri, A., Fine, A., et al. (2017). Intolerance of uncertainty, social anxiety, and generalized anxiety: differences by diagnosis and symptoms. Psychiatry Res. 252, 63–69. doi: 10.1016/j.psychres.2017.02.046
Darley, J. M., and Latané, B. (1968). Bystander intervention in emergencies: diffusion of responsibility. J. Pers. Soc. Psychol. 8, 377–383. doi: 10.1037/h0025589
Ellemers, N., Spears, R., and Doosje, B. (2002). Self and social identity. Annu. Rev. Psychol. 53, 161–186. doi: 10.1146/annurev.psych.53.100901.135228
Erikson, E. H. (1956). The problem of ego identity. J. Am. Psychoanal. Assoc. 4, 56–121. doi: 10.1177/000306515600400104
Finkel, E. J., Campbell, W. K., Brunell, A. B., Dalton, A. N., Scarbeck, S. J., and Chartrand, T. L. (2006). High-maintenance interaction: inefficient social coordination impairs self-regulation. J. Pers. Soc. Psychol. 91, 456–475. doi: 10.1037/0022-3514.91.3.456
Fiske, S. T., Rosenblum, K. E., and Travis, T.-M. C. (2009). Social beings: a core motives approach to social psychology . New York: Wiley.
Furnham, A., and Stringfield, P. (1993). Personality and occupational behavior: Myers-Briggs type indicator correlates of managerial practices in two cultures. Hum. Relat. 46, 827–848. doi: 10.1177/001872679304600703
Galinsky, A. D., Hugenberg, K., Groom, C., and Bodenhausen, G. V. (2003). “The reappropriation of stigmatizing labels: implications for social identity” in Identity issues in groups . Ed. J. Polzer, (Emerald Group Publishing Limited), 221–256.
Gilbert, D. T., Fiske, S. T., and Lindzey, G. (1998). The handbook of social psychology : Vols. 1–2, 4th ed. New York, US, McGraw-Hill: Oxford University Press.
Hayes, A. F. (2013). Introduction to mediation, moderation, and conditional process analysis: a regression-based approach . New York, NY, US: Guilford Press.
Hua, J., and Zhou, Y.-X. (2023). Personality assessment usage and mental health among Chinese adolescents: a sequential mediation model of the Barnum effect and ego identity. Front. Psychol. 14:1097068. doi: 10.3389/fpsyg.2023.1097068
Jonas, E., Schulz-Hardt, S., Frey, D., and Thelen, N. (2001). Confirmation bias in sequential information search after preliminary decisions: an expansion of dissonance theoretical research on selective exposure to information. J. Pers. Soc. Psychol. 80, 557–571. doi: 10.1037/0022-3514.80.4.557
Jones, E. E., and Pittman, T. S. (1982). Toward a general theory of strategic self-presentation. Psychol. Perspect. Self 1, 231–262.
Jung, C. G. (1971). Collected works of C.G. Jung, volume 6: psychological types : Princeton, New Jersey, US: Princeton University Press.
Kashdan, T. B., Weeks, J. W., and Savostyanova, A. A. (2011). Whether, how, and when social anxiety shapes positive experiences and events: a self-regulatory framework and treatment implications. Clin. Psychol. Rev. 31, 786–799. doi: 10.1016/j.cpr.2011.03.012
Leary, M. R. (1983). A brief version of the fear of negative evaluation scale. Personal. Soc. Psychol. Bull. 9, 371–375. doi: 10.1177/0146167283093007
Leary, M. R. (1995). Self-presentation: impression management and interpersonal behavior . Madison, WI, US: Brown & Benchmark Publishers.
Leary, M. R., and Kowalski, R. M. (1990). Impression management: a literature review and two-component model. Psychol. Bull. 107, 34–47. doi: 10.1037/0033-2909.107.1.34
Lee, R. M., and Robbins, S. B. (1995). Measuring belongingness: the social connectedness and the social assurance scales. J. Couns. Psychol. 42, 232–241. doi: 10.1037/0022-0167.42.2.232
Liu, Y., Zhu, J., and He, J. (2022). Can selfies trigger social anxiety? A study on the relationship between social media selfie behavior and social anxiety in Chinese youth group. Front. Psychol. 13:1016538. doi: 10.3389/fpsyg.2022.1016538
Loevinger, J. (1957). Objective tests as instruments of psychological theory. Psychol. Rep. 3, 635–694. doi: 10.2466/pr0.1957.3.3.635
Lopez, K. R. B., Gaticales, N. P., Provido, A. V. C., Santelices, S. M. B., and Arcinas, M. M. (2021). Social contagion of astrology in the social media amid COVID-19 pandemic. Int. J. Multidisciplinary 2, 349–363. doi: 10.11594/ijmaber.02.04.08
Mahar, A. L., Cobigo, V., and Stuart, H. (2013). Conceptualizing belonging. Disabil. Rehabil. 35, 1026–1032. doi: 10.3109/09638288.2012.717584
Marcia, J. E. (1966). Development and validation of ego-identity status. J. Pers. Soc. Psychol. 3, 551–558. doi: 10.1037/h0023281
Mazalin, D., and Moore, S. (2004). Internet use, identity development and social anxiety among young adults. Behav. Chang. 21, 90–102. doi: 10.1375/bech.21.2.90.55425
McCaulley, M. H., and Martin, C. R. (1995). Career assessment and the Myers-Briggs type indicator. J. Career Assess. 3, 219–239. doi: 10.1177/106907279500300208
Morrison, A. S., and Heimberg, R. G. (2013). Social anxiety and social anxiety disorder. Annu. Rev. Clin. Psychol. 9, 249–274. doi: 10.1146/annurev-clinpsy-050212-185631
Moser, C. A., and Kalton, G. (1971). Survey methods in social investigation (1st ed.). London, UK: Routledge.
Pestana, J. V., and Codina, N. (2019). Being conscious of one’s own heroism: an empirical approach to analyzing the leadership potential of future CEOs. Front. Psychol. 9:2787. doi: 10.3389/fpsyg.2018.02787
Pestana, J. V., and Codina, N. (2020). Collective and individual sources of women’s creativity: heroism and psychological types involved in enhancing the talent of emerging leaders. Sustain. For. 12:4414. doi: 10.3390/su12114414
Quenk, N. L. (2009). Essentials of Myers-Briggs type indicator assessment , New York, NY, US: John Wiley & Sons.
Ruth, C. E. (2013). Crisis as Opportunity: Personality Constructs and Erikson Identity Development. Graduate Theses, Dissertations, and Problem Reports . Available at: https://researchrepository.wvu.edu/etd/3640
Schlenker, B. R., and Leary, M. R. (1982). Social anxiety and self-presentation: a conceptualization model. Psychol. Bull. 92, 641–669. doi: 10.1037/0033-2909.92.3.641
Serafini, T. E., and Adams, G. R. (2002). Functions of identity: scale construction and validation. Identity 2, 361–389. doi: 10.1207/S1532706XID0204_05
Stein, M. B., and Stein, D. J. (2008). Social anxiety disorder. Lancet 371, 1115–1125. doi: 10.1016/S0140-6736(08)60488-2
Suchanek, F.M., Vojnovic, M., and Gunawardena, D. (2008). "Social tags: meaning and suggestions", in: Proceedings of the 17th ACM conference on Information and knowledge management), 223–232.
Tangney, J. P., Baumeister, R. F., and Boone, A. L. (2004). High self-control predicts good adjustment, less pathology, better grades, and interpersonal success. Journal of personality , 72, 271–324. doi: 10.1111/j.0022-3506.2004.00263.x
Tedeschi, J. T. (2013). Impression management theory and social psychological research . New York, NY, US: Academic Press.
Turnley, W. H., and Bolino, M. C. (2001). Achieving desired images while avoiding undesired images: exploring the role of self-monitoring in impression management. J. Appl. Psychol. 86, 351–360. doi: 10.1037/0021-9010.86.2.351
Uziel, L. (2010). Rethinking social desirability scales: from impression management to interpersonally oriented self-control. Perspect. Psychol. Sci. 5, 243–262. doi: 10.1177/1745691610369465
Uziel, L. (2014). Impression management (“lie”) scales are associated with interpersonally oriented self-control, not other-deception. J. Pers. 82, 200–212. doi: 10.1111/jopy.12045
Wang, C., Gao, Y., and Xie, Y. (2024). "Analyzing the dissemination of the Myers-Briggs type Indicator (MBTI) in China: a case study of Weibo and Xiaohongshu texts", in: Proceedings of the 4th International Conference on Public Management and Intelligent Society, PMIS 2024, 15–17 March 2024, Changsha, China
Watson, D., and Friend, R. (1969). Measurement of social-evaluative anxiety. J. Consult. Clin. Psychol. 33, 448–457. doi: 10.1037/h0027806
Wu, L., Zhou, Z., and Chen, B. (2011). "The application of MBTI personality type theory in the Bank management", in: 2011 6th IEEE Joint International Information Technology and Artificial Intelligence Conference, Chongqing, China, 455–458.
Xin, S., Liang, X., Sheng, L., and Zhao, Z. (2021). Changes of teachers’ subjective well-being in mainland China (2002~ 2019): the perspective of cross-temporal meta-analysis. Acta Psychol. Sin. 53:875. doi: 10.3724/SP.J.1041.2021.00875
Xin, S., Peng, H., and Sheng, L. (2022). Changes of social anxiety in Chinese adolescents during 2002∼ 2020: an increasing trend and its relationship with social change. Child Youth Serv. Rev. 142:106614. doi: 10.1016/j.childyouth.2022.106614
Yang, F., Li, M., and Han, Y. (2023). Whether and how will using social media induce social anxiety? The correlational and causal evidence from Chinese society. Front. Psychol. 14:1217415. doi: 10.3389/fpsyg.2023.1217415
Zhang, Y. (2024). “Impression management and interpersonal communication in the internet age: exploring the new dynamics with MBTI personality testing as an example” in Addressing global challenges-exploring socio-cultural dynamics and sustainable solutions in a changing world (London, UK: Routledge), 856–862.
Keywords: MBTI, social labels, personality types, social anxiety, Chinese youth
Citation: Wu W, Hao W, Zeng G and Du W (2024) From personality types to social labels: the impact of using MBTI on social anxiety among Chinese youth. Front. Psychol . 15:1419492. doi: 10.3389/fpsyg.2024.1419492
Received: 23 April 2024; Accepted: 19 August 2024; Published: 04 September 2024.
Reviewed by:
Copyright © 2024 Wu, Hao, Zeng and Du. This is an open-access article distributed under the terms of the Creative Commons Attribution License (CC BY) . The use, distribution or reproduction in other forums is permitted, provided the original author(s) and the copyright owner(s) are credited and that the original publication in this journal is cited, in accordance with accepted academic practice. No use, distribution or reproduction is permitted which does not comply with these terms.
*Correspondence: Weiyi Du, [email protected]
Disclaimer: All claims expressed in this article are solely those of the authors and do not necessarily represent those of their affiliated organizations, or those of the publisher, the editors and the reviewers. Any product that may be evaluated in this article or claim that may be made by its manufacturer is not guaranteed or endorsed by the publisher.

COMMENTS
Definition: Research Paper is a written document that presents the author's original research, analysis, and interpretation of a specific topic or issue. It is typically based on Empirical Evidence, and may involve qualitative or quantitative research methods, or a combination of both. The purpose of a research paper is to contribute new ...
Style. The prose style of a term paper should be formal, clear, concise, and direct. Don't try to sound "academic" or "scientific.". Just present solid research in a straightforward manner. Use the documentation style prescribed in your assignment or the one preferred by the discipline you're writing for.
Experimental research paper. This type of research paper basically describes a particular experiment in detail. It is common in fields like: biology. chemistry. physics. Experiments are aimed to explain a certain outcome or phenomenon with certain actions. You need to describe your experiment with supporting data and then analyze it sufficiently.
Step 1: Find a topic and review the literature. As we mentioned earlier, in a research paper, you, as the researcher, will try to answer a question.More specifically, that's called a research question, and it sets the direction of your entire paper. What's important to understand though is that you'll need to answer that research question with the help of high-quality sources - for ...
Choose a research paper topic. Conduct preliminary research. Develop a thesis statement. Create a research paper outline. Write a first draft of the research paper. Write the introduction. Write a compelling body of text. Write the conclusion. The second draft.
The main guidelines for formatting a paper in APA Style are as follows: Use a standard font like 12 pt Times New Roman or 11 pt Arial. Set 1 inch page margins. Apply double line spacing. If submitting for publication, insert a APA running head on every page. Indent every new paragraph ½ inch.
A research paper is a type of academic writing that provides an in-depth analysis, evaluation, or interpretation of a single topic, based on empirical evidence. Research papers are similar to analytical essays, except that research papers emphasize the use of statistical data and preexisting research, along with a strict code for citations.
Then, writing the paper and getting it ready for submission may take me 3 to 6 months. I like separating the writing into three phases. The results and the methods go first, as this is where I write what was done and how, and what the outcomes were. In a second phase, I tackle the introduction and refine the results section with input from my ...
Checklist: Writing a Great Research Paper. Published on October 16, 2022 by Shona McCombes.Revised on November 29, 2022. A research paper is an extended piece of writing based on in-depth independent research. It may involve conducting empirical research or analyzing primary and secondary sources.. Writing a good research paper requires you to demonstrate a strong knowledge of your topic and ...
How to start your research paper [step-by-step guide] All research papers have pretty much the same structure. If you can write one type of research paper, you can write another. Learn the steps to start and complete your research paper in our guide.
Once you've got a firm grip on your subject and the source material available to you, formulate a detailed outline, including your thesis statement and how you are going to support it. The structure of your paper will depend on the subject type—ask a tutor for a research paper outline example if you're unsure. Get Writing!
This interactive resource from Baylor University creates a suggested writing schedule based on how much time a student has to work on the assignment. "Research Paper Planner" (UCLA) UCLA's library offers this step-by-step guide to the research paper writing process, which also includes a suggested planning calendar.
A research proposal comes before a research paper. It is a detailed document that outlines an intended area of exploration. It includes the research topic, methodology, timeline, sources, and potential conclusions. Research proposals are often required when seeking approval to conduct research. A research paper is a summary of research findings ...
General formatting rules are as follows: Do not put page breaks in between the introduction, method, results, and discussion sections. The title page, abstract, references, table (s), and figure (s) should be on their own pages. The entire paper should be written in the past tense, in a 12-point font, double-spaced, and with one-inch margins ...
Below is a step-by-step guide to starting and completing your research paper. Organize your papers in one place. Try Paperpile. No credit card needed. Get 30 days free. 1. Choose your topic. Choose a topic that interests you. Writing your research paper will be so much more pleasant with a topic that you actually want to know more about.
Media Files: APA Sample Student Paper , APA Sample Professional Paper This resource is enhanced by Acrobat PDF files. Download the free Acrobat Reader. Note: The APA Publication Manual, 7 th Edition specifies different formatting conventions for student and professional papers (i.e., papers written for credit in a course and papers intended for scholarly publication).
Definition paper. Experimental paper. Interpretative paper. Survey paper. Let us understand each type of research paper in detail. 1. Analytical Research Paper. While writing an analytical paper, the student asks a question, collects relevant data from a variety of sources, and analyzes it. The findings, viewpoints, and conclusions presented in ...
Table of contents. Step 1: Introduce your topic. Step 2: Describe the background. Step 3: Establish your research problem. Step 4: Specify your objective (s) Step 5: Map out your paper. Research paper introduction examples. Frequently asked questions about the research paper introduction.
The pages in this section cover the following topic areas related to the process of writing a research paper: Genre - This section will provide an overview for understanding the difference between an analytical and argumentative research paper. Choosing a Topic - This section will guide the student through the process of choosing topics ...
Writing a Research Paper. This page lists some of the stages involved in writing a library-based research paper. Although this list suggests that there is a simple, linear process to writing such a paper, the actual process of writing a research paper is often a messy and recursive one, so please use this outline as a flexible guide.
This type of paper hopes to persuade its reader to adopt the view presented. Example: a paper that argues the merits of early exposure to reading for children would be an argumentative essay. Analytical. An analytical research paper states the topic that the writer will be exploring, usually in the form of a question, initially taking a neutral ...
To format a paper in APA Style, writers can typically use the default settings and automatic formatting tools of their word-processing program or make only minor adjustments. The guidelines for paper format apply to both student assignments and manuscripts being submitted for publication to a journal. If you are using APA Style to create ...
A research method is your strategy used to implement that plan. These ideas are closely related but research design ensures you will answer your research question more effectively. Which research method should I choose? It depends on your research and the data you are trying to collect. Common research methods used are:
A research paper outline is a useful tool to aid in the writing process, providing a structure to follow with all information to be included in the paper clearly organized. A quality outline can make writing your research paper more efficient by helping to: Organize your thoughts; Understand the flow of information and how ideas are related
Aspect-based sentiment analysis (ABSA) is a fine-grained type of sentiment analysis that identifies aspects and their associated opinions from a given text. With the surge of digital opinionated text data, ABSA gained increasing popularity for its ability to mine more detailed and targeted insights. Many review papers on ABSA subtasks and solution methodologies exist, however, few focus on ...
School of Journalism and Communication, Shanghai International Studies University, Shanghai, China; Introduction: As the Myers-Briggs Type Indicator (MBTI) gains popularity among Chinese young people, it has undergone a gradual transition from being perceived as a personality assessment tool to being regarded as a social label. The objective of this study was to ascertain whether the use of ...